What are the odds of a full acceptance of the Big Data powered xG model in modern football?
Big Data in world football is on the rise. A plethora of clubs use data analysis to optimize their performance. The question is whether this kind of data driven approach doesn’t drown out other kinds of research. Are there ways in which traditional analysis is lost? And do the big numbers take the place of other valuable types of research?
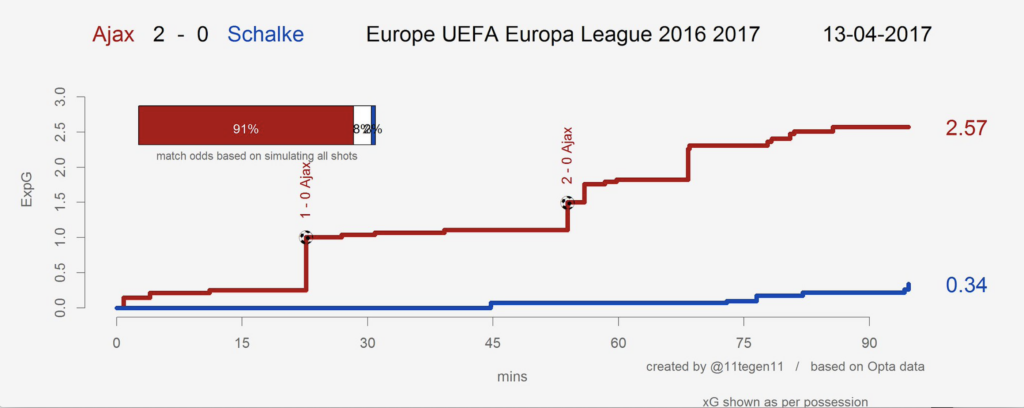
There are almost too many ways to describe the role of Big Data in our everyday lives. Take some examples in: ‘Big Data is everywhere today (Bismart, n.d.), ‘Big Data is all around us’ (Ricoh 2016), or, in a bit more nuanced form, ‘The era of Big Data is well underway’ (Boyd & Crawford 2012, 663). Although dated, the latter quote is a good starting point to discuss what Big Data does for all different fields it encompasses and in which it operates.
In their article ‘Critical Questions for Big Data’, Boyd and Crawford (2012) look at the phenomenon of Big Data in ways it can do good or harm. Through analyzing the different ways it operates in various fields, they aim to facilitate discussion where Big Data does well and where it can improve. Looking at their first point of discussion, they start with stating that Big Data changes the definition of knowledge. “Big Data reframes key questions about the constitution of knowledge, the processes of research, how we should engage with information, and the nature and the categorization of reality” (Boyd & Crawford 2012, 665). It is not only about how big the data set is, but Big Data also refers to a computational turn in thought and research. This is often not just a positive turn though, as it contains several drawbacks. As a key pointer, they warn Big Data discussion often sidelines other types of discussion. They state that there are other methods as to why people do, write, or make things that simply get lost in the sheer volume of numbers (Boyd & Crawford 2012).
‘The Future of Football’
One of these ways discussion is lost is in a similar computational turn in the world of football analysis. By no lack of surprise, Big Data analysis has found its way into the world of sports and has subsequently taken football discourse by storm (Marr, n.d.). One of the most pervasive ways is through the xG (expected goals) model (Kelly 2019). To provide a solid definition: “an expected goals model tries to estimate the probability of any given shot being converted to a goal based on various different factors describing the shot” (Anzer & Bauer 2021, 2). By looking at football from a statistical point of view, analysis can be performed whether a player or team does well based on key parameters, which gives the ability to predict further behavior (Bundesliga 2019).
Many believe this to be the future of football (Marr n.d.). Even scholars argue this kind of model brings a positive extended level of analysis to the world of football. For example, Rathke (2017) states various plusses of the usage of the model. Most notably, he mentions that Danish Club Fc Midtjylland won their first Danish League title based on judging players based on their xG data. Although their methods have been kept secret, Rathke (2017) mentions that their success shows the benefits of the model. Furthermore, he quotes football analysis website 11tegen11 by saying that xG is in fact a good predictor of a team’s performance. To give an indication on global usage, data science company StatsPerform (n.d.) states that more than 500 teams worldwide make use of their data driven model in world football.
Nuance lost in 1’s and 0’s
However, there is a growing sentiment that this Big Data analysis moves exactly in the direction which Boyd and Crawford predict; there is too much that is lost in numbers and there are additional factors that cannot be considered by the model. As Arastey (2018) states: “The xG model is only as good as the factors being input into its calculations.” Scenarios that can change the outcome of a game, such as a goalkeeper that is off balance or the dip of the shot, are not considered by the model and exclude qualities of a player that would in this case be overlooked. The model looks at averages, numbers, and finds patterns. Qualitative abilities of players that set them apart from their peers get lost in a stream of 1’s and 0’s.
Additionally, there are definitive methods being excluded from conversation. Take the ‘eye test’ for example, a method used by professionals to look at the game of football within general context and their own professional understanding of the game. Jobs4Football (2021) state that academies and football organizations have been focusing on stats and ‘xModels’ to such a large extent that experts from the game will be excluded in conversation about improvement. “I just fear that we will neglect what our eyes see and in a conversation amongst friends use the argument that this play did or did not perform because of xT” (xT meaning expected threat, another model to predict action), they voice as concern (Jobs4football 2021).
Although the xG model (and similar models) have a large backing in the world of football, they have still not found themselves as the protagonist in main sports discourse. Whether that will be the case in the future has yet to be seen. There is no arguing that the model has merit; even official leagues have introduced the model to the masses, like the aforementioned Bundesliga (German National League) example. xG is here to stay and has the ability to transform football to the next level. However, it remains to be seen whether it can accomplish that without drowning out valuable qualitative analysis. Various older methods still have incredible value to add to the game but will be excluded due to a computational turn. Additionally, data without interpretation lacks tact and guidance; two factors which are essential in sports analysis.
To quote Boyd and Crawford (2012) one last time: “Do numbers speak for themselves? We believe the answer is no.”
References
Anzer, G. and P. Bauer. 2021. “A Goal Scoring Probability Model for Shots Based on Synchronized Positional and Event Data in Football (Soccer).” Frontiers in Sports and Active living. 3:624475. doi: 10.3389/fspor.2021.624475
Arastey, Guillermo. 2018. “What are expected goals (XG)?” SportPerformanceAnalysis. May 22, 2018. https://www.sportperformanceanalysis.com/article/what-are-expected-goals-xg
Bismart. n.d. “Big Data is Everywhere: 5 Ways It’s Used in Your Everyday
Life.” Accessed on October 2, 2021. https://blog.bismart.com/en/big-data-is-everywhere
Boyd, D., and K. Crawford. 2012. “Critical Questions for Big Data.” Information, Communication & Society, 15:5, 662-679, DOI: 10.1080/1369118X.2012.678878
Bundesliga. 2019. “xG stats explained: the science behind Sportec Solutions’ Expected goals model.” October 2, 2021. https://www.bundesliga.com/en/bundesliga/news/expected-goals-xg-model-what-is-it-and-why-is-it-useful-sportec-solutions-3177
Jobs4Football. 2021. “Statistics and the ‘eye test.” Augustust 27, 2021. https://jobs4football.com/statistics-and-the-eye-test/
Kelly, Ryan. 2019. “What is xG in football & how is the statistic calculated?” Goal. October 2, 2021. https://www.goal.com/en/news/what-is-xg-football-how-statistic-calculated/h42z0iiv8mdg1ub10iisg1dju
Marr, Bernard. n.d. “How Big Data and Analytics Are Changing Football.” SmartDataCollective. Accessed on October 2, 2021. https://www.smartdatacollective.com/how-big-data-and-analytics-are-changing-football/
Rathke, Alex. 2017. “An examination of expected goals and shot efficiency in soccer.” Journal of Human Sport and Exercise 12, no. 2 (March):514-529.
Ricoh. 2016. “Big Data is all around us.” September 1, 2016. https://www.ricohediscovery.com/blog/big-data-is-all-around-us
Statsperform. n.d. “Working with 500 teams.” Accessed on October 2, 2021. https://www.statsperform.com/team-performance/football-performance/