Face the Bubble: Revealing How Facebook Selects Users’ News Stories
Introduction
The media content we are exposed to is becoming increasingly personalised (Borgesius 2). Personalisation in this sense can be explained as online media content tailored to an individual for it to have a more personal appeal to the user (Pariser). This idea gives way to a suggestion by Eli Pariser (2011) who coined the phenomenon: the “filter bubble”, a way of explaining how users’ content is tailored to them personally. It explains what is visible to users and what is not visible to them (Schaap).
There are two ways in which content is tailored to the user: firstly the user may select their preferences, called self-selected personalisation. The second is pre-selected personalisation based on algorithms which predict what content you would like based on past online behaviour (Borgesius 3). One of the concerns surrounding the filter bubble is the topic of autonomy referring to issues of users not being aware of what is self-selected and pre-selected content (Borgesius 3). Using the example of Facebook and how users may receive news stories on their Newsfeed, it is found that posts are a combination of what the user has chosen themselves and what Facebook is suggesting them.
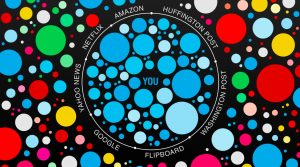
Visualising the filter bubble. Credit: Pariser (2015)
Eli Pariser questions the Internet’s motives in the title of his 2011 book What is the Internet Hiding from You? where he explains what is being kept outside of the filter bubble. We would like to explore the concept of the filter bubble from the ‘inside’. Our aim is to understand why users see what content they see. We would like to suggest the design of an extension revealing to users which Newsfeed posts are self-selected and/or algorithmically pre-selected. Regarding the discussion of autonomy and transparency, we would like to make users aware of what Facebook is suggesting to them. The question we then pose is: how can we understand the filtering process of posts on the Facebook Newsfeed?
Contextualising the Filter Bubble
Overwhelmed by the sheer abundance of online information, “personalised filters” help users view information relevant to their interests (Pariser). However, one can argue that the tailoring of content narrows “the way we encounter ideas and information”, preventing access to diverse information that can “challenge one’s existing beliefs” (Pariser; Resnick et al. 96). The term “filter bubble” develops from this understanding, as a concept that refers to this gathering of users’ online behaviour and interests to filter the content they receive on websites. This creates “invisible barriers” to diverse information and the potential to isolate the individual (Pariser; Maccatrozzo 391). The algorithm filters out information that the website deems “of little interest” for specific users, providing them with content they are expected to consume (Haim et al. 2).
Similar theoretical ideas to the filter bubble appeared in Sunstein 2009 and Gitlin’s 1998 work, as both fear citizens “unknowingly missing various pieces of information which prohibit individuals from being properly informed and rational democratic citizens” (Haim et al. 4). Bozdag and Hoven found that users online are typically unaware of the filters employed through algorithms (262). Filter bubbles are thus a problem due to their “non-transparent filters” that have “negative effects on user’s autonomy and choice” (Bozdag and Hoven 250-251).
In recent years the filter bubble has evolved as a key concept for theorists to research online personalisation, due to web companies striving to tailor their services to a user’s personal taste and thus limiting user’s autonomy (Resnick et al.; Nguyen et al.; Bozdag and Hoven; Garimella). On one hand, users are being exposed to content that supposedly interests them and can even “learn and explore” something they may have not seen. (Nguyen 678).
Yet, a key critique of this concept is that we “actively choose” the content we receive, just as we have done with older media technologies – television, newspapers – attempting to debunk the concept as a new threat to user’s autonomy (Borgesius et al. 10). Similarly, Liao and Fu (2013) found that people will naturally “preferentially select information that reinforce their existing attitudes” (2359). Although relatively new, Borgesius’ work has created a new direction of research that sees Pariser’s “concerns about algorithmic filter bubbles” as potentially “exaggerated” (Haim et al 1). However, in Haim et al.’s study their focus was on Google News, a far cry from the complicated and ever-changing algorithms of Facebook.
Hence, there is a justifiable concern of the filter bubble limiting users’ exposure to information on the internet that could challenge or expand their worldview, but also of unawareness about the extent their choice is being filtered, which is where we situate our research (Pariser). This lack of transparency of “pre-selected personalisation” leaves users oblivious to the algorithms which thus shape what they see online and unaware of lack of diverse content (Borgesius et al. 3).
Relevance
We find there is limited academic literature proposing real life solutions for making the filter bubble transparent. Regarding the personalisation process and the way Facebook pre-selects content, we propose a new solution to reveal this to users. Personalisation and tailored content can come at the expense of our autonomous choice to see things beyond our bubble (Pariser).
To compare to old media methods of receiving news, political biases in newspapers are usually discoverable; for instance, most newspapers in the UK can be allocated specific political leanings by their readership (Smith). On Facebook however, it is harder for users to determine political biases of news stories. We would like to offer a way to make algorithmic or self-selected biases transparent to create awareness of the bubbles people are in.
We have also found existing ‘filter bubble bursting’ extensions (1, 2) are limited to the American political system (Bozdag and Hoven), and we would like to propose an alternative that analyses Facebook news stories more broadly than just a categorisation into ‘Republican’ and ‘Democrat’. Therefore, we offer an insight into why each news post has shown up in a user’s Newsfeed. We believe this is especially relevant with Facebook’s shift from a chronological Newsfeed to filtering by “top stories” (Luckerson).
Methodology
The methodology chosen for our research is a discursive interface analysis, guided by the work of Stanfill (2014) to understand affordances of websites. We aim to observe the perceivable components of Facebook’s interface that could partly reveal the platform’s algorithmic workings. There are two important factors to take into account: firstly, none of the actors’ actions are pre-definite as these can vary among people and over time. Secondly, as Grint and Woolgar argue, an interface can be written with a purpose while being read with a very different one (Hutchby 445).
Analysing an interface, and the way users interact with it, means looking into what the interface affords to users (Hartson 319). Hence, it involves questioning what the affordances of the interface offer to the users regarding different aspects of the user experience. There are four kinds of affordances: cognitive, physical, sensory and functional (Hartson 319). For the purpose of this research, we focus on functional affordances which add a purpose to an action; clicking on a link does not happen just because it is possible, but because there is an intention justifying this action (Hartson, 319).
These distinctions are necessary within the framework of a discursive analysis since it is aimed at observing the presupposed uses of a website, along with the actual ones. In practical terms, we will be looking at the main interface of Facebook and the Newsfeed preferences. We will try to understand the different elements involved in why a post is presented in a user’s feed. During our analysis, we will give meaning to our findings according to the framework of Pariser (2011) and Borgesius (2016). Moreover, the TechCrunch article titled “How the Facebook Newsfeed Works” will serve us as model to analyse the various possibilities and dynamics at play in the Newsfeed.
Facebook Interface Analysis
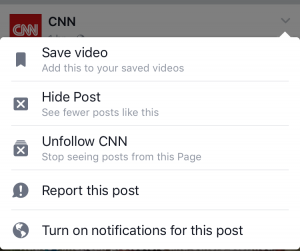
Fig.1. Facebook’s post visibility options
Homepage
The Facebook homepage, centered in the middle of the page, mainly revolves around the user’s Newsfeed which prioritises content to what the users “like, comment on, share, click, and spend time reading” (Constine). The Facebook Ticker is placed on the right side, small in size and less prominent. Here, Facebook provides real-time activity of the user’s Friends, in contrast with the Newsfeeds algorithmic priorities.
Also available on the homepage is the post visibility options (fig.1) which allows the user to control the Newsfeed. This is accessed by clicking on the arrow at the top right corner of every post. Through this “explicit tool”, Facebook affords the user the ability to say what they want and do not want to see (Constine). Similarly, Facebook allows the Newsfeed to be controlled through the Newsfeed button options; ‘Top Stories’, ‘Most Recent’, ‘Edit Preferences’ ( fig.2).
Newsfeed Preferences
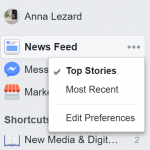
Fig.2. Facebook’s Newsfeed button
When the user clicks on ‘Edit Preferences’, five sections appear on the screen (fig.3). These five preferences enable users to customise their Newsfeed. The first offers the user to choose their most visible friends. The second, inversely, allows them to hide activities of specific friends. The third section makes it possible to reconnect with previously hidden friends. The fourth section permits the user to find out about new pages that might interest them according to their preferences. The last section offers more options such as seeing apps hidden from the feed.
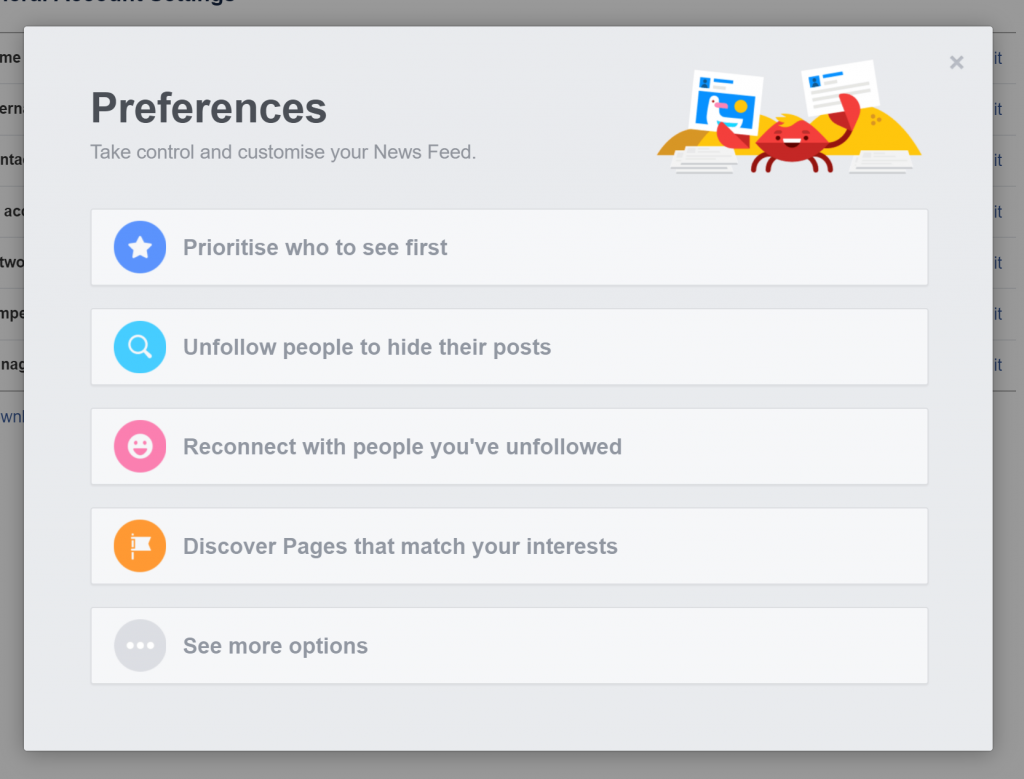
Fig.3. Facebook’s Newsfeed preferences.
Discussion
Looking at these functions and their affordances, there are a few things we can assume in relation to how Facebook filters and pre-selects posts on one’s Newsfeed. Following Pariser and Borgesius, we understand and see the filter bubble as a combination of personalisation by both human; self-selection, and non-human algorithms; algorithmic pre-selection personalisation.
Firstly, we have noticed that Facebook gives the impression that users can ‘control’ their Newsfeed preferences, this is stated in their Newsfeed preferences section: “Take control and customize your Newsfeed (fig.3). However, looking at what is actually being shown on the newsfeed, we have reason to believe that Facebook still pre-selects and filters the user’s newsfeed. For example, we found that when adjusting the Newsfeed to ‘most recent’ instead of ‘top stories’, Facebook still selects which of the ‘most recent’ posts to show to us. As Constine explains, there is such a large amount of content with only limited available space in the Newsfeed, so even ‘most recent’ posts are algorithmically selected. Further, the option to see ‘fewer posts like this’ (fig.1) points to a categorisation process of posts that is unknowable to the user.
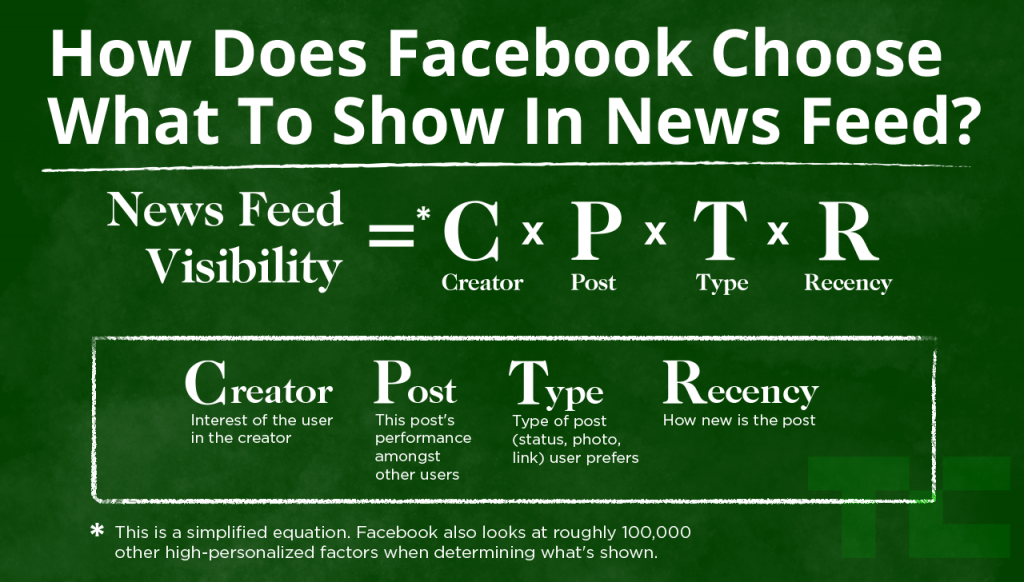
Fig.4. TechCrunch’s simplified equation for Facebook’s personalised Newsfeed (Constine)
We came across several factors which seem to influence the algorithmic selection process. Our findings are in line with an article by Constine (2016) denoting the factors that make up our Newsfeed content: Creator, Post, Type and Recency (fig.4). This is one simple understanding of how posts are pre-selected by Facebook. Though users have explicit options to self-select their content, we find that there is always an implicit undercurrent of Facebook’s algorithmic pre-selection. Agreeing with the concept found in Pariser’s literature, this shows evidence of the presence of a filter bubble.
Our intervention
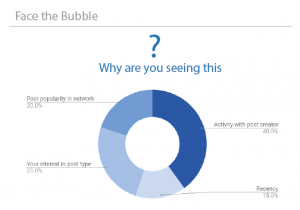
Fig.5. Face the Bubble Facebook extension.
As shown, Facebook gives us options to personalise our Newsfeed, but they are limited. Although the factors involved in algorithmic pre-selection of posts are still not completely clear in the front-end of the interface, we have seen evidence that this process occurs.
Following these findings, we would like to clarify the filtering process on a post-by-post basis to users. In doing so, we aim to provide users with more transparency and therefore increase autonomy with understanding of their Newsfeed.
The extension we propose is downloadable for all internet browsers to be used on Facebook. On the user’s Newsfeed, a small question mark is displayed next to each post (fig.6). The user can hover over this question mark and a box will pop up showing a pie chart displaying the four factors identified by Constine. We understand that these factors are not mutually exclusive, and will be differently relevant for different posts, so we thought a pie chart would be a good way of visually representing the things involved in post selection while still showing they are all at play together.
We chose an extension versus a separate app or website because it can be fully integrated into the Newsfeed and used in real time. As Bozdag and Hoven found, the previous filter bubble interventions were “not widely known” when used away from Facebook and other major social media sites (262).
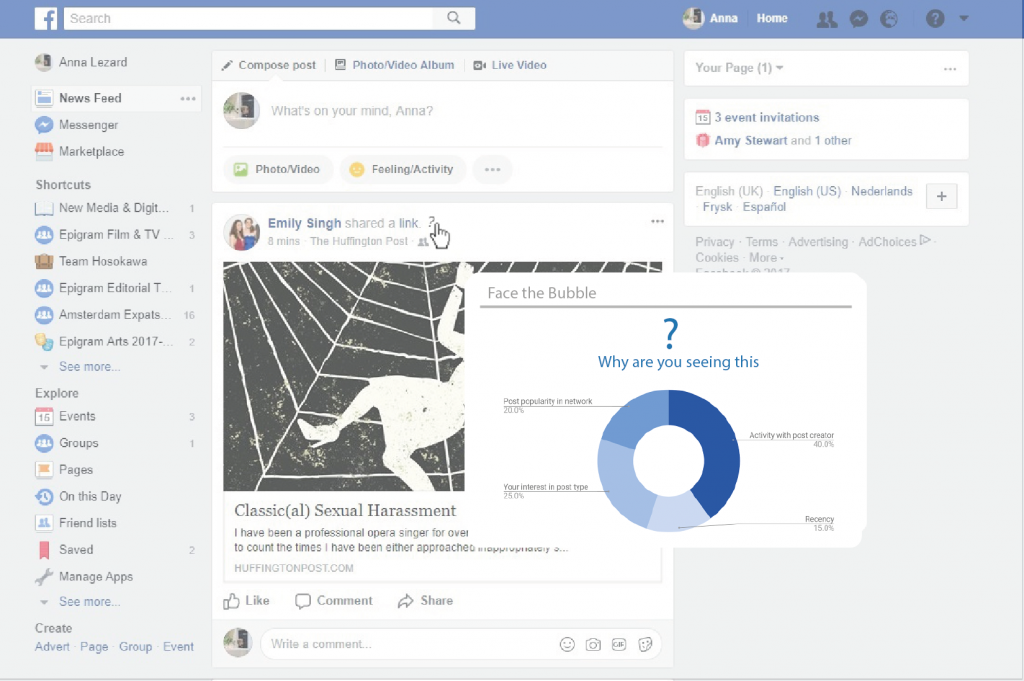
Fig.6. Our extension visualised on a Facebook interface.
Limitations
Our greatest limitation during our research was our inability to fully understand Facebook algorithms, mainly due to the inaccessibility of the back-end of the platform. This affected our interpretation of how the pre-selection algorithms work and how to translate this onto our extension. Another limitation is our lack in ability to actually create a real prototype of our extension, hence why we have proposed a hypothetical extensions with a suggested outcome in percentages, without actually created it.
Conclusion
In completing this project, we have found that the processes of self-selecting and the algorithmic pre-selection of content on the Facebook Newsfeed are not mutually exclusive. Although users have options to ‘control’ what they see, Facebook still creates a filtered Newsfeed. We have attempted show how transparency of the filter bubble can be achieved by designing an extension for users that reveals Facebook’s personalisation algorithm. We have shown that creating transparency affects users’ autonomy.
Karis Mimms, Nadine Linn, Anna Lezard, Elodie Mugrefya
References
Borgesius, Zuiderveen, et al. Should We Worry About Filter Bubbles? SSRN Scholarly Paper, ID 2758126, Social Science Research Network, 2 Apr. 2016.
Bozdag, Engin, and Jeroen van den Hoven. “Breaking the Filter Bubble: Democracy and Design.” Ethics and Information Technology vol.17, no.4, 2015. 249–65.
Constine, Josh. “How Facebook Newsfeed Works.” TechCrunch, http://social.techcrunch.com/2016/09/06/ultimate-guide-to-the-news-feed/ . Accessed 23 Oct. 2017.
Foer, Franklin. “Facebook’s War on Free Will.” The Guardian, 19 Sept. 2017. http://www.theguardian.com/technology/2017/sep/19/facebooks-war-on-free-will. Accessed 17 Oct. 2017.
Garimella, Kiran. Quantifying and Bursting the Online Filter Bubble. Cambridge, United Kingdom;NY, USA:ACM, 2017. 837–837.
Gitlin, Todd. “Public Spheres or Public Sphericules.” In Media, Ritual and Identity, edited by Tamar Liebes and James Curran, 1998. 168–174. London: Routledge.
Haim, Mario.; Graefe, Andreas.; Brosius, Hans-Bernd. “Burst of the Filter Bubble?: Effects of personalization on the diversity of Google News” Digital Journalism. 2017. 1–14.
Hartson, Rex. “Cognitive, Physical, Sensory, and Functional Affordances in Interaction Design.” Behaviour & Information Technology, vol. 22, no.5, 1 Sept. 2003. 315-338.
Hutchby, Ian. “Technologies, Texts and Affordances.” Sociology, vol. 35, no.2, 2001. 441-456.
Liao, Q., and Wai-Tat Fu. Beyond the Filter Bubble: Interactive Effects of Perceived Threat and Topic Involvement on Selective Exposure to Information. ACM, 2013. 2359–2368.
Luckerson, Victor. “Here’s How Your Facebook Newsfeed Actually Works.” Time 19 July 2015. http://time.com/collection-post/3950525/facebook-news-feed-algorithm/ . Accessed 21 Oct. 2017.
Maccatrozzo, Valentina. “Burst the Filter Bubble: Using Semantic Web to Enable Serendipity.” The Semantic Web – ISWC 2012, Springer, Berlin, Heidelberg, 2012. 391–98.
Nguyen, Tien T., et al. “Exploring the Filter Bubble: The Effect of Using Recommender Systems on Content Diversity.” Proceedings of the 23rd International Conference on World Wide Web, ACM, 2014. 677–686.
Pariser, Eli. The Filter Bubble: What The Internet Is Hiding From You. Penguin UK, 2011.
Resnick, Paul, et al. “Bursting Your (Filter) Bubble: Strategies for Promoting Diverse Exposure.” Proceedings of the 2013 Conference on Computer Supported Cooperative Work Companion, ACM, 2013. 95–100.
Schaap, Jorrit. “Book Review: The Filter Bubble by Eli Pariser.” Masters of Media, 17 Sept. 2011, https://mastersofmedia.hum.uva.nl/blog/2011/09/17/book-review-the-filter-bubble-by-eli-pariser/. Accessed 16 October 2017.
Smith, Matthew. How Left or Right-Wing Are Britain’s Newspapers? The Times. 6 Mar. 2017. https://www.thetimes.co.uk/article/how-left-or-right-wing-are-britain-s-newspapers-8vmlr27tm. Accessed 18 Oct. 2017.
Stanfill, Mel. “The Interface as Discourse: the Production of Norms through Web Design.” New Media & Society, vol. 17, no.7, 2015, 1059-1074.
Sunstein, Cass R. Republic. Com 2. 0. Princeton; Ewing: Princeton University Press California Princeton, 2009.