Netflix and Choose? Choice, Recommendation and Streaming in an Age of Algorithmic Culture
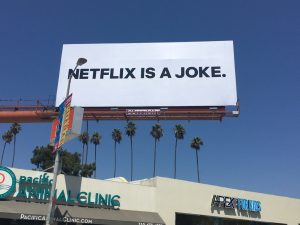
Recent Netflix advertising campaign. Photo courtesy of Claudia Eller at Variety Magazine: @Variety_Claudia
Have you ever had that moment on “Movie Night”, when the pizza is on it’s way, the ice cream is cooling in the freezer and all you need to do is decide on which dystopian thriller to watch (other genres are available)? That’s the fun part right? Wrong. Anyone who uses video streaming services for their latest film fix will agree that this has become a regular chore. The abundance of choice has become all too daunting resulting in streamers panic-watching, turning off or in some cases cancelling subscriptions (Gomez-Ubribe & Hunt, p.13:7). According to consumer research undertaken by Netflix in 2016 “a typical Netflix member loses interest after perhaps 60 to 90 seconds of choosing…the user either finds something of interest or the risk of the user abandoning [the] service increases substantially” (Gomez-Ubribe & Hunt, p.13:2). As the wealth of entertainment at our fingertips overflows, the harder it has become to take our pick. Accordingly, this blog will consider the website Cinesift alongside Netflix’s recommender algorithms and in doing so will deliberate the potential ramifications of collaborative filters on choice culture.
Logically if it can be agreed that having choice is good; so it can be assumed that having more choice is better. Psychologist Barry Schwartz disagrees with this theory and his research maintains that “increased choice can lead to decreased well-being” (“The Tyranny” p.2). This is particularly bad for persons he describes as “maximizers”; those who ultimately want to choose the best in any given situation (p.2). But who can blame them? I cannot. It is inherently woven into the fabric of my being a Millennial (see Gillett, 2015). However help is seemingly here in the form of a website that aims to combat the growing issue concerning what Schwartz defines as the “Paradox of Choice” (“The Paradox”).
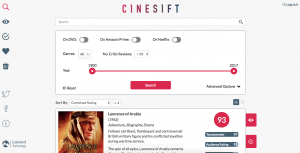
Screenshot of Cinesift (a product of Lakeland Technology) (Accessed 23.09.17)
Whilst the web is scattered with generally tame attempts at curing the movie-choice “paralysis” (“The Tyranny”, p.2), it is the clean interface of the Cinesift (Lakeland Technology) algorithm that firmly stands out. As well as combining a total of five respected ratings systems (The Tomatometer, Rottentomatoes Audience Rating, IMDB, Letterboxd & Metascore) into a mean average, Cinesift crucially incorporates your access and location capabilities, not forgetting your genre or era (year) preferences either. As a user you can switch on a “DVD”, “Amazon Prime” or “Netflix” filter as well as inputting your location to show only the highest-rated films logistically available to you. Other websites such as Whatisonnetflix and Flixroulette come close to a similarly respectable solution, however the defining factor that champions Cinesift is its ability to accommodate cross-platform recommendation whilst incorporating location-specific content. Additional features include watched-lists, wish-lists, favoriting and even trashing (where you can remove a film from appearing in the interface). However the benefits of the algorithm end here. So in that respect, does this tool solve the streamer’s “Paradox of Choice”? For the “maximiser” it may well do.
The fact that Cinesift doesn’t have any content indicates that its role cannot necessarily grow beyond that of film consultant or movie middle-man. Additionally the gaping flaw with the algorithm is although admirable and glossy, it doesn’t learn about the user like Netflix does. While I initially praised the features of Cinesift, admittedly after continued use, they quickly became a chore and one naively turned nostalgic for the lack of effort required with Netflix, momentarily forgetting my initial choice “paralysis”. The business goals of Cinesift are unclear but the same cannot be said for Netflix. This multi-billion dollar corporation is more than aware of the potential they possess to dominate the streaming arena but only if they can heal their algorithmic ailments.
With this in mind Netflix indeed have their work cut out for them and even in 2006 reached out to the public, offering a $1m award to whoever could solve their collaborative filtering crisis (see Vedrashko). While this was situated in a time of ratings and DVDs, Netflix reprogrammed their algorithm in the last year and decided to mimic Youtube using a simple “thumbs up” or “thumbs down” system. The quality of this new system has been largely successful with “thumbs” getting 200% more ratings than the traditional star-rating feature (Roettgers), but it has also come under some small scrutiny (Kasana). Netflix’s latest claim is that “the more titles you rate, the better [their] suggestions will be” (Netflix Help Center). This encouragement of increased interaction and divulging of preferences is essential if Netflix are to succeed in awakening paralysed users. But what is the fate for these ever-evolving algorithms or “codes with consequences” (Gillespie, p.26)? And how does this shape the future of choice?
The notion of the “black box” is repeated throughout algorithm literature (see Hallinan & Striphas, Joler & Petrovski, Gillespie, Sinha & Swearingen et al) and this concept is certainly fitting where Netflix is concerned. Holt and Vonderau highlight GAFA’s attempts to give users a look behind the curtain by releasing images of their data centers, and I believe Netflix’s funding of Gomez-Ubribe & Hunt’s academic paper is done so in a similar vein. While we are seemingly getting a privileged look into the inner-workings of these secretive companies, as users, we are still none-the-wiser. The everyman doesn’t understand the workings of the large servers pictured, and nor does he feel included by the confusing technical jargon of Gomez-Ubribe and Hunt’s algorithm explanations. In reality we are lulled into contributing to a supposed transparent entity with little idea where or how our input is being used. Indeed Sinha and Swearingen validify this when they state that “users like and feel more confident in recommendations [when] perceived as transparent” (p.2). This indicates that GAFA and Netflix are more than aware that transparency (or the illusion thereof) can translate to increased user contribution as well as trust and in turn, continue to fuel their future successes.
We already contribute and conduct much of our personal lives through our interactions with GAFA, so why not Netflix too? How long before Netflix is linked to Facebook and you are receiving recommendations based on arbitrary posts or pages you’ve liked? How long before your Amazon Echo overhears your interest in visiting London, only for you to then get recommendations to watch Notting Hill and Love Actually?
A woman sued Netflix in 2009 after concern her sexuality could be determined from her ratings choices (eg. rating gay-themed content). If made public she stated this knowledge would be detrimental to her and her childrens livelihood (Jane doe vs Netflix in Hallinan and Striphas p.125). This case emphasises how such minute user input can be subject to such a wealth of interpretation and thus inflames concerns surrounding privacy. For if user data is placed in the wrong hands; any number of disastrous consequences could arise.
Additionally we must also remember Netflix is a global corporation with financial gain at the route of its intentions. So therefore it is paramount to consider the power they possess in gauging our preferences and consequently the ability to control our tastes. Through a trusted illusion of transparency, a question of ethics has to be raised and the potential for biased recommendations become a possibility. For example, Netflix regularly pin their “Netflix Originals” on the front webpage where you cannot miss them. Even if they are failing in the critical film arena they are pushed to the front of your recommendations, “nudging” (see Thaler and Sunstein) you to watch them despite perhaps not matching your preferences. It is then easy to fathom that Netflix’s algorithms have the power to “skew to [their] commercial or political benefit” (Gillespie p.10) because “operation within accepted parameters does not guarantee ethically acceptable behaviour” (Mittelstadt et al p.1). Mittelstadt et al propose necessary “auditing” (p.13) as the solution to taming uncertainty surrounding corporate “Black Box” algorithms.
The transparency of Cinesift is somewhat clear: by inputting minor preferences the chore of choice can be quashed and one will be provided simply with the best filmic options legally available to us. The site even admits that it is “entirely automated” encouraging an ambivalent feeling of trust (Lakeland Technology). However it falls down as a solution to the choice paradox in the respect that these “highly-rated” films simply might not be in line with our tastes and therefore may not satisfy all of Schwartz’s “maximisers”. Conversely whilst Netflix may not have a full catalogue of the “highest-rated” content, the more we interact with their systems; the more likely we will be paired with “a few compelling choices simply presented” (Gomez-Ubribe and Hunt p.7) to suit our needs. If we consider that “two years in the world of algorithms is like centuries” (Joler & Petrovski), it looks likely that a future is near where Netflix solve, or at least dilute, the “Paradox of Choice”.
For now we are left in another dilemma. Do we stick with Cinesift and hope the “best” available is actually the “best” for us? Or do we succumb to the parting of personal data to the mythical recommendations of Netflix? And if so, how much personal information are we willing to part with to receive outstandingly accurate recommendations?
We are not necessarily envisioning a negative streaming future just an unnerving one. Recommender algorithms encourage continued discussion around where the line of privacy will be drawn and whether it will even be drawn at all. What is certain is the alarming reality of Hallinan and Stripha’s suggestion that engineers and their algorithms are fast becoming “important arbiters of culture” (p.131). They are certainly defining our culture but at what cost to our privacy?
I don’t want to watch a dystopian thriller anymore; I think I might already be in one.
References
Gillespie, Tarleton. “The relevance of algorithms.” Media technologies: Essays on communication, materiality, and society 167 (2014).
Gillet, Rachel ‘The book that inspired Aziz Ansari’s ‘Master of None’ shows how having too many options is screwing us up” (2015). Business Insider. businessinsider.com/how-modern-life-is-screwing-up-millennials-2015-11?international=true&r=US&IR=T Accessed 24 September 2017.
Gomez-Uribe, Carlos A., and Neil Hunt. “The netflix recommender system: Algorithms, business value, and innovation.” ACM Transactions on Management Information Systems (TMIS) 6.4 (2016): 13.
Hallinan, Blake, and Ted Striphas. “Recommended for you: The Netflix Prize and the production of algorithmic culture.” New Media & Society 18.1 (2016): 117-137.
Holt, Jennifer, and Patrick Vonderau. “‘Where the internet lives’: Data centers as cloud infrastructure.” Signal Traffic: Critical Studies of Media Infrastructures (2015): 71-93.
Joler, Vladen, and Andrej Petrovski. “Quantified Lives on Discount” (2016) Share Lab. labs.rs/en/quantified-lives/ Accessed 24 September 2017.
Kasana, Mehreen. “Netflix’s recommendation algorithm sucks”. (2017). The Outline. theoutline.com/post/1300/netflix-recommendation-algorithm Accessed 24 September 2017.
Lakeland Technology. Cinesift. cinesift.com Accessed 24 September 2017.
Lakeland Technology. “About” Cinesift. cinesift.com Accessed 24 September 2017.
Mittelstadt, Brent Daniel, et al. “The ethics of algorithms: Mapping the debate.” Big Data & Society 3.2 (2016): 1-21.
Netflix Help Center. “Netflix Ratings & Recommendations”. (2017). Netflix. https://help.netflix.com/en/node/9898 Accessed 24 September 2017.
Roettgers, Janko. “Netflix Replacing Star Ratings With Thumbs Ups and Thumbs Downs”. (2017). Variety. variety.com/2017/digital/news/netflix-thumbs-vs-stars-1202010492/ Accessed 24 September 2017.
Schwartz, Barry. “The paradox of choice.” (2004).
Schwartz, Barry. “The tyranny of choice.” Scientific American Mind 14.5 (2004): 44-49.
Sinha, Rashmi, and Kirsten Swearingen. “The role of transparency in recommender systems.” CHI’02 extended abstracts on Human factors in computing systems. ACM, (2002).
Thaler, Richard H., and Cass R. Sunstein. “Nudge: Improving Decisions About Health, Wealth, and Happiness.” (2008).
Vedrashko, Ilya. “Netflix Battles Paradox of Choice with $1m Award” (2006) Futurelab.
futurelab.net/blog/2006/10/netflix-battles-paradox-choice-1m-award. Accessed 19 September 2017.