Artificial Intelligence: an objective method for measuring beauty?
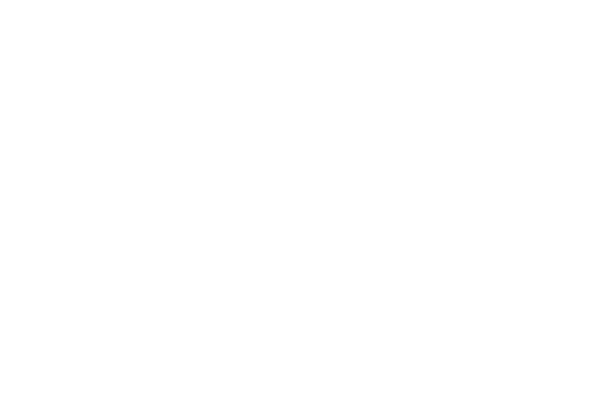
© Anna Subbotina / Adobe Stock & © BUYOUROBOT
Last August, a team of biogerontologists and data scientists, organised the First International Beauty Contest Judged by Artificial Intelligence ever: Beauty.AI (Beauty.AI). With the support of major (tech)companies, like EY, NVidia and Microsoft, a team of scientist developed various deep- and symbolic learning algorithms that should have been able to measure ‘the beauty of humans’ unbiased (PRweb UK). Should, since the results of the contest were not as unbiased as you might expect from a sophisticated mathematical algorithm. The robots judging the contestants on various facial features had a strong preference for Caucasoid participants. But could we expect an algorithm, created by humans, to be as objective and unbiased as the mathematical approach feigns?
The deployment of algorithms to make classifications is not unique to this beauty pageant. Machine-based learning is commonly used in spam filters, loan qualification, insurances and credit scoring (Burrell 1). Information scientist, Jenna Burrel, researched possible inequality and discrimination inflicted by these algorithms. She states that algorithms are “opaque”: the input data may be completely, or partial unknown, and the output generated by the algorithm is seldom questioned (Burrell 1). On top of the opaqueness of the in- and output, the algorithm itself is a specialist piece of software, hardly accessible to a layman.
John Symons and Ramón Alvarado also question the trustworthiness of Big Data. They acknowledge cognitive and social biases caused by human-centred interactions with Big Data (4). On top of that, they state that:
“In the broader social and political context, a pre-condition for understanding the potential abuses that can result from the deployment of Big Data techniques by powerful institutions is a careful account of the epistemic limits of computational methods.” (13)
So we should take into account the errors that are included in complex software like artificial intelligence. But what exactly happened with the Beauty.AI project?
Over 6.000 people from 100 countries participated in the beauty contest (Levin). The robots selected 44 winners in various age and gender categories, their faces most closely resembling “human beauty”. Only 1 of the 44 winners had a dark skin (Levin). According to the Beauty.AI chief science officer, the open source dataset used to the train the deep learning algorithms was not a representative composition of the diverse world population (Levin). Since there simply were not enough minorities included in the dataset, the algorithms were not able to learn to appreciate human beauty in other skin tones. But that is not the only reason why almost all winners in the contest had a Caucasoid appearance.
Deep learning is a form of machine learning, which relies on advanced computational models that break down data objects in multiple layers, continuously changing internal parameters to be able to detect certain representations in these layers (LeCun, Bengio, and Hinton 436). The adjustments of the internal parameters are made to correct for specific conditions a particular image may have: lighting, shade, composition and so forth. In this distinct contest, the algorithms would break down images in layers (vectors, patterns and pixels) and analyse the layers on representations of certain facial features, like wrinkles and symmetry (Beauty.AI). But how does an algorithm know whether certain features represent human beauty? According to an article on Motherboard, Beauty.AI learned algorithms to evaluate facial features using a database that consisted of pre-labelled images describing what was in the picture (Pearson). This form of deep learning is called (semi-)supervised deep learning, since the algorithms learn to recognise certain features based on human qualification (LeCun, Bengio, and Hinton 436). The algorithm then integrates these findings as generalisations in the algorithm itself. When the algorithm is applied on a “new”, unlabelled database, it will classify beauty based on the generalisations learned from human classification. Although a complex mathematical algorithm does analyse the pictures, it actually learned to classify based on the input of people.
In this particular pageant, both the input data and the algorithm itself were somewhat opaque, to speak in the words of Burrell (1). Although the input data was (partly)known, only after running the experiment the scientist learned that the database used was too small to develop an unbiased algorithm. In addition to the unrepresentative database, the human-centred interaction with the algorithm, (the manual labelling of images) as described by Symons and Alvarado (4), was biased by the unconscious predispositions of the scientist involved in qualifying the database. Both Burrel as well as Symons and Alvarado state that the output data generated by algorithms should be questions critically (Burrell 1; Symons and Alvarado 13). Luckily, the programmers involved in the Beauty.AI project did, and they are currently working on a 2.0 version with adjusted algorithms that should be able to detect beauty in multiple skin tones (PRWeb USA).
Although this AI beauty pageant is quite offensive and racist, the consequences of the pageant are quite harmless. In courtrooms in the United States of America, algorithms are used to predict if a defendant is likely to commit a future crime (Kirchner). The findings of the algorithms directly affect the incarceration of the convicts. The algorithms used, also seem to have a bias against black people (Kirchner). This case again, stresses the importance of thinking critically about the functioning of algorithms and the confidence that we can grant to their findings. As Symons and Alvarado state: “A clear sense for the nature of error in these systems is essential before we can decide how powerful they should become and how much trust we should grant them” (13).
References
Beauty.AI. “The First International Beauty Contest Judged by Artificial Intelligence”. Beauty.AI. 15 Sept. 2016. <http://beauty.ai>.
Burrell, J. “How the Machine ’Thinks: Understanding Opacity in Machine Learning Algorithms”. Big Data & Society 3.1 (2016).
Kirchner, Julia Angwin Surya Mattu, Jeff Larson, Lauren. “Machine Bias: There’s Software Used Across the Country to Predict Future Criminals. And It’s Biased Against Blacks.” ProPublica. 16 Sept. 2016. <https://www.propublica.org/article/machine-bias-risk-assessments-in-criminal-sentencing>.
LeCun, Yann, Yoshua Bengio, and Geoffrey Hinton. “Deep Learning”. Nature 521.7553 (2015): 436–444.
Levin, Sam. “A Beauty Contest Was Judged by AI and the Robots Didn’t like Dark Skin”. the Guardian. 13 Sept. 2016. <http://www.theguardian.com/technology/2016/sep/08/artificial-intelligence-beauty-contest-doesnt-like-black-people>.
Pearson, Jordan. “Why An AI-Judged Beauty Contest Picked Nearly All White Winners”. Motherboard. 15 Sept. 2016. <http://motherboard.vice.com/read/why-an-ai-judged-beauty-contest-picked-nearly-all-white-winners>.
PRweb UK. “Beauty.AI Announces the First International Beauty Contest Judged by an Artificial Intelligence Jury”. PRWeb. 15 Sept. 2016. <http://www.prweb.com/releases/2015/11/prweb13088208.htm>.
PRWeb USA. “Beauty.AI 1.0 Announces the First Humans Judged by a Robot Jury; Beauty.AI 2.0 to Be Launched Soon”. PRWeb. 17 Sept. 2016. <http://www.prweb.com/releases/2016/02/prweb13199572.htm>.
Symons, J., and R. Alvarado. “Can We Trust Big Data? Applying Philosophy of Science to Software”. Big Data & Society 3.2 (2016).