ImageNet Roulette: This mean Sorting Hat is not magic, it’s how AI works
ImageNet Roulette is not just another selfie app. It shows us why it is deeply problematic to classify people through “AI” systems. The debate around ImageNet Roulette is urgently needed: Around the globe, machine learning systems are popping up in many crucial contexts to automatically make decisions about people.
“Look at this – what a shitty app!”, a friend texted me yesterday, followed by a screenshot of the web application ImageNet Roulette to which she had uploaded a picture of herself in a busy street of New York. Based on the photo, the app had classified my friend as “psycholinguist: a person (usually a psychologist but sometimes a linguist) who studies the psychological basis of human language”. To her bewilderment and anger, a random guy walking behind her in the photo was classified as “rape suspect”.
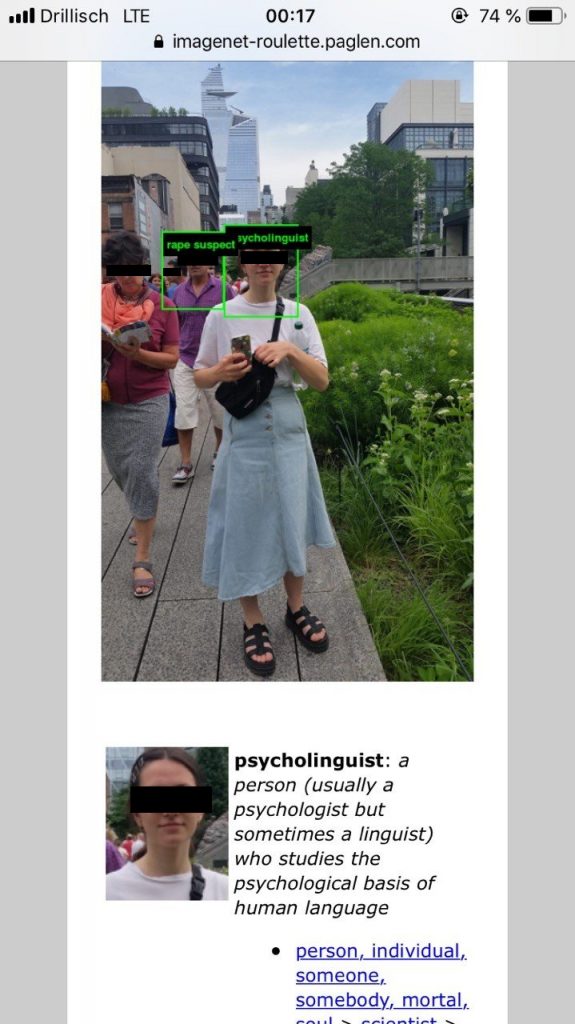
Courtesy of my friend.
ImageNet Roulette is an “AI”-based web application that went viral after its release a few days ago. It allows users to provide a picture (via upload, URL or their own webcam) which is then classified by the app according to a complex classification scheme. ImageNet Roulette has incited a huge debate because many people were sorted into discriminatory, misogynistic and racist categories.
Behind the project are researcher Kate Crawford and artist Trevor Paglen who study the implications of large datasets which are used to train a specific type of machine learning systems. Crawford and Paglen devised ImageNet Roulette to provoke people to think about the (inherent) problems that occur when an “AI” is classifying people. “Our hope was that we could spark in others the same sense of shock and dismay that we felt as we studied ImageNet and other benchmark datasets over the last two years”, they explain on the website.
How does ImageNet Roulette work?
Supervised machine-learning systems for facial recognition like ImageNet Roulette are fed with huge collections of images which have already been sorted into specific categories. After this “training” process (also referred to as deep learning), the artificial neural network can be used to classify pictures it has not processed before (Sudmann 10).
ImageNet Roulette is based on a dataset called ImageNet which has been widely used to train AI systems during the past 10 years (Crawford and Paglen). It consists of about 14 million images sorted into more than 20 thousand categories. The images were scraped from the web and then labeled by crowdworkers on Amazon’s Mechanical Turc platform (Crawford and Paglen). For their roulette, Kate Crawford and Trevor Paglen used all the pictures in the “person” category of this dataset, including its respective sub-categories.
ImageNet Roulette is yet another example (see also this, this or this) that clearly shows us some of the negative symptoms that arise when an “AI” is making judgments or predictions about people. But it only shows us the tip of the iceberg. To understand what is going on – or better, what is going wrong – we have to dig deep.
Many layers, many possible causes for bias
Seen through the lens of Actor-Network-Theory, machine learning systems are embedded in a complex network of developers, institutions, sometimes crowdworkers, hardware components, pre-assumptions, legal frameworks, input data, algorithms, users and many more. In every phase of their development, people and technical materialities together influence the eventual outcomes of the system.
Thus, technical and social discrimination can be implanted at many different points: in the minds of the developers (just think about the recent revelations about tech’s entanglement with Jeffrey Epstein), in the dataset, in the functioning of the algorithm or even through the users who might misinterpret the results. This not only holds true for “computer vision” (the subfield of “AI” which includes object and face recognition) but also for other applications of machine learning and pattern recognition.
Crawford and Paglen digged deep into the datasets which is one level on which the seeds for negative, discriminatory outcomes can be planted. Their archeology of datasets explains in detail which problematic assumptions underlie the input data (more specifically, its taxonomy, categories and images). The whole article is available here.
Luckily, ImageNet Roulette respects the privacy of its users. According to the website, all pictures are immediately deleted after the classification. It does not further process the data that is generated by users. But similar systems are already in place worldwide. They do not only classify people but also base decisions on the classifications.
The dangers of derived data: Automated decisions
Technically, the labels attached to people by ImageNet Roulette are derived data (also called inferences). Derived data “are produced through additional processing or analysis of captured data” (Kitchin 6). In this sense, the photos that people upload to ImageNet Roulette (input) are captured data whereas the resulting classifications (output) can be considered derived data.
Researchers Sandra Wachter and Brent Mittelstadt argue that we need a right that protects us from unjust and abstruse derivations like the ones produced by ImageNet Roulette. The problem is that “inferences draw on highly diverse and feature-rich data of unpredictable value, and create new opportunities for discriminatory, biased, and invasive decision-making.” (Wachter and Mittelstadt 1) The whole point of automatically classifying people based on their data is often to automatically make decisions about them.
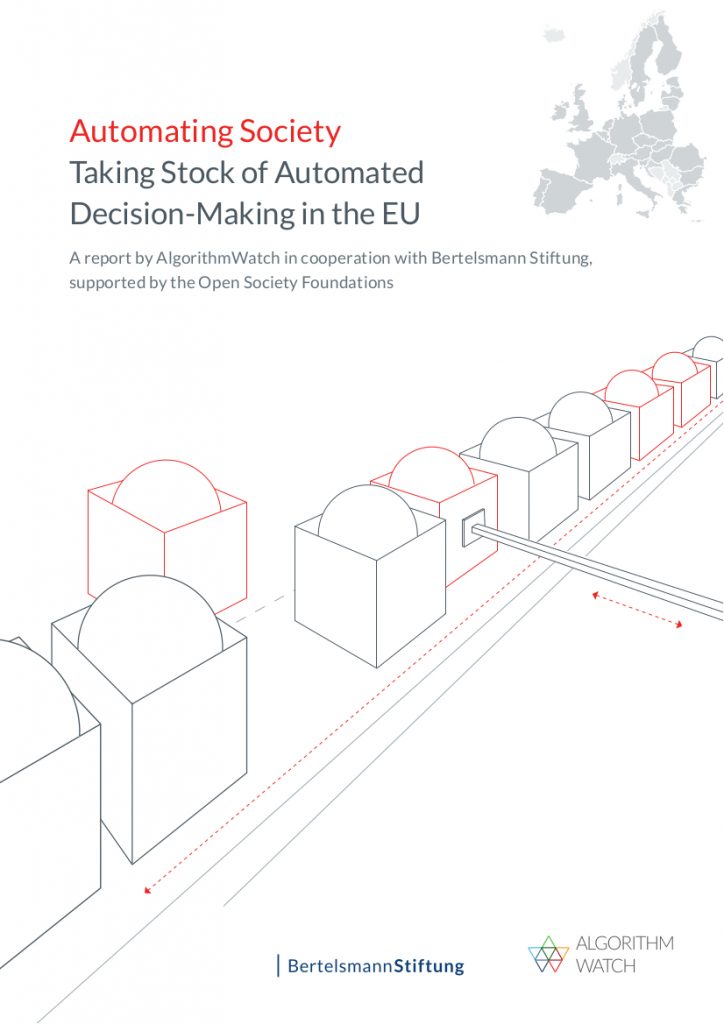
CC-BY 4.0 – AlgorithmWatch
Such automated decision-making systems based on derived data are already being used in many corporate and governmental settings worldwide. These systems, particularly machine learning systems (Apprich et al.), often mirror and amplify patterns of discrimination based on categories like class, race, gender and sexuality that exist in the culture from which they have emerged (Barocas and Selbst).
For example, research has shown that many systems are biased and discriminate against people of colour and lower-income communities in crucial areas such as risk-based sentencing (Angwin et al.), predictive policing (Ferguson) or predatory lending (Fisher; O’Neil). These systems are unjust, increase inequality and already have serious negative implications for people’s lives.
Bruno Latour famously wrote that “technology is society made durable” (Latour 1). Human assumptions, biases and political opinions are materialized in the technologies we build and use. But automated decision-making systems also actively make durable: They can reproduce and stabilize structural inequalities that traverse our societies. Virginia Eubanks therefore warns that these systems are “Automating Inequality”.
Not a ‘shitty’ app after all?
In the end, I was quickly able to convince my friend that ImageNet Roulette is not a ‘shitty’ app after all. It is a critical intervention pointing to the complexity and problems of machine learning systems which have become immensely influential in many people’s lives. It succeeded in sparking a public debate where it is urgently needed.
References
Angwin, Julia, et al. Machine Bias: There’s Software Used across the Country to Predict Future Criminals. And It’s Biased against Blacks. 23 May 2016, https://www.propublica.org/article/machine-bias-risk-assessments-in-criminal-sentencing.
Apprich, Clemens, et al., editors. Pattern Discrimination. meson press, 2018.
Barocas, Solon, and Selbst, Andrew D. ‘Big Data’s Disparate Impact’. California Law Review, vol. 104, no. 3, 2016, pp. 671-732.
Crawford, Kate, and Trevor Paglen. Excavating AI: The Politics of Images in Machine Learning Training Sets. www.excavating.ai.
Eubanks, Virginia. Automating Inequality: How High-Tech Tools Profile, Police and Punish the Poor. St. Martin’s Press, 2018.
Ferguson, Andrew G. The Rise of Big Data Policing: Surveillance, Race, and the Future of Law Enforcement. New York University Press, 2017.
Fisher, Linda. ‘Target Marketing of Subprime Loans’. Journal of Law and Policy, vol. 18, no. 1, 2009, pp. 121-155.
Kitchin, Rob. The Data Revolution: Big Data, Open Data, Data Infrastructures & Their Consequences. Sage Publications, 2014.
Latour, Bruno. ‘Technology is Society Made Durable’. A Sociology of Monsters: Essays on Power, Technology, and Domination, edited by John Law, 1991, pp. 103-132.
O’Neil, Cathy. Weapons of Math Destruction: How Big Data Increases Inequality and Threatens Democracy. Crown, 2016.
Sudmann, Andreas. “Zur Einführung: Medien, Infrastrukturen und Technologien des maschinellen Lernens.” Machine Learning – Medien, Infrastrukturen und Technologien der Künstlichen Intelligenz, edited by Christoph Engemann and Andreas Sudmann, transcript Verlag, 2018, pp. 9-36.
Wachter, Sandra, and Brent Mittelstadt. ‘A Right to Reasonable Inferences: Re-Thinking Data Protection Law in the Age of Big Data and AI’. Columbia Business Law Review, no. 1, 2019, https://papers.ssrn.com/sol3/papers.cfm?abstract_id=3248829.
Credits for the Sorting Hat preview picture go to Chad Sparkes. The image size was altered for the purpose of this article — CC BY 2.0