Empowered by Data: Streaming Platforms Becoming Media Distributors
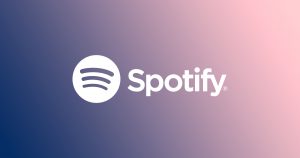
Fig. 1. Logo: Spotify. “Image.” Ixquick, 18 Oct 2018.
Introduction
Media streaming platforms have transformed traditional media industries and continue to do so. Netflix successfully started their own in-house production company based on insights from their user data, which has led the the company to save a lot of money recently. It seems likely now that the potential of data might empower other platforms that deal with different media types as well. Recently, the music streaming platform Spotify has aroused attention by announcing a direct upload option for artists. This means that music can bypass the institutions of record labels and distributors on its way to the listener. The music industry now fears the possibility of Spotify cutting out distribution companies from the value chain. With moves like this, big platforms are able to force whole industries to change their business models. It seems like their location at the site of the listener and their access to user data are essential for this. This paper seeks to investigate the role of big data in the current trend of media streaming platforms that are moving up the distribution chain.
Spotify has established itself as a successful platform with high popularity and a large user base, effectively allowing it to cut the formalities of the music industry’s distribution process (Shepard). This means that the platform is not as dependent on record labels and their distributors to receive music from artists anymore, and is starting instantiate features for direct contact with artists. What empowers Spotify to aspire to become its own media distributor? Does their data silo give them special insights?
In an increasingly diversified platform world it seems that platform-specific content might be the key to a sustainable business. Disney movies are expected to be taken off of Netflix in 2019, as Disney is creating its own exclusive streaming service (Hruska). In 2018, Spotify formally announced that they were going to change their business model, rebrand themselves and give artists access to upload music to the platform directly and for free. By June 2018, 200,000 artists had taken advantage of the new feature (Ingham). This might force other players in the industry to adapt themselves to the changed circumstances of music distribution. We seek to shed light on the particular properties of Spotify as a data-driven platform that allow it to push this transformation forward.
Context
The music industry has seen some significant changes in the past decade, brought on by new technologies. After illegal piracy struck the industry heavily around the millennium, it took a while to introduce digital distribution platforms such as iTunes that could reduce the losses and bring the music industry to the digital age. However, music streaming has established itself as the dominant mode of consumption nowadays, which is again a fundamental paradigm shift.
According to Patrik Wikström, the music world has traditionally operated with the ownership model, meaning that music is sold materially for the public to “own” and store in their personal collections (7). However, digital media platforms have marked a shift towards new distribution models, as seen in digital retailers like iTunes and later in streaming platforms like Spotify. With these developments, Wikström identifies the access model and the context model, respectively, as new dominant modes of music consumption. Consumers get access to music without actually owning either physical or virtual copies. Hence, music is not owned anymore, it is accessed. According to the Global Music Report, there was a 41.1% growth in streaming revenue in 2017 alone, and streaming revenues made up for 54% percent of the entire recorded music market (Global Music Report).
However, Wikstrom (7) hypothesized that it is not enough for platforms to merely operate via access models anymore if they wish to distinguish themselves from other platforms. As a result, Spotify and other music services have adopted the context model. User data is being captured and used for specific features that allow users to “do things” with music, which thereby affects the distribution process and the creative production of new music (Wikstrom 7). The context model makes use of user data in order to generate a listening experience that is extremely personalized. In doing so, it is assumed that the more precisely a music service can predict a listener’s preferences, the more time and money the listener will spend on the platform (Prey 2).
Objective
The relevance of our study lies in the investigation of how data can lead to transformations of traditional distribution models, with regard to the music industry. We focus on Spotify in this research, even though it is likely that findings may be applied to other media streaming platforms as well. As Spotify recently opened the artist submission feature and summoned up discussions on the future of music distribution, it is becoming evident that the platform has a massive transformative potential for the music industry.
How is this direct relationship to the artists beneficial to the platform and the artists, though? Spotify claims that by eliminating redundant parts of the distribution chain, less of the royalties are shared with these, meaning the artist themselves get to keep more of their money and be in direct contact with the platform that offers their music. Our objective is to investigate how this move is economically advantageous, how the platform’s data infrastructure plays a key role in this, and how and if a general idea can be derived about how the power of data empowers streaming platforms to become distributors themselves. From this we can distinguish how the anticipatory transformation affects the rest of the music industry.
Methodology
Spotify’s recent decision to open a direct distribution option for artists indicated a move towards taking over efforts of the traditional music distribution company. This led us to questioning how the streaming platform as a massive data-capturing enterprise can have a strategic advantage over third-party music distributors, especially when it comes to business.
To answer this, we will first have to review the relationships in the music industry between artists, labels, music distributors and streaming platforms. We will focus on Spotify in this research, even though we investigate a development that is likely to affect other platforms as well. For this purpose, we will rely on PR material from the music industry association IFPI and educational material from The Music Maze.
Since we want to research an ongoing development in the music business, we will conduct literature reviews about current transformations in the distribution chain that are brought about by larger technological and social trends. This involves a short review of how the music industry has changed over the last 20 years due to the impact of digital media.
We will turn to Spotify and identify its data-related and context-related features. To analyze these, we will make use of online research and academic literature by Prey. Points of interest are the datafication model, the algorithmic recommender system and the importance of playlists on Spotify. The capture model of surveillance by Agre will help us understand this data-driven application in a media theory context. We will then use data collected from Spotify to see how their algorithm functions in order to optimise it and collect additional data from it. This additional data with help us create a comprehensive data bank, that will be used to educate anyone who chooses, about how Spotify works, and how data collection can further improve their personal experiences with the platform. Finally, we will evaluate an outlook for the future of data-driven music distribution.
Analysis
Streaming platforms have become the single largest revenue source for the music industry and the main access point for music listening to many people. Music labels and distributors work closely with Spotify and other platforms to ensure this model’s success. Record labels or independent artists usually employ distribution companies to get their music featured in market catalogs. The streaming platform has largely replaced the record store here. Royalties generated from sales, or in this case user streams, are then channeled back to the distributors who take a percentage and pass the rest on the record label, which in turn pays the artist(s). These concepts are represented in the diagram below.
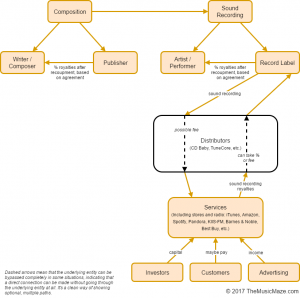
Fig. 2. The music industry: Shephard, Isaac. “How does the music industry work? Give me the high-level overview!” The Music Maze, 15 October 2018.
The revenue streams through the distributor entity is marked as optional in this diagram. Labels and artists do not need to work with distributors to get their royalties from the services, yet they do so because it is efficient. The development we are researching in this paper happens right here: Spotify opened a direct channel to the artists and laid the foundation for them to bypass distributors and possibly record labels.
To put this development in a theoretical context, we refer back to Patrik Wikström’s typology of distribution models, where he argues that the ownership model has been almost entirely succeeded by the access model, providing a temporary solution for the music industry (11). In the end, however, it will be the context model that wins (Wikström 11). Contextual features like sharing, organizing, remixing, and other third-party-services will soon generate the most economic value in music distribution. Wikström attributes the success of Spotify to the early adoption of such contextual features (12).
Let us take a look at the inner workings of Spotify now, as its data-driven and contextual features are what constitute its power. The platform employs a vast data-driven analytics framework to predict its users’ music taste and recommend songs to them. This is what Prey calls the “datafication of listening” (3). Every detail of a song and of a user’s listening behavior becomes valuable data used for music recommendations, targeted advertisements and the organization of curated formats, such as playlists.
Creating and sharing playlists are powerful contextual features, and placements in the platform’s editorial playlists are highly sought-after promotion tools (Fortune). Top playlists can have many thousands or millions of followers and placement in these guarantees lots of streams – and revenues. The curators of these, in-house or external, can leverage the exposure of a song dramatically. Further important contextual features are Discover Weekly and Release Radar. These algorithmically composed personalized curations are based on the user’s taste profile. According to Prey, over 40 million listeners have turned to Discover Weekly in 2015, when it was first introduced, making it one of Spotify’s most successful products (12). Furthermore, the algorithm automatically queues up recommended songs based on the user’s quantified taste profile when his current queue comes to an end.
The point of all this is: the huge amounts of data the platform’s algorithms gather and use for predictions determine the exposure that songs and artists get. It is therefore obvious that new songs that are similar in the terms of the algorithm to popular songs are likely to be recommended by it. This comes down to more exposure and more royalties in the end.
We could, therefore, argue that the algorithm friendliness of a song might have an impact on its success. Indeed, multiple accounts of songs that have been observed to gain popularity for the simple fact that they were queued up in recommendations on streaming platforms can be found online (Shepard).
From the perspective of Spotify aspiring to cut out distributors it is thus fair to assume that the lords of the algorithm have exact insights into what properties make songs algorithm-friendly, and furthermore have an economic interest in not sharing these algorithmic gold mines with third parties. Data that is accumulated from millions of users can be reverse engineered to create a process that can further streamline how artists and their followers interact. It can also help users understand how their data is used – visually.
Much about the music industry has to do with speculation, and creating a data bank for easy access to a data cache is a good way to provide insight into an industry that is at a crossroads. A sustainable path of profit lies in the platform’s editorial hegemony over the top playlists, into which they might place their in-house signings with preference to guarantee exposure. When analyzing the metrics of these popular playlists, they could easily derive a blueprint for an artist to fit in there – and start looking for a match to sign a direct distribution deal with.
The DataSpot
We envision The DataSpot to be similar to a resource posted by Facebook, that lets you see how they use algorithms and data. The DataSpot will be an app provided under the Spotify banner, and released by the Spotify website. It will have login features that can be used by users, artists, students, teachers, industry professionals, and others – you just need a social media account. Presented below is a mockup of how we envision the data screens to be viewed.
On the application’s interface, we envision the start page to present a new way of looking at distribution within the music industry. By clicking any part of the infographic, a user will be given access to data collected with that particular header in mind. When they choose a topic, they will be led to a series of visually interpreted data screens, with an option to download data files if they would like to create their own data sets. Users can provide feedback on what they would like to be featured by The DataSpot, and the data processed each week (along with an explanation or opinion by The DataSpot) will reflect relevant trends and shifts within the music industry.
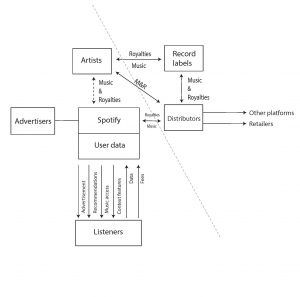
Fig. 3. Infographic. “The music industry and Spotify’s influence” 14 October 2018.
This platform can also help Spotify engage with the industry around them, and could be a source of raw data that could power their algorithms, while also keeping them fresh and emergent within the market.
A new market, a new way of music?
For the last few years several critics have called on Spotify to change their business model, which they felt was stale, outdated, and exploitative. Spotify has since responded with a move that could change the landscape of the music industry and the way that money flows through the industry. This change could lead to more personalized access to music that would serve to create a unique listening experience for fans. For artists, however, it could predict a way to earn more money from their original content, and a better way of navigating through the industry. Spotify’s decision to invest with Distrokid, a service that helps musicians upload and sell their music through online platforms, is further proof that the future of the music industry could be in the hands of the artists, and not music labels.
In closing, we conclude that streaming platforms can reshape digital music distribution through strategic use of contextual features, datafication, and algorithmic recommendations that raise the incentive for the music industry to work data-driven in the future. As the industry continues to evolve and our ways of listening broaden, we will see similar changes across the board. Datafication, machine-based learning, and algorithmic functionality have taken over in a music industry that has become significantly more computerized. Therefore, the DataSpot is a perfectly positioned tool to provide an abundance of information regarding these changes to anyone who may be interested.
References
Agre, Philip E. “Surveillance and Capture: Two Models of Privacy.” The Information Society, vol. 10, no.
2, 1994, pp. 101–127. Informa UK Limited, doi:10.1080/01972243.1994.9960162.
Arcand, Rob. “Is Lo-Fi House the First Genre of the Algorithm Age?” Thump, VICE, 30 Mar. 2017,
thump.vice.com/en_us/article/yp9e5j/lo-fi-house-youtube-related-video-algorithm-essay.
Bratton, Benjamin H. The Stack – On Software and Sovereignty. MIT Press, 2016.
Chavanne, David. “Generalized Trust, Need for Cognitive Closure, and the Perceived
Acceptability of Personal Data Collection.” Games, vol. 9, no. 2, 2018, p. 18. MDPI AG, doi:10.3390/g9020018.
Drott, Eric A. “Music as a Technology of Surveillance.” Journal of the Society for American
Music, vol. 12, no. 3, Oct. 2018, pp. 233–267. Journal of the Society for American Music,
doi:10.1017/s1752196318000196.
Hruska, Joel. “Disney’s Streaming Service Will Cost Less Than Netflix, Carry Less Content.”
ExtremeTech, 28 Aug. 2018,
www.extremetech.com/extreme/276195-disneys-streaming-service-wil
L-cost-less-than-netflix-carry-less-content.
IFPI. Org. Global Music Report 2018 ANNUAL STATE OF THE INDUSTRY. IFPI, 2018,
www.ifpi.org/downloads/GMR2018.pdf.
Ingham, Tim. “Spotify Opens the Floodgates: Artists Can Now Upload Tracks Direct to the Streaming
Platform for FREE.” Music Business Worldwide, 20 Sept. 2018,
www.musicbusinessworldwide.com/spotify-opens-the-floodgates-users-can-now-upload-tracks-to
-the-streaming-platform-for-free/.
“Ixquick.” Ixquick, ixquick-proxy.com/do/spg/show_picture.pl?
l=english&rais=1&oiu=https://ne
wsroom.spotify.com/static/img/story-background-5.jpg&sp=dbfa9e371753221bf2c4c
89105d1d1f0.
Jacobson, Kurt, et al. “Music Personalization at Spotify.” Proceedings of the 10th ACM Conference on
Recommender Systems – RecSys 16, 2016, doi:10.1145/2959100.2959120.
Madrigal, Alexis C. “How Netflix Reverse Engineered Hollywood.” The Atlantic, Atlantic Media
Company, 11 Dec. 2015,
www.theatlantic.com/technology/archive/2014/01/how-netflix-reverse-engineered-hollywood/282
679/.
Orcan Intelligence. “How Netflix Uses Big Data – The Startup – Medium.” Medium, The Startup, 12 Jan.
2018, medium.com/swlh/how-netflix-uses-big-data-20b5419c1edf.
Petruska, Karen, and John Vanderhoef. “TV that Watches You: Data Collection and the Connected Living
Room.” Spectator – the University of Southern California Journal of Film and
Television,vol.34,no.2,2014,pp.33-42.ProQuest,
http://proxy.uba.uva.nl:2048/docview/1616427303?accountid=14615.
Prey, Robert. “Knowing Me, Knowing You: Datafication On Music Streaming Platforms”. Musik und Big
Data, 2018.
Prey, Robert. “Musica Analytica: The Datafication of Listening”. Networked Music Cultures,
2016, pp. 1-21.
Shepard, Isaac. “How Does the Music Industry Work? Give Me the High-Level Overview!” The Music
Maze, The Music Maze, 13 July 2018,
www.themusicmaze.com/music-industry-work-give-high-level-overview/.
Skelton, Eric. “Youtube’s Algorithms Keep Recommending This Song, And It’s A Hidden Gem.”
Pigeonsandplanes, 2018,
pigeonsandplanes.com/discover/2017/10/boy-pablo-everytime-youtube-algorithm-interview.
“The Murky Business of Spotify ‘Playlist Pitching’.” Fortune,
fortune.com/2018/08/10/spotify-playlist-pitching-curators/.
Welch, Chris. “Spotify Is Trying to Lure Artists into Licensing Their Music Directly.” The Verge,
TheVerge,6 June 2018,
www.theverge.com/2018/6/6/17435070/spotify-artist-direct-licensing-advances-rumor.
White, Peter. “Netflix: Diving into the Data.” Broadcast, 2016, pp. 1-N_A. ProQuest,
http://proxy.uba.uva.nl:2048/docview/1922363524?accountid=14615.
Wikstöm, Patrik. “A Typology of Music Distribution Models”. International Journal of Music Business
Research, 2012, pp. 7-20.