“I want to show you something”: Cinetree as an alternative to automated recommendations
A boutique among department stores
Last year, Dutch actress Hanna Verboom launched a new video on demand service, Cinetree. It distinguishes itself from other popular movie streaming sites by offering a very limited yet carefully curated selection of films. When entering Cinetree, members get ten movies to choose from, and every three days the one that has been online the longest is replaced. It does not show the latest releases that you might find highlighted on for example Netflix; instead there is a team of well-known and respected editors that personally recommend both newer and older titles. Filmmakers, photographers, actors and journalists personally introduce their choice of film and members can watch the movie right away. Users pay € 5 per month (the standard Netflix package is € 9,99), or can decide to pay € 3 to ‘rent’ one specific title.
The motivation behind the new platform is clear: in a web that is clogged with content, Verboom and her team wish to offer a guide towards quality. Their selection claims to have more depth in terms of content than the average amusement-driven movie (Dijksterhuis). Of course, the platforms that Cinetree competes with do offer their own forms of recommendation. In fact, algorithmic recommendation systems have developed into an important element within the new media environment, and are constantly looking to fine-tune and improve the suggestions that users receive. YouTube, Spotify, Amazon, and Netflix itself: they all offer several lists of popular content as well as personalized tips.
Recommended for you
Looking at these continuously updated and personalized suggestions, there are a few things to be considered. Firstly, it is important to be aware of the factors that determine the content selected for users. With categories such as ‘trending’, ‘most watched’ and ‘best rated’, many online services rely on popularity as an indicator for what their users want. Yu et al. highlight the impact of popularity on the distribution of views: with the opportunity to pick from a wide selection of files, “23% of the objects account for 80% of the accesses” (338). On YouTube it is even more skewed: “10% of the top popular videos account for nearly 80% of views” (Cha et al. 3). Even though the internet provides the opportunity to practically view whatever one wants, these dominant platforms seem to create a type of consumption that is similar to that of mass media.
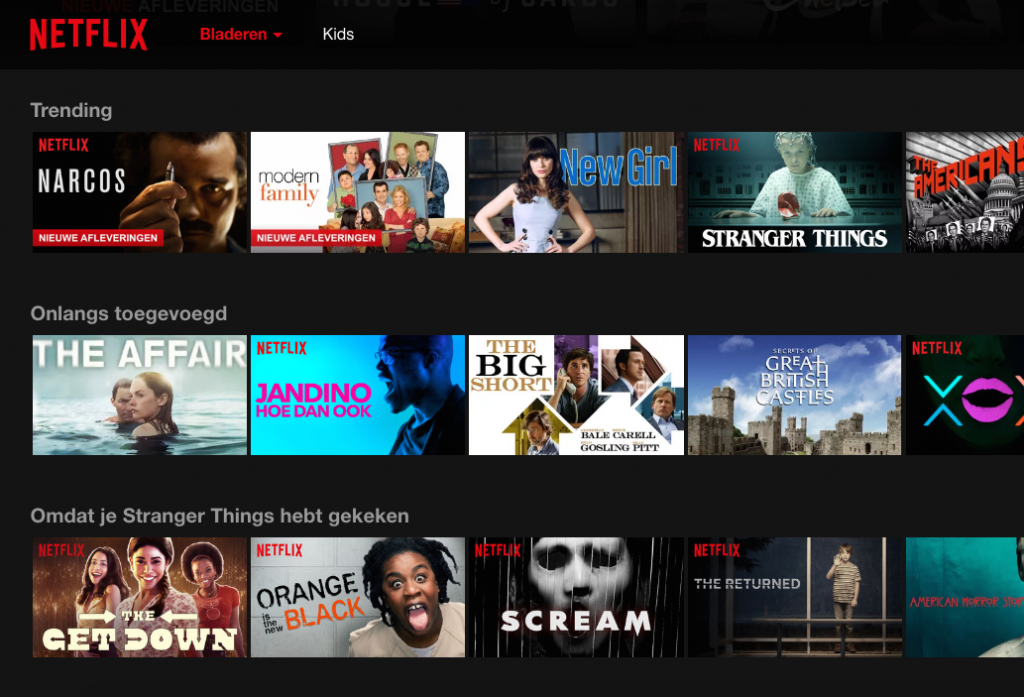
Netflix recommendations
In the recommendation of content, individual preferences are of course not disregarded. Based on personalized profiles, platforms show links that are deemed interesting for that person. Developing the algorithms that generate these lists has become key to keep customers satisfied. Using the approach of “Collaborative Filtering”, predictions are made based on similarity among users and items in terms of ratings, clicks, views, purchases, etc. (Cremonesi, Koren and Turrin 41). Spotify’s Discover Weekly, for example, has shown to be a great success (The Guardian). Incorporating all sorts of feedback into the mechanism, recommendations become increasingly accurate and users face less and less mismatched information.

Wikipedia animation on Collaborative Filtering
Especially in the online news realm, this phenomenon has been widely talked about and problematized as the Filter Bubble. This term was coined in 2011 by Eli Pariser in his book The Filter Bubble: What the Internet Is Hiding from You, in which he argues that it “closes us off to new ideas, subjects and important information” (USA Today). Selective exposure in this field can lead to a constant reinforcement of existing opinions, and some developers and researchers work on countering this and finding an optimal diversity level (Liao & Fu 2360). But what about selectivity in the entertainment industry? Only watching the type of movie that a person already knows they like, or hearing music from similar genres – does that not negate the power that art can have? Being exposed to a variety of stories, movements and styles contributes greatly to personal development that goes beyond cultural ‘taste’. Cinetree is built upon this principle and wishes to share these stories, taking into account current themes and thus putting the view-broadening qualities of film central to their services. In this scenario, however, the selection of stories includes a bias resulting from the limitations to the composition, knowledge, tastes and views of the board of editors. The risk of creating a separate bubble should therefore be noted.
A human touch
How do users value an algorithm’s prediction compared to a human being’s personal and contextualized recommendation? The Digital Democracy Survey confirms that “Personal recommendations […] play a major role in buying decisions” (17). Messing & Westwood describe how this “social endorsement” of content increases the chance that people select particular sources (2). Recommendation systems continue to improve, but online discussions labeled Why do the movies on Netflix suck? (McGauley) and 59 reasons Netflix streaming is extremely disappointing (Perpetua) indicate that the service ‘for the masses’ is far from perfect.
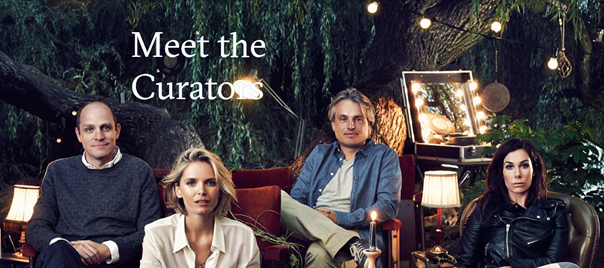
Some of the Cinetree curators
Do we need a ‘human touch’ to ensure a valuable online viewing experience? In his blog about algorithmic versus human curation, Banks argues that “When it comes to long-form video content platforms, more sophisticated personalization driven by improved machine-learning will likely triumph”. Services like Cinetree claim the opposite and continue to try and open our eyes to what is beyond our own bubble, however sophisticated and comfortable it might become.
Bibliography
Banks, Braeden. “Algorithm v. Human Curation: Is the Debate Really Settled?” Digiflare. 2016. 12 Sept. 2016. <http://www.digiflare.com/algorithm-v-human/>
Cha, Meeyoung et al. “I Tube, You Tube, Everybody Tubes: Analyzing the World’s Largest User Generated Content Video System.” Proceedings of the 7th ACM SIGCOMM Conference on Internet Measurement. 2007. 1–14. 18 Sept. 2016. <http://doi.acm.org/10.1145/1298306.1298309>
Cremonesi, Paolo, Yehuda Koren, and Roberto Turrin. “Performance of Recommender Algorithms on Top-N Recommendation Tasks.” Proceedings of the Fourth ACM Conference on Recommender Systems. 2010. 39–46. 14 Sept. 2016. <http://doi.acm.org/10.1145/1864708.1864721>
Digital Democracy Survey. Deloitte LLC, 2015. 14 Sept. 2016. <https://www2.deloitte.com/content/dam/Deloitte/global/Documents/Technology-Media-Telecommunications/gx-tmt-deloitte-democracy-survey.pdf>
Dijksterhuis, Edo. “Cinetree wil ‘de boetiek onder de VOD-platforms’ zijn.” Filmkrant. 2014. 14 Sept. 2016. <http://www.filmkrant.nl/nieuws_2014/11241>
“First Monday: What’s on Tap This Month on TV and in Movies and Books.” USA TODAY. N. pag. 18 Sept. 2016. <http://www.usatoday.com/money/companies/management/monday/2011-04-29-first-monday-business-media-in-may_n.htm>
Liao, Q. Vera, and Wai-Tat Fu. “Beyond the Filter Bubble: Interactive Effects of Perceived Threat and Topic Involvement on Selective Exposure to Information.” Proceedings of the SIGCHI Conference on Human Factors in Computing Systems. 2013. 2359–2368. 14 Sept. 2016. <http://doi.acm.org/10.1145/2470654.2481326>
McGauley, Joe. “Why Do the Movies on Netflix Suck?” thrillist. 2016. 14 Sept. 2016. <https://www.thrillist.com/tech/nation/why-do-the-movies-on-netflix-suck>
Messing, Solomon, and Sean J. Westwood. “Selective Exposure in the Age of Social Media: Endorsements Trump Partisan Source Affiliation When Selecting News Online.” Communication Research (2012): 1-22. 14 Sept. 2016. <http://crx.sagepub.com/content/early/2012/12/28/0093650212466406>
Pariser, Eli. The Filter Bubble: What The Internet Is Hiding From You. Penguin UK, 2011.
Perpetua, Matthew. “59 Reasons Netflix Streaming Is Extremely Disappointing.” BuzzFeed. 2014. 14 Sept. 2016. <http://www.buzzfeed.com/perpetua/netflix-is-disappointing>
“Spotify’s Discover Weekly: How It Works.” The Guardian. 18 Sept. 2016. <https://www.theguardian.com/spotify-discover-weekly/2016/aug/01/spotify-discover-weekly-how-it-works>
Yu, Hongliang et al. “Understanding User Behavior in Large-Scale Video-on-Demand Systems.” Proceedings of the 1st ACM SIGOPS/EuroSys European Conference on Computer Systems 2006. New York, NY, USA: ACM, 2006. 333–344. 14 Sept. 2016. <http://doi.acm.org/10.1145/1217935.1217968>