CAN MUSIIO BURST THE SPOTIFYBUBBLE?
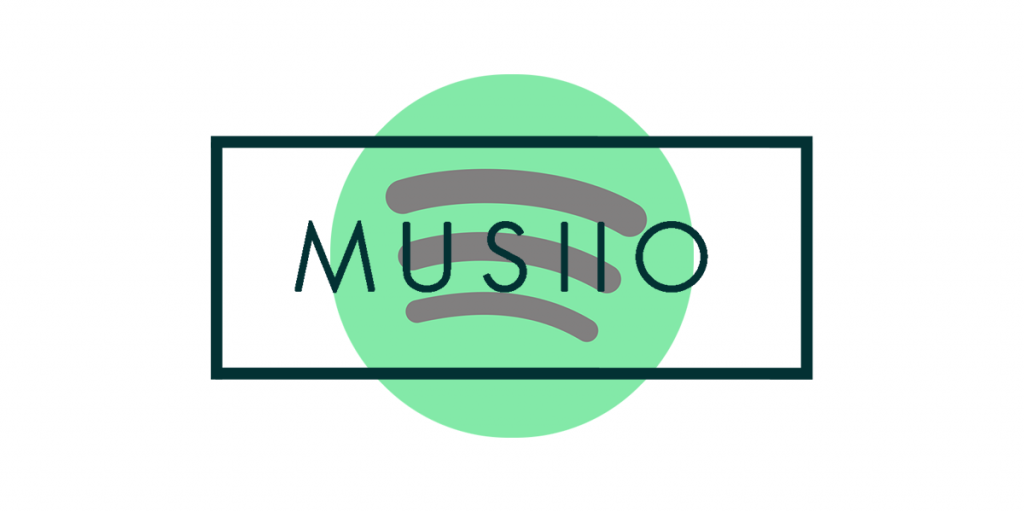
“When you own discovery, you own so much of the ecosystem (…)” Paul Vogel [1]
In the ever-changing music industry offering the best discovery tools is key. A small start-up from Singapore called Musiio could be a serious rival to big player Spotify.
The paradigm shift from music ownership to music streaming and the consequences for music production, distribution and reception have deeply changed our relationship to music. The problem of diminishing physicality and the associated change in the handling of music entails positive aspects such as increased mobility and diversity. On the other hand, this positive must also be understood as negative if one considers the excessive demands on users dealing with the flood of titles and possibilities available (Sinclair & Tinson 1-9).
What we listen to has been altered as selection processes have been automated and personalised due to growing music catalogues making it increasingly difficult for listeners to discover the music they love. Therefore, algorithmic support processes have been developed in the field of music information retrieval, i.e. the administration and sorting of music titles. In addition to automatic genre classifications or the identification of artists through an audio signal (e.g. Shazam), Spotify’s recommendation engines are particularly worth mentioning, as to a large amount of Spotify subscribers.
Recommender engines are mostly assigned to the discipline of artificial intelligence because they rely on adaptive algorithms. Their purpose is to relieve the user of the task of estimating the value of an object that was previously unknown to him or that he has not yet evaluated. In this way, recommender engines can be understood as tools, which allow the user to create a personalised order of their collection (Hosanagar et al. 805-823). A distinction is made between collaborative filtering, content-based filtering and hybrid filtering systems (809). Regardless of the filter system used, all recommendation systems are dependent on receiving information from users to correctly assess their preferences and create more precise evaluations.
To increase navigation and discovery in Spotify’s 30 million-title big library, the music service purchased Echo Nest in 2014, a database that has collected and analysed extensive data on user behaviour during music consumption. Information on how long songs are played and if shared is included. Using this data, Spotify has developed several discovery features, the most popular being the Discover Weekly playlist. 40 million people use this playlist worldwide. It can only be accessed if the user has been a Spotify subscriber for two weeks. Spotify creates an algorithmically curated, personalised playlist via the subscriber’s usage behaviour and played songs. This playlist contains 30 titles and is automatically deleted and recreated every Monday of a week.
The Spotifybubble
“By definition, a world constructed from the familiar is a world in which there’s nothing to learn” Eli Pariser (2011)
The variety of Spotify’s extensive algorithmic recommendation systems raises the question, whether algorithmic recommendation systems broaden the musical horizon or narrow it down by only reflecting the user’s preferences. Mike Mulligan assumes that Spotify users consume only 5% of available titles ( Knupper 1). In this context, the question arises whether users of these services move in musical filter bubbles and the stream only reflects what is already preferred. Spotify’s recommender engines have been broadly criticized due to their imprecise and misleading results.
Kohn (2014) discusses the aspect of social entropy in this context. He places random encounters with new content at the centre of his reasoning on diversity. He further argues that recommendation systems merely reflect user preferences at a certain moment in time. As a result, he constructs the preference bubble: „This is a bubble that represents what you currently prefer and will change (…) over time through a variety of ways. (…) As marketers get better at mining the preference bubble we have an opportunity to engage instead of obfuscating “ (1).
Bas Grasmayer (2017), a specialist for digital music distribution, identifies the filter bubble on Spotify as The Moby Problem. This problem does not concern the musician Moby, but it describes the operating modes of algorithmic recommendation on Spotify and the corresponding errors (1). After once listening to an old Moby song, his tracks started repeatedly appearing in Grasmayer’s Discover Weekly playlist even though he continually skipped them (1). He further discusses The Remix Problem, defining it as not only receiving recommendations of Moby titles but remixes of these titles.
This happens due to the parameter of title similarity being used as a foundation for the compilation of titles. Worst case, this can lead to users getting recommendations for ten different versions of the same title. The described problem shows us the necessity of equilibrium between predictive accuracy and diversity of proposed objects.
In contrast to Grasmayer, Bowles (2015) regards the Spotify recommendation cycle to be a novelty bubble. According to Bowles, the human brain presents a far more severe filter bubble than any algorithm. He hereby peruses the fact that humans cannot search for objects or, in this case, music titles they do not already know about, algorithms, however, possess those abilities (1).
Musiio
This is where the Singapore based start-up Musiio comes in. Their final goal is the reduction of inefficiencies of recommending systems by using Artificial Intelligence (AI). They claim that their AI model can „recognise thousands of features from every single audio track and categorise music with an accuracy greater than 90%“ (Sagar 1). Moving away from recommendations based on a user’s behaviour profile but rather focussing on actual traits of the audio will give music lovers an advanced search and discovery experience.
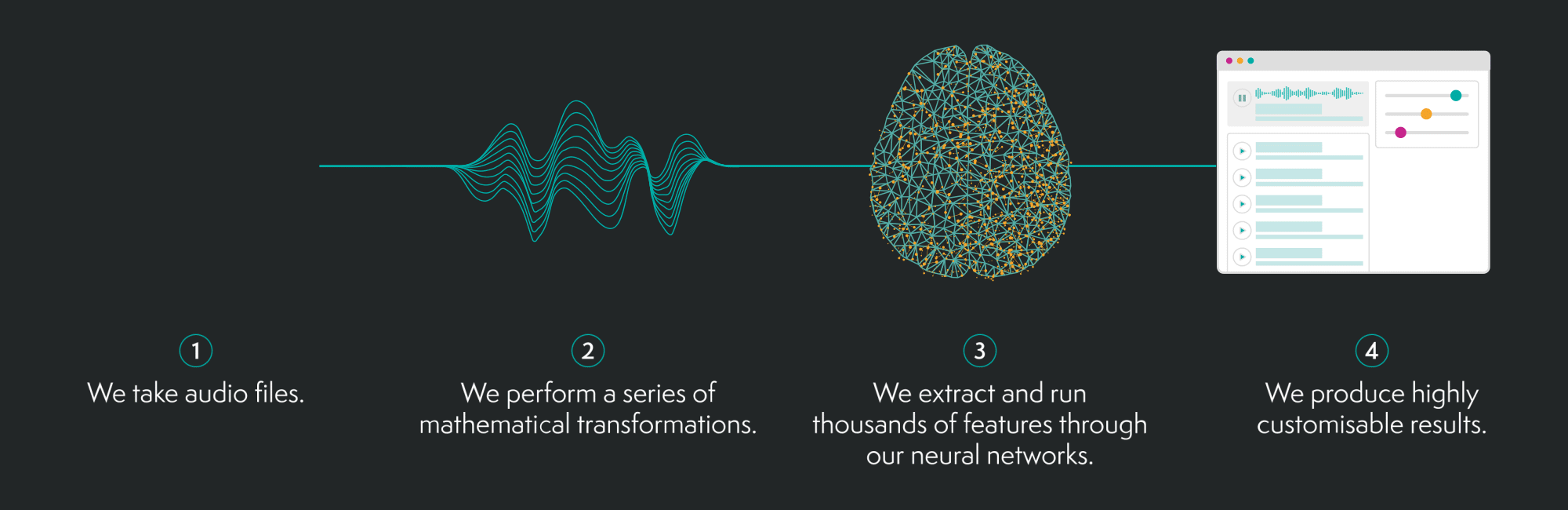
Their AI model can analyse and tag over 200,000 new tracks per day. Once ‘heard’ the technology can search through music at a rate of 1m+ tracks in two seconds. Furthermore, it can be custom-trained to work from a cold start. The percentages here represent the AI’s calculated feasibility of the tag.
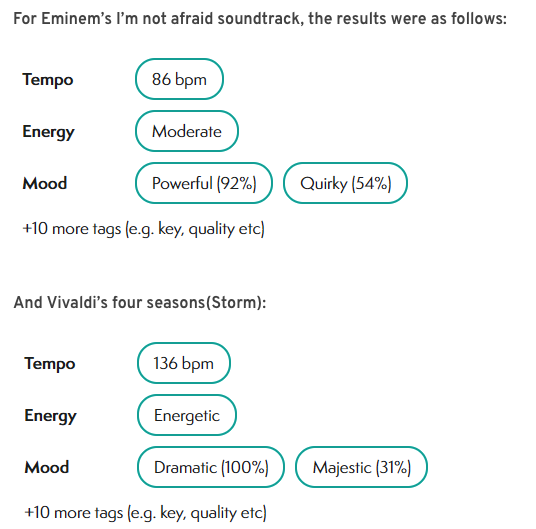
Musiio offers a real alternative to Spotify in the sense of increased accuracy when it comes to song recommendations. Nevertheless, the question in my opinion as a music lover will not necessarily concern the accuracy of recommendations but the inherent possibility for serendipity to prevent filter bubbles. Algorithmically calculated recommendations and the curation of creative content that goes with it miss one feature in particular – the human trait.
Kappes (2012) formulates this status quo as the absence of alternatives to filtering in order to participate in modern, digitized society, the only question is how these filters should work (4). Scientific work on whether recommendation systems can broaden the musical horizon at all and the question of what role music can play for identity formation in the age of its technical reproducibility must follow.
[1]This mission statement by Paul Vogel, Spotify’s Vice President and Head of Financial Planning & Analysis was revealed in an interview with Music Business Worldwide on the 17th of September 2019 (Stassen 1).
References
BOWLES,Carter. “Filter Bubble? Not Really. Try Novelty Bubble.“ Northcutt,https://northcutt.com/digital-marketing/filter-bubble-not-really-try-novelty-bubble/
GRASMAYER, Bas.“The Moby Problem: Open letter to Matt Ogle’s successor at Spotify.“ Musicxtechxfuture, https://northcutt.com/digital-marketing/filter-bubble-not-really-try-novelty-bubble/
HOSANAGAR, Kartik; Fleder, Daniel; Lee, Dokyun; Buja, Andreas. Will the Global Village Fracture into Tribes? Recommender Systems and their Effects on Consumer Fragmentation.Management Science,vol. 60, no. 4, 2014, p. 805-823.
KAPPES, Christoph. “Filter Bubble? Warum die Gefahren der Filter Bubble überschätzt werden.“Christophkappes, http://christophkappes.de/wp-content/uploads/downloads/2012/06/TZD_Kappes-Christoph_-Filter-Bubble.pdf
KNUPPER, Francisca. “Wie die Spotify-Algorithmen deinen Musikgeschmack verändern.“ Vice, https://www.vice.com/de/article/z4kvwj/wie-die-spotify-algorithmen-deinen-musikgeschmack-verandern
KOHN, AJ. “The Preference Bubble.“ Blindfiveyearold, http://www.blindfiveyearold.com/the-preference-bubble.
SAGAR, Ram. “Can Singapore Startup Musiio Rival Spotify With Its Push For Personalisation?“. Analyticsindimag,https://www.analyticsindiamag.com/can-singapore-startup-musiio-rival-spotify-with-its-push-for-personalisation/
SINCLAIR, Garry; TINSON, Julie. Psychological ownership and music streaming consumption.Journal of Business Research vol. 71, 2017 p. 1–9.
STASSEN, Murray.“Spotify: ‘Owning’ discovery of music will lead to improved gros
margin.“ Musicbusinessworldwide, https://www.musicbusinessworldwide.com/spotify-owning-discovery-of-music-will-lead-to-improved-gross-margin/
PARISER, Eli. The Filter Bubble: What the Internet is hiding from you. Penguin, 2011.