Musical Horizons: Spotify the Secret Nudger
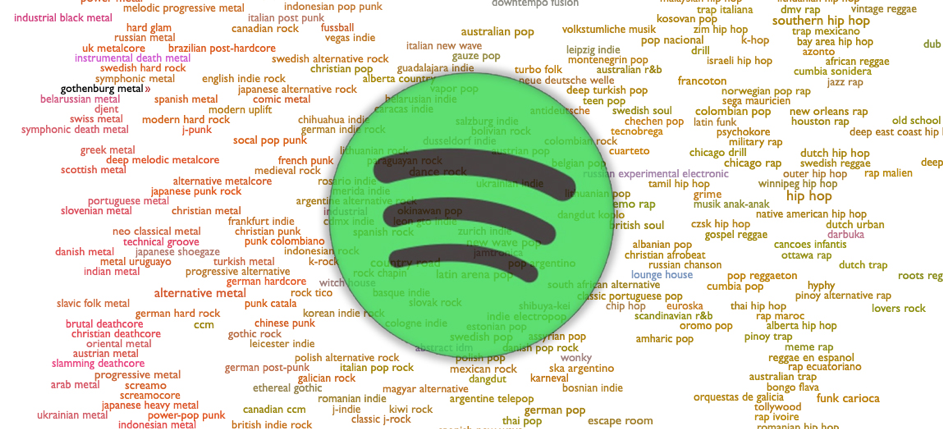
Introduction
Human interaction with music has changed dramatically since the paradigm shift from traditional music to streaming (Herrfurth). Music no longer has to be possessed – access through subscription is now the gateway to the entire music catalogue. The cost for diversity is, therefore, drastically reduced. However, Sinclair et al. expect that a certain search cost will remain. Because of these search costs, streaming platforms develop personalized algorithmic recommendation features with the help of algorithmic recommender engines, which should make it easier for users to navigate through the flood of titles and playlists.
“Streaming is going mainstream”, proclaims journalist Bas Grasmayer (1). On his blog musictechxfuture, he deals with the question of whether the status of music as “Social glue of society” or as an expression of culture is lost through algorithmic curation (Grasmayer). Since streaming and algorithmic curation of content are relatively new phenomena, research has increased considerably (Datta et al.), (Sinclair et al.), (Aguir et al.). However, the analysis of music recommender engines is mostly conducted regarding them either increasing or decreasing diversity and discovery (Tang et al.).
We aim to investigate whether Spotify is impacting our musical horizons by comparing our perceived music taste to Spotify’s suggestions curated by their recommender engines over time. For these purposes, we used data from our four Discover Weekly, Yearly Wrapped and Hidden Side playlists, retrieved through Spotify Statistics.
Spotify and its Influence on Music Reception
Streaming
The term streaming describes online access to digital data streams without having to download their respective files. The operators of music streaming platforms such as Spotify use storage space in clouds and make music available as a stream in their music titles. Finally, the user can access these music titles via the streaming platforms, select a title and thus initiate a stream (Webwise 1). Music consumption via streaming has substantially modified listener-music interaction which shall now be explained by taking a closer look at today’s music reception processes.
Music Reception
There are numerous studies on the topic of music reception. Until the 1970s, a cultural-critical evaluation of listening situations was carried out (Adorno 1962). Unlike Adorno, Rauhe (1975) presented the first hearing typology that did not seek an “appropriate” reception of music. Finally, Behne (1986) distinguishes between unconscious and conscious hearing. The fact that music now can be consumed individually and without being tied to a specific time, which is made possible by dematerialization, makes it an appreciated leisure activity today (2).
There are numerous studies on the topic of music reception. Until the 1970s, a cultural-critical evaluation of listening situations was carried out (Adorno). Unlike Adorno, Rauhe presented the first hearing typology that did not seek an “appropriate” reception of music. Finally, Behne (1986) distinguishes between unconscious and conscious hearing. The fact that music now can be consumed individually and without being tied to a specific time, which is made possible by dematerialization, makes it an appreciated leisure activity today (2). However, these new framework conditions also call for new and more differentiated listening typologies. One of the most extensive studies in Europe by Facit Media Efficiency, Age of Ears attempts a modern hearing typology. The central finding of the study was that a shift towards the digital is taking place in the reception of music (Facit Media Efficiency). In addition to the digitalization of reception channels, the contexts in which individual music titles are consumed are also changing. Physical sound carriers have so far determined the order in which titles are received. Accordingly, the album was a stringent concept developed by the musician (Knolle 13). As a result of digitization, however, a fragmentation of the albums can be observed especially in streaming services such as Spotify and Applemusic. Here concepts such as mood, genre or personal taste in music play an essential role in algorithmic curation of playlists (Spotify 1). Due to Spotify’s position in the market and the high number of users, Spotify is suitable as an object of investigation for determining changes in music reception and discovery processes.
Spotify
Today, Spotify is the global market leader in music streaming services with a market share of 43% (IFPI 2017) and is available in 61 countries (Spotify). According to Spotify around 140 million people worldwide actively use their platform. The company’s business model is based on the content principle. Wirtz summarizes this model as follows:
“(The) collection, selection, systematization, compilation (packaging) and provision of content on one’s own platform. The aim is to make content simple, convenient, visually appealing and accessible online for users”
— Wirtz, 219
Accordingly, Spotify’s service includes not only the provision of access to music but also its organization. To this end, features such as editorially curated playlists, for example by genre, mood or user situation, are presented to the user. In addition, algorithmic recommendation systems help the user navigate through the approximately 30 million music titles and 2 billion playlists that the Spotify library currently contains (Spotify).
Recommender Engine
Various algorithmic support procedures have been developed in the area of music information retrieval (the administration and sorting of music titles). In addition to automatic genre classifications and the identification of artists based on an audio signal (Shazam), the recommender engines are particularly worth mentioning in connection with Spotify as they guide music discovery.
Various algorithmic support procedures have been developed in the area of music information retrieval (the administration and sorting of music titles). In addition to automatic genre classifications and the identification of artists based on an audio signal (Shazam), the recommender engines are particularly worth mentioning in connection with Spotify as they guide music discovery.
Recommender engines are assigned to the discipline of artificial intelligence because they are based on adaptive algorithms. Their purpose is to relieve the user of the evaluation of an object that was unknown to him or that has not yet been evaluated. Recommender engines can, therefore, be understood as tools that allow the user to create a personalized order of their collection. A distinction is made between Collaborative Filtering, Content-Based Filtering and Hybrid Systems (Burke).
In order to increase navigation and discovery possibilities in Spotify’s extensive streaming library, the music service purchased Echo Nest in 2014, a database that has collected and analyzed extensive data on user behaviour during music consumption. This includes information on how long songs are played and which songs are shared. Using this data, Spotify has developed several discovery features. As part of our investigation, we analyzed the following features: Discover Weekly, Yearly Wrapped Playlist and Hidden Side, which will further be elaborated in our methodology.
Algorithmic Identity and Black Box Spotify
“When we work with our marketing partners, we use the full power of our platform to deliver the right messages to the right audiences.” — Spotify
Before we go into the methodology, it is important to mention, what we base our claims of Spotify’s possible impact on our musical horizons on. Spotify works through algorithmic recommendations systems, the nature of which can be compared to “black boxes” (Eriksson 99). The algorithms of these systems are unreadable and opaque besides being well hidden and protected trade secrets (Yeung 26). Algorithmic decision making techniques can work as a design based control and are designed to hyperpersonalize the individual users’ informational choice context (Yeung 118). Thus, Spotify can use algorithmic decision-making techniques to influence our behaviour. These very subtle nudges push user choices in directions preferred by the choice architect through processes nearly invisible to the Spotify user. According to Yeung’s “hypernudge” theory, the platform may shape the user’s individual decision-making process to serve its interests. Through the constant capture of the data, the algorithms of Spotify assign their users an algorithmic identity, inferring categories of identity on users while computing not only who the user is believed to be but also what content they want, creating a feedback loop in which the algorithm ultimately has the upper hand of control (Cheney-Lippold 168). Our research is trying to pick up on and add to existing studies about Spotify’s recommendation algorithms by examining how the algorithmic recommendations actually affect our music taste over time and if they are trying to keep us in a filter bubble or expand our horizons.
Methodology
Our aim is to investigate whether Spotify is impacting our musical horizons by comparing our perceived music taste to Spotify’s suggestions curated by their recommender engines over time.
Source of Data

The primary concern when working with Spotify is the permeability of the platform. The lack of transparency and retrievability of user data urged us to rely on an intermediary – Spotify Statistics. This web app provides the user with “insight into how they listen to music.” Despite a multiplicity of similar web apps, Spotify Statistic’s emphasis on genre distribution appeared to better complement our research incentive. Based on your Spotify playlist, Spotify Statistics offers different variables of analysis such as genre and artist distribution charts, “quick facts” such as average BPM (Beats per Minute) or length of track and mood distribution. In the interest of answering the research question more adequately the data processing solely focused on genre distribution.
Data Object
Once established that the data processing would gravitate exclusively around genres it was necessary to determine the most representative playlists to use and the time period over which they should be analyzed. We decided to analyze Spotify’s generated playlist Wrapped which provides the user with a compilation of their most listened songs in one year. We also focused on the Hidden Side playlist which, alongside Wrapped, provides the user, based on their genre taste, with a list of songs that they could potentially like. Finally we also analyzed Spotify’s Discover Weekly playlist, an algorithmically curated, personalized playlist based on user’s consumption behavior, automatically deleted and recreated every Monday (Spotify). Furthermore, the extent of the data analysis was limited to the year 2017 and 2018 due to a lack of previous and future data available. All the data was gathered from the Spotify accounts from the four researchers. The aim was to look at the genres of both songs that we had listened too recurrently and songs that are suggested over time and see if there is a change in our musical horizon.

Data Collection
Due to the nature of the data, being a secondary source, it was necessary to critically engage with it before analyzing it. As it appears here the genre distribution is highly specific, which despite being interesting, skews the distribution in itself. To counteract this effect and redistribute the genre more adequately researcher had to sub summarized genres under anchor terms which were rewritten with the process of reduction “into a concise, descriptive form limited only to the content” (Mayring 61). The process being highly subjective, the researchers attempted to be as objective as possible verifying the genre’s definition through everynoiseatonce in order to best fit them to a representative category.
Data Results
From this process emerged a recalibrated dataset from which top genres could be extracted in order to be compared over time.

Analysis of the Results
The extracted data shows a progression in our music taste and what Spotify recommends based on our streaming habits in order to create an algorithmic identity.
Mode of Analysis
We can see that each of us prefers some genres over others with slight changes over time. We wanted to present our data in a form that would visualize this progression in the most apprehendable way. So we chose to create a compilation mix track that represents results for each person, we then added a commentary to compare the results and anticipate how the perception our music taste differs from Spotify’s recommendations.
Songs used in the music mix were selected from the website everynoise.com according to the 5 most liked genres regarding our discover weekly and wrapped playlists. This website offers an extensive map of all music genres, each one has a song that represents it. We then created a snippet of each song and connected them in a way that shows the progression in our music taste.
The track starts with genres that were in our top five list only in 2017. Genres that we consistently listened to in both 2017 and 2018 and were also recommended to us by this week’s Discover weekly playlist are in the middle of the track, creating the basis of our music taste. The next part features genres we listened to only in 2018. The track is ending with genres included in recommendations made by Spotify’s Discover Weekly playlist that we have never listened to before. (Figure 1)
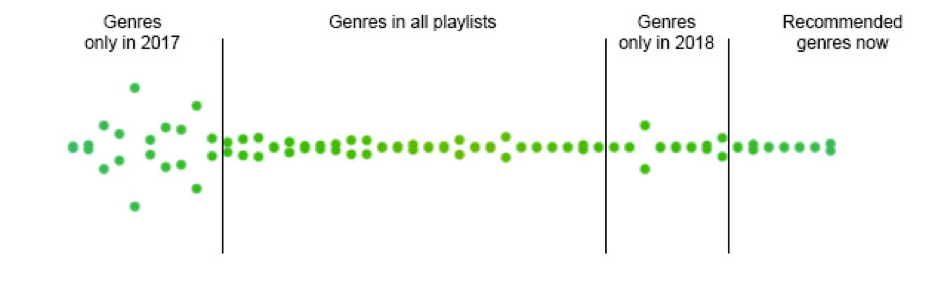
Figure 4: Song distribution in a mix track
Visual Representation of Results
Participant A
— Participant A, 2019
The beginning I loved, it was me, the end also. However I HATED the middle part, I wanted to take me earphones off. It is really bizarre because that part is supposed to represent me the most. I guess I am quite an eclectic listener.
Participant B
— Participant B, 2019
“The playlist resembles my music horizon throughout the years well. I can hear exactly when I moved back to Germany from Spain and suddenly stopped listening to the high amount of latin songs.”
Participant C
— Participant C, 2019
I thought it was accurate for the most part, I was bobbing my head along to every song. I also really got into musicals in 2018 and it was nice to see that translated into my music identity. However there was latin music at the end a genre that I really dislike.
Participant D
— Participant D, 2019
“My mix is very accurate in its representation of what was listened to on my account. There is a wide range of genres because I used to share my account. Recommendation that seems slightly out of place for me is country”.
The results suggest that Spotify considers our listening habits when recommending music. However, its recommendations are not keeping us in filter bubble as we first thought, but rather try to push us out of it slightly without disrupting our user experience. The Hidden Side 2017 playlist hasn’t influenced our music taste between the years 2017 and 2018. It would take longer more exhaustive research to unwrap how recommendations truly shape our music taste by following most popular influencing playlist Discover Weekly by weekly data collection. With the data available we cannot determine if recommendations are based purely on our listening habits or if Spotify is trying to force its own agenda upon us.
Limitations
Despite conclusive results, there were several limitations to this project. Due to the limited amount of time, creating new Spotify accounts was not viable as recommendation algorithms on Spotify require several weeks of user data to work. Thus, solely working with the contributors’ existing accounts was restrictive in the exploration of Spotify’s recommendations and music discovery algorithms. Another obstacle was the small amount of user data publicly accessible due to the black-box nature of Spotify which pushed the research to rely on secondary data sources such as Spotify Statistics and Spotify Wrapped for its data collection. The way those sources process, code and categorize the data remains obscure, which makes the data less transparent. It is possible to obtain all the data Spotify holds on you through its new privacy tool, but it takes four weeks for Spotify to provide the information. The data collection could have had the prospect to offer valuable findings and far broader insights using first-hand data collection methods. Another limitation is that the second-degree researcher does not know what kind of tests Spotify runs on the profiles and how that affects our recommendations. We agree for Spotify to use our data for tests, as soon as we sign up. Because of the scope of the blog post, it is not possible to address all aspects of the analysis nor the extensive theoretical frameworks needed.
Additionally, the categorized genres are subjective to an extent as they are based on the researchers’ pre-conditioned notions and definitions of the genre. Thus, it is arguable that the classification process of the genre categories is subjective to a certain extent and not free from interventions. Furthermore, using the category “genre” has been criticized in several sociological studies of music. They suggest decreasing importance of genre categories (Silver 1).
Conclusion
This paper tried to investigate whether Spotify is impacting our musical horizons by comparing our perceived music taste to Spotify’s suggestions curated by their recommender engines over time. We first offered an introduction into the relevance of streaming services using the example of Spotify and their recommendation engines in particular. Due to the little amount of user data publicly available we relied on the data provided by Spotify statistics. Focusing on the category of genres recommendation features such as Yearly Wrapped, Hidden Side and Discover Weekly were compared and their progression over time was analyzed and visualized. We were able to analyze if our music taste progresses over time, but the actual ways Spotify is interfering remained opace. Further research over a longer time, based on a far larger set of data will therefore have to be conducted. Additional analyses of biases and nudges based on Spotify’s algorithms are necessary.
Bibliography
ADORNO, Theodor W. Einleitung in die Musiksoziologie. Zwölf theoretische Vorlesungen. Suhrkamp, 1962.
AGUIR, Luis; Waldfogel, Joel. As streaming reaches flood stage, does it stimulate or depress music sales? International Journal of Industrial Organization.1-30. 2017.
BURKE, Robin. Hybrid recommender systems: Survey and experiments. User modeling and user-adapted interaction, 12(4):331–370. 2002.
CHENEY-LIPPOLD, John. “A New Algorithmic Identity: Soft Biopolitics and the Modulation of Control.” Theory Culture Society. 28.6. 164–181. 2011.
DATTA, Hannes; Knox, George; Bronnenberg, Bart J. Changing Their Tune: How Consumer’s Adoption of Online Streaming Affects Music Consumption and Discovery. Marketing Science, 2017.
ERIKSSON, Maria and Rasmus Fleischer, Anna Johansson, Pelle Snickars and Patrick Vonderau. Spotify Teardown – Inside the Black Box of Streaming Music. MIT Press. 2019.
GRASMAYER, Bas. “The Moby Problem: Open letter to Matt Ogle’s successor at Spotify.“ Musicxtechxfuture, 2017. https://northcutt.com/digital-marketing/filter-bubble-not-really-trynovelty-bubble/
HERRFURTH, Konrad. “Im Netz spielt die Musik. Rezeptionsstudie zu Einfluss und Wirkung cloudbasierter On-Demand-Streaming-Dienste auf das altersspezifische Musiknutzungsverhalten.” info7, vol. 28, no. 1, pp. 55 – 60. 2012.
IFPI. International Federation of the Phonographic Industry (Hrsg.). Digital Music Report 2017 [online]. In: Bundesverband Musikindustrie. 2017. http://www.ifpi.org/ content/library/dmr2009.pdf
KNOLLE, Niels. Musik im Zeitalter ihrer Digitalisierbarkeit. In: Musikforum , 03, 8-14, 2008.
MAYRING, Philipp. Qualitative Inhaltsanalyse. Grundlagen und Techniken. Beltz Verlag. 2003.
SILVER D, Lee M, Childress CC. Genre Complexes in Popular Music. PLoS ONE 11(5): e0155471.2016. https://doi.org/10.1371/journal.pone.0155471
SINCLAIR, Garry, Tinson, Julie. Psychological ownership and music streaming consumption. Journal of Business Research 71, 1–9. 2017.
TANG, Muh-Chyun; Yang; Mang-Yuan. Evaluating Music Discovery Tools on Spotify: The Role of User Preference Chracteristics. Journal of Libary and Information Studies, 1-16. 2017.
WEBWISE. What is streaming? BBC, 10th October 2012. http://www.bbc.co.uk/webwise/guides/about-streaming
WIRTZ, Bernd W. Medien- und Internetmanagement. 6. überarb. Auflage. Gabler, 2009.
YEUNG, Karen. Hypernudge: Big Data as a mode of regulation by design. Information, Communication & Society, 20:1, 118-136, 2017.
Figure Table
Figure 1: Spotify Statistics
Figure 2: Spotify Statistics
Figure 3: Figure 3: Genre Ranking all Participants (% relative to the overall segment)
Figure 4: Song distribution in a mix track