AI Fact Checking: Artificial? Yes. Intelligent?
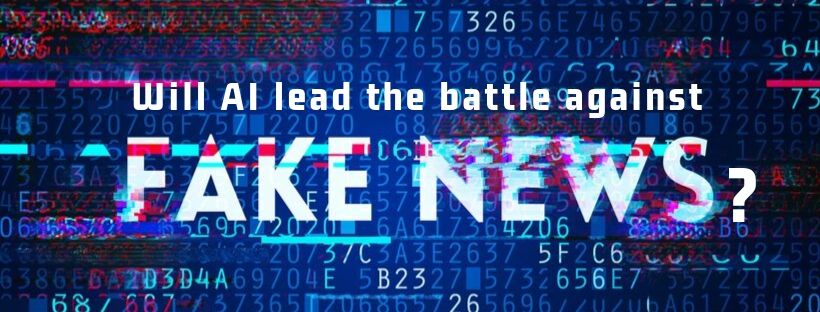
The phenomenon of ‘Fake news’ is now a household discussion, journalists and fact-checking platforms working solely to analyze and debunk these false pieces of information are now becoming increasingly visible. While it’s becoming harder than ever to tackle the seemingly unstoppable force of misinformation and disinformation peddled on social media, there is one ‘hope’ humanity is betting on, that is Artificial Intelligence (AI).
With all speculations around AI taking over the world for good/bad, a global discussion is beginning around ‘Automated Fact Checking’ to tackle the menace of fake news and foster the development of AI technology in newsrooms. Journalists and technologists are now working jointly to leverage AI techniques of machine learning, natural language processing, vision and speech recognition etc., to perform fact checks in a quicker and more-efficient manner.
On March 12, 2019, MIT’s Media Lab and Harvard’s Berkman Klein Center for Internet and Society announced their winners for ‘AI and the News Open Challenge’. A total of 7 winners from the field of technology and journalism who aimed to pioneer the study of how AI can be used to improve journalism were awarded a grant of $750,000. The list of winners included ‘Chequeado’, a fact checking organization based in Argentina who have long been at the forefront of using AI towards effective fact-checking on web. Just 2 months later, in the month of May, Chequeado along with United-Kingdom based Full Fact, Africa Check and Open Data Institute won $200,000 ‘Google AI Impact’ grant to bolster their work in the field of Automated Fact Checking. Facebook in a big move invested millions on building a machine learning model to flag potentially-fake images and videos on its platform, it also collaborated with several fact-checking platforms to test the possibilities of using AI to effectively tackle fake news.
So, why are research institutions like MIT and Harvard pouring millions of dollars on AI fact-checking? Why are tech giants like Google and Facebook investing huge capitals on AI fact-checking? Why are traditional media outlets and fact-checking platforms actively exploring the dimensions of AI to tackle fake news? the answer lies further below.
The current state of fact checking:
Almost every fact-checking platform, including the likes of FactChecker.org, PolitiFact, Snopes etc., follow a standardized form of steps to go about reaching the roots of false information. These fact checkers are highly proactive on social media and monitor platforms like Facebook and Twitter constantly in pursuit of a false piece of information to debunk. Once a potentially false piece of information (text/visual) has been picked for fact checking, these fact checkers do a thorough research and engage themselves in a systematic process to find out the truth behind the content. After the research, an evaluation of the findings is done and a fact-check report is written. This framework the platforms follow is also known as ‘Post-hoc’ fact checking.
But, in this age of social media, when content creation tools are at every layperson’s disposal, not only is fake news thriving more than ever but it can now spread faster than we could imagine. By the time a fact-checking platform goes about researching the information, the fake information might have already reached a sizeable population of netizens. Needless to say, the need of the hour is real-time fact checking and that’s where AI could lend a helping hand.
How can AI bolster swift and effective fact checking?
AI in its simplest understanding is a system that is programmed to mimic human intelligence and make autonomous decisions on given tasks. In the field of fact checking, an increasingly used approach to go about automatically detecting and debunking fake information is an intersection of 2 sub-fields of AI; namely, Natural Language Processing and Machine Learning. Here, besides programming computers to identify written and spoken words, computers are programmed to look beyond individual words and phrases to understand the context they are delivered in, this function is called Natural Language Processing: an expansive task where, with the help of algorithms and data, a computer learns what deceptive content looks like and in what patterns do they usually appear on web. Over the time, computers also learn from past tasks, past data and past outcomes to make better self-made decisions in the future, this aspect of AI, where a computer starts contextualizing information and understands the narratives around a specific topic to better the fact checking performance is called Machine Learning. The positives of AI are that computers can read-through large amounts of texts at a towering pace and can generate fact check reports in a much larger scale compared to human fact checkers.
For instance, ‘ClaimBuster’, the first end-to-end automated fact checking platform developed by computer scientist Chengkai Li and his team at the University of Texas, Arlington was trained on 20,000 genuine and fraudulent claims from the past US presidential debates to recognize and distinguish between facts, opinions and mere false statements. In a test during a US primary debate in 2016, more than 70% of actual claims checked by mainstream fact checking platforms were among the top statements identified by ClaimBuster too. This sheds light on how efficiently automated fact checkers can perform at a given task.
Other than fact checking, AI can do a lot more to journalism:
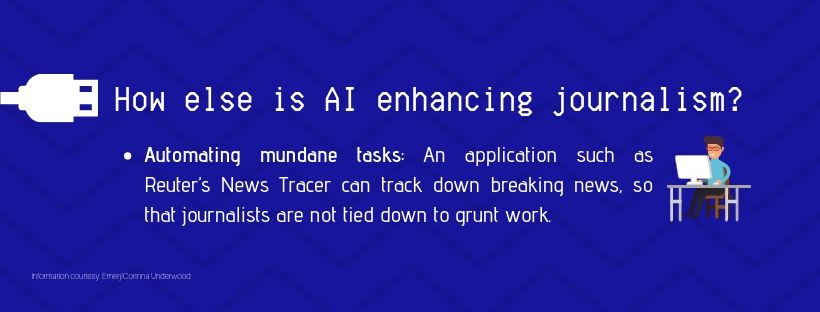
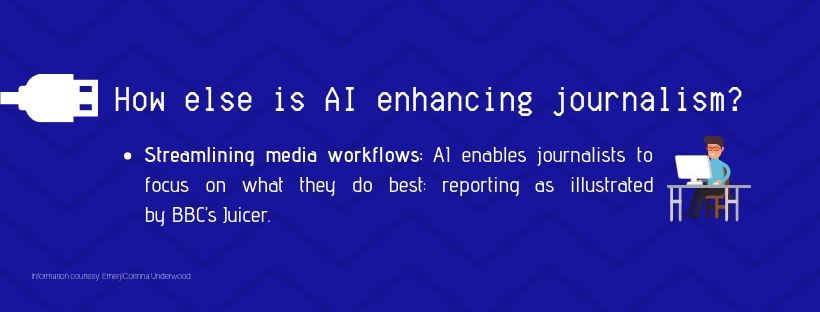
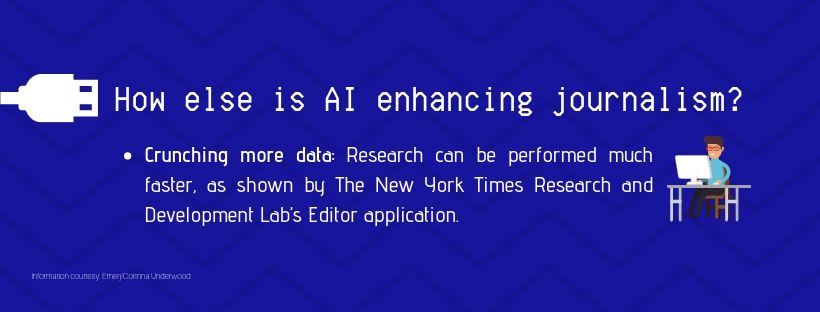
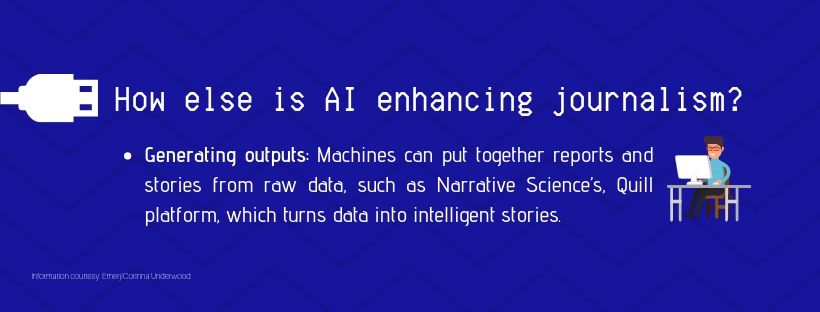
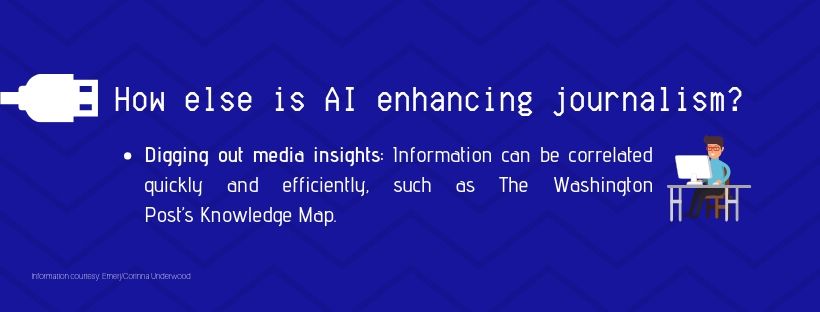
However,
Setting aside all the trust and faith research institutions and tech giants have posed in AI and automated fact checking, there are still a considerable number of researchers who are sceptic about AI’s efficiency. Dr Mark Klein, principal research scientist at MIT believes “Computers still seem to be very rudimentary in terms of being able to ‘understand’ what people say. They (people) do not follow grammatical rules very rigorously. They leave a lot of stuff out and rely on shared context. They are ambiguous or they make mistakes that other people can figure out. There’s a whole list of things like irony that are completely flummoxing computers now.”
Adding to the never-ending academic debate of ‘Humans versus Computers’, Dr Klein’s statement reiterates the ongoing ambiguity around the efficiency of AI. On the other hand, the investments made in the field of AI by researchers and tech-giants indicate otherwise. While it’s uncertain how closer can machines come in understanding the context and nuance while verifying information, what’s certain is that AI’s potential benefits make it a promising investment and a possible agent for real-time fact checking.
Bibliography:
Allcott, Hunt, and Matthew Gentzkow. “Social Media and Fake News in the 2016 Election.” JOURNAL OF ECONOMIC PERSPECTIVES, doi:DOI: 10.1257/jep.31.2.211.
Anderson, CW. “Towards a Sociology of Computational and Algorithmic Journalism.” New Media & Society – SAGE, 10 Dec. 2012, doi:https://journals.sagepub.com/doi/10.1177/1461444812465137.
Bucher, Tiana, and Anne Helmond. “The Affordances of Social Media Platforms.” The Sage Handbook of Social Media.
Bucher, Tiana. “‘Machines Don’t Have Instincts’: Articulating the Computational in Journalism.” 14 Jan. 2016, doi:https://doi.org/10.1177/1461444815624182.
Chua, Yvonne T. “Staying True to Journalistic Principles in an Era of Alternative Facts.” 4 May 2018, doi:https://doi.org/10.1080/01296612.2017.1455593.
Gillespie, Tarleton. “The Relevance of Algorithms.” Mit Press.
Konstantinovskiy, Lev, et al. “Towards Automated Factchecking: Developing an Annotation Schema and Benchmark for Consistent Automated Claim Detection.” ARXIV, 21 Sept. 2018.
Lim, Chloe. “Checking How Fact-Checkers Check.” Research and Politics – SAGE, 19 July 2018, doi:https://doi.org/10.1177/2053168018786848.
Thorne, James, and Andreas Valchos. “Utomated Fact Checking: Task Formulations, Methods and Future Directions.” Computation & Language, 20 June 2018, https://arxiv.org/abs/1806.07687.