Discerning Familiarity in the Rise of Facial Recognition
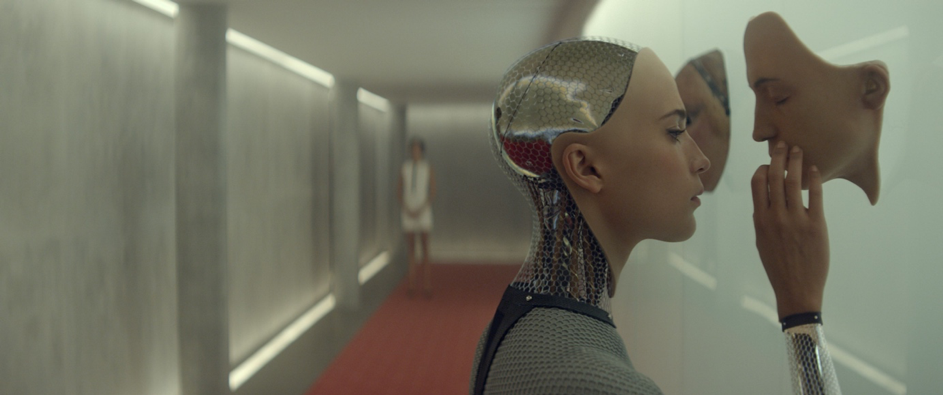
Facial recognition is ‘hot’: it has been in science fiction since the 1960’s, but nowadays biometric technologies – the technologies that recognize individuals based on biological aspects – are advancing and are increasingly being adapted by tech giants and governments, and hereby intervene in human daily life. Facial recognition technology is emerging on a spectrum from unlocking the newest iPhones to surveillance purposes and policing.
The algorithmic network behind facial recognition in electronic devices is provided through machine learning – which may not sound particularly new or surprising, as our current paradigm is dominated by big data. Billions of pictures from social networking sites are collected in databases in which the algorithmic network learns how to detect faces and eventually identify them, by going over pictures multiple times. Faces are captured and mapped through measuring geometric proportions, for example the distance between the eyes, nose and mouth. These proportions, or ‘faceprints’, are translated to vector – a string of numbers that uniquely identifies an individual, rendering body parts into binary codes (Sample 2019; Wevers 89; Liljeford & Lee-Morrison 70).
As facial recognition is increasingly favored, it is becoming more and more accurate – although multiple factors are at stake in the process of facial recognition that influence its accuracy. Conditions reasonably are ideal when pictures are bright and clear and faces are centered, however people typically take pictures in different settings, lightings, or positions. But, apart from contextual discrepancies, most importantly: human appearances are very extensive. Subsequently, algorithmic cycles are not free of failure, as these are primarily recognizing dominant groups of people and correspond to said individuals. Failure lies within algorithmic bias and lack of neutrality, from which marginalized groups suffer.
This type of standardization is what Max Liljeford and Lila Lee-Morrison connect to the concept of space of subjectivity, in which they propose that faces reduced to mappable surfaces and standardized shapes lose their depth, as these are obliged to “a web of immaterial statuses and obligations” (54). The general assumption of these standardized shapes, is that these are neutral, objective and value-free (Wevers 91). Identities always can be altered and judged through “masquerade, camouflage, mimicry and deception” (Liljeford & Lee-Morrison 72), and therefore biometric reduction reinforces unequal norms – as subjectivity lies in perception. Lisa Gitelman elaborates upon this debate in “Raw Data” Is an Oxymoron with an example of the race-classification in the era of apartheid in South-Africa by Geoffrey C. Bowker and Susan Leigh Star, specifying that “when phenomena are variously reduced to data, they are divided and classified, processes that work to obscure—or as if to obscure—ambiguity, conflict, and contradiction” (9). Data is always cooked, and never raw (2).
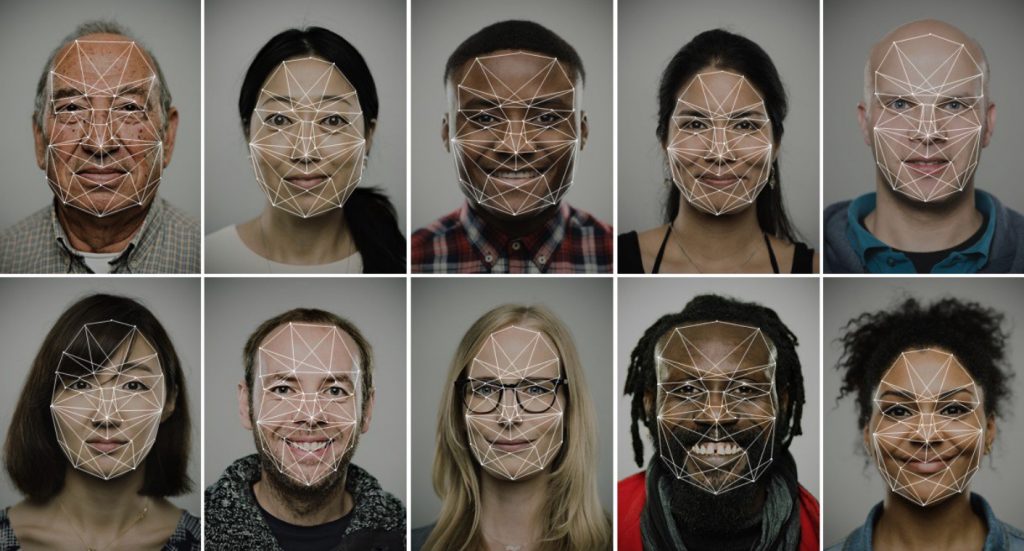
Various gender scholars have argued that technologies by default are white, male, cis and able (Magnet 50; West et al. 109; Ferrando 15; Richardson 51; Lee 57). An algorithmic system that is trained for white, male faces, will experience difficulty in recognizing otherwise and will be less accurate in detecting female faces or those from people of color. This underrepresentation leads to disproportional misidentification, as biometrics make them hypervisible, which results from “either a failure of the biometric machine, which is likely to mark subjects as potential threats, or from the use of biometrics for profiling” (Wevers 101).
One such example occurred in 2015, when Google Photos accidentally labeled black people as ‘gorillas’ (Simonite 2017). The deep neural network (which is built after the neural network in human brains) collects and categorizes pictures and labels these, and similarly to a human brain, the system gets smarter through undergoing errors and having these corrected. In 2017, researchers from the University of Virginia discovered that their algorithmic software contained gender bias predictions – a large set of images that were used to train the software with displayed sexist gender biases, for example in activities such as cooking and sports. Women would be more likely to be shopping or doing dishes, as men would be coaching and shooting (ibid.). According to the researchers, this gender bias could amplify other social biases such as race discrimination. In a more recent study from MIT Media Lab, researchers found that three different commercial cloud services for facial recognition worked better on light male faces (with an error rate of 0.3%), as opposed to dark female faces (with an error rate up until 35%) (ibid.). These exemplified errors are only superficial, but illustrate the ambiguous complexity of facial recognition technology, and that these therefore share core similarities with other technologies that work according to algorithmic logic.
Other than not being recognized and being excluded, as facial recognition software is being implemented not only by tech giants but also generally is commercialized and used for surveillance by governmental institutions, this could lead to serious implications when conclusions are drawn from digital systems without human verification – that is, when said systems have not been tested and improved. This issue cuts like a double-edged sword, as the input, design and programming of the algorithm is the result of human intervention, as Evgeny Morozov argues in his article The Tyranny of Algorithms: “algorithms don’t build their judgments on anything—their creators do” (2012). Misidentification could lead to wrongful accusations, limiting of freedom and invading of privacy. Therefore, algorithms should not be blindly applied – datasets should be reflecting real statistics, but foremostly be transparent and aware of potential bias.
Bibliography
Ex-Machina. Dir. Alex Garland. A24, Universal Pictures, 2014.
Ferrando, Francesca. “Is the Post-human A Post-woman? Cyborgs, Robots, Artificial Intelligence and the Futures of Gender: A Case Study”. Eur J Futures Res. 2:43. (2014): 1-17.
Gitelman, Lisa (ed.). “Introduction”. ‘Raw Data’ Is an Oxymoron. Cambridge: MIT Press. (2013): 1-14.
Lee, Jason. Sex Robots: Future of Desire. Cham: Springer International Publishing AG, 2017.
Liljeford, Max and Lee-Morrison, Lila. “Mapped Bodies: Notes on the Use of Biometrics in Geopolitical Contexts”. Socioeasthetics: Ambience – Imaginary. Social & Critical Theory:19. (2015): 53-72.
Morozov, Evgeny. “The Tyranny of Algorithms”. Wall Street Journal. 2012. Accessed 22 September 2019. <https://www.wsj.com/articles/SB10000872396390443686004577633491013088640>
Magnet, Soshana Amielle. When Biometrics Fail. Gender, Race, and the Technology of Identity. Durham, London: Duke University Press. (2011): 41-50.
Richardson, Kathleen. “Sex Robot Matters: Slavery, the Prostituted, and the Rights of Machines”. IEEE Technology and Society Magazine. 1932:4529. (2016): 46-53.
Sample, Ian. “What Is Facial Recognition – And How Sinister Is It?”. The Guardian. 2019. Accessed 22 September 2019. <https://www.theguardian.com/technology/2019/jul/29/what-is-facial-recognition-and-how-sinister-is-it>
Smith, Brad. “Facial Recognition: It’s Time for Action”. Microsoft. 2018. Accessed 22 September 2019. <https://blogs.microsoft.com/on-the-issues/2018/12/06/facial-recognition-its-time-for-action/>
Simonite, Tom. “Machines Taught by Photos Learn a Sexist View of Women”. Wired. 2017. Accessed 22 September 2019. <https://www.wired.com/story/machines-taught-by-photos-learn-a-sexist-view-of-women/>
Simonite, Tom. “Photo Algorithms ID White Men Fine—Black Women, Not So Much”. Wired. 2018. Accessed 22 September 2019. <https://www.wired.com/story/photo-algorithms-id-white-men-fineblack-women-not-so-much/>
West, Mark, Kraut, Rebecca and Chew, Han Ei. “I’d Blush If I Could: Closing Gender Divides in Digital Skills Through Education”. UNESCO. 2019. Accessed 23 May 2019. <https://unesdoc.unesco.org/ark:/48223/pf0000367416.page=1>
Wevers, Rosa. “Unmasking Biometrics’ Biases: Facing Gender, Race, Class and Ability in Biometric Data Collection”. Tijdschrift voor Mediageschiedenis. 21:2. (2018): 89-105.