Old Town Road to Success: How Music Becomes Popular on Spotify
Jasper van der Heide – jasper.vanderheide@uva.nl
Tamar Hellinga – tamarhellinga@gmail.com
Rimmert Sijtsma – sijtsma.rimmert@gmail.com
Ziyi Liu – ziyiliu.rachel@gmail.com
Spotify has become an established phenomenon in our daily routines. How do songs become successful on the platform? Follow us as we explore the (Old Town) road to success.
Introduction
Spotify, since its launch in 2008, has become an established phenomenon. It’s name and concept are used as framework for other platforms, like ‘The Spotify of Art’, ‘The Spotify for Books’ and ‘The Spotify of the Auto Industry’. The platform itself has also nestled itself into our routines; 44% of its users listen to music on Spotify daily. Besides, of the 217 million monthly active users, a hundred million are paying subscribers (Harris et al. 1).
No wonder there has been great interest in researching Spotify. Existing academic research attempts to explain the workings of Spotify, its economic implications and the business model it is built upon (Eriksson et al.; Marshall). Spotify is particularly interesting to investigate, because it can be seen “as a media company that operates at the intersection of advertising, technology, music, and finance” (Vonderau 1). Its versatility has also sparked mainstream media’s attention, for example on how streaming services have changed the release of songs, song title changes and decrease of song length.
Whereas academic research is more focused on the foundations of Spotify, mainstream media point out trends. In this blog post, we strive to bridge these practices. Our attention focuses on popular music on Spotify. To determine what popular music is on Spotify, we look at Charts, which are playlists composed by Spotify based on “a formula that protects against any artificial inflation of chart positions”. Specifically, we look at the Top 50 Global, which features songs that are streamed a lot, updated daily.
The underlying algorithmic structures of Spotify determine many elements on the platform: from discovering new music, artists or playlists to the endless stream of music on Spotify radio. But the algorithm is not the only factor accountable for popularity of music on Spotify. We are interested in the factors that contribute to music being successful on the streaming platform. To answer the question ‘How does music become popular on Spotify?’ we look at the following four factors: categorization, playlistification, song properties, and external factors.
Categorization
Categorization is a familiarity in the digital era, as computer algorithms are capable of creating categories of identity based on users’ web surfing habits, it could connect this understanding to music as well. Music categorization is an algorithmic process where music is split into different segments such as tempo, acoustics, energy and danceability according to similarity-based criteria (Höthker 65). Generally, genre definition and attribution is subjective work, which could lead to vague results as people’s relative knowledge about each music style will cause different answers, reducing the efficiency and accuracy of matching suitable to music with target audiences.
Machine learning techniques, on the other hand, are found to be successful in extracting trends and patterns from massive datasets. It is hard to tell if a song is happy or sad, but categorization could help us make sense of it, assigning them to similar categories regarding style or mood. The ability of extracting valuable information from musical audio data would benefit a number of application functions such as music retrieval and recommender systems (McKay 315), which constitutes the basis of personalized Spotify playlists like ‘Daily Mix’ and ‘Discover Weekly’.
Music categorization is similar to “user identity” categorization in the fact that both of them are continuous processes rather than one-off conclusions (Cheney-Lippold, 173). Gillespie regard algorithm as a credible information tool that can consolidate or reshape public discourse (191). Algorithmic categorization supplements the discursive understanding of music genres like hard rock by continuously collecting statistics to shape the musical definition of hard rock. Music genres are constantly evaluated and definitions might change according to the feedback data.
An example is Every Noise at Once, a massive genre map developed by Spotify data alchemist Glenn McDonald. When the site was established, McDonald thought 400 genres would be a lot. However, the site lists more than 1700 genres and is still growing (Johnston). Analyzing data over years contributes to identifying new ideas about music and could direct musicians working within popular genres. Where there is a cluster of listening, there is a genre, even if it does not have a name yet. Categorization enlightened us with a deeper understanding of how music styles come into fashion, exploring new types of music and unique cultures that create specific genres.
To sum up, categorization is an important factor for popularizing a song. For the user, categorization helps to match them to the genre that fits user habits. For the artist, various genres become useful sources to understand where they can fit in the overall music universe, where there potentially is a valuable context for inspiration. For streaming media like Spotify, categorization is the cornerstone of linking customers to music.
Playlistification
Our experience with music has become increasingly personalized. Spotify invested heavily in affording its users a tailored experience. For example, Spotify’s ‘radio’ function automatically aggregates a stream of songs based on the original song that started the radio. The company also creates playlists curated specifically for users, based on previous behavior within the platform (Prey 12). Here, we can see hints of the underlying logic of Spotify.
Spotify’s strategy to invest in personalizing users’ music experience seems to pay off. Its ‘Discover Weekly’ playlist, “a personally tailored playlist of 30 new tracks that is delivered to each subscriber every Monday morning” (Prey 12), has over 40 million listeners and is considered one of its most successful products. In an age of abundance of media content, it seems inevitable that platforms guide users through the vast array of content by personalizing each interface to the specific user. Spotify’s ‘Taste Profile’ is capitalising on this idea by showing users curated playlists specifically for each unique user.
Spotify’s feedback loop consists of allowing on-demand access, encouraging intensive use by users, capturing every move, personalizing experiences through algorithms, and feeding that experience back into on-demand access. Users’ ‘Taste Profile’ is a constant negotiation between user actions and the algorithm (Cheney-Lippold 177).
The feedback loop is not only of interest to users, however. It is argued that songwriters are testing the water to see which songs work and which do not (Forde). Driven by access to back-end analytics, artists increasingly focus on characteristics that seem to be picked up by the algorithm of Spotify.
The monetary gains from featuring on a popular playlist are substantial. Being featured on the playlist ‘Today’s Top Hits’ is worth almost 20 million extra streams, which translates to roughly $150,000 in extra revenue from Spotify alone (Aguiar & Waldvogel). If being featured on playlists matters, then this understanding of how Spotify curates these playlists is vital to streaming success.
Song properties
Besides Spotify’s features, we also looked at some characteristics of popular songs. Firstly, we analyzed length of songs. We compared the average song length in a year in the Billboard Top 100 with the length of songs in the Top 50 Global on Spotify on the 22nd of October 2019. We were unable to scrape the Billboard information from their website, so had to rely on a non-official source. We then coupled the Billboard with the MusicBrainz database, an encyclopedia for music, to get the length of songs. The information of the Spotify songs were obtained through their API. Source code can be found here on Github.
The data shows that the length of songs in the Billboard top 100 rose until the 1990’s (presumably because of the introduction of the CD), but has since been decreasing. Songs in the Spotify Top 50 Global have the same average, but there is great variation in song length (Fig.1).
This decline in the average song length is sometimes attributed to Spotify for two possible reasons. Firstly, Spotify pays an artist after their song has been played by a users for at least 30 seconds, with the amount of monetary compensation received not dependant on the length of the song. Thus, an artist receives as much money for a two minute song as for a six minute song. Secondly, the attention span of people has been declining, and Spotify makes it really easy to skip a song (McKinney). But since the decline started way before Spotify, which was launched in 2018, even existed.
Gauvin had similar findings regarding the length of intros to a song, both the time before the first voice in a song appears and the time before the title of the song is mentioned. This can also be related to the aforementioned explanations. He also researched whether songs with shorter intros get more popular than songs with longer intros, but found no evidence.
We also examined some other features provided by the Spotify API, like Energy, Tempo, Valence and Danceability. Again using the Billboard top 100 set, this time looking up the song through the Spotify API and obtaining the features. This process was not always possible, because not all songs are on Spotify, and there is great inconsistency in the names of songs and artists.
We see an increase over time in energy and danceability, and a decrease in valence, but no differences between Billboard and Spotify (Fig. 2). Spotify also provides the distribution of these features for all songs on its platform. We then see that songs in the Global Top 50 have a higher danceability, and lower energy than the overall distribution of songs in Spotify.
To conclude, popular music has changed over time, possibly supported by Spotify’s affordances. Songs have gotten shorter, more danceable and more energetic. Popular songs are also more danceable and less energetic than non-popular songs. There is nevertheless a lot of variance, so these features are not really predictors of popularity.
External factors
There is more to success on Spotify than the streaming platform itself. Not only does social influence within the platform play a role, where songs with a high amount of streams are streamed even more due to users’ assumptions about quality (Salganik et al. qtd. by Suh 8). In fact, the popular music on Spotify could also be seen as a reflection of popularity offline and on other online platforms. External events influence how often songs are streamed (Harris et al. 2). Events for example are the release of music videos accompanying song release, concerts, social media and featuring in a series. Maasø has identified this concept as “the eventization of listening, whereby outside events and happenings influence streaming patterns” (165).
In this section, we focus on external factors that contribute to success on Spotify. Firstly, it has been found beneficial to feature popular artists on a song (Suh 33). This way, multiple audiences can be reached at once (27). Secondly, the concept of eventization is relevant to popularity of songs (Maasø 154). This could mean artists performing at festivals, where new audiences can discover their music (165). Other events with influence on popularity are releasing music videos, where the buildup and aftermath lead to increased discussion regarding an artist (Greener et al. 12).
Artists can also use social media in order to create a fan base (Haynes and Marshall 1976). Through social media, more attention can be generated towards the release of songs. Songs being featured on apps like TikTok, where users create videos with music, can increase the likelihood of popularity. Besides, being used in a Netflix series and memefication can help popularize music. Old Town Road is a good example of the latter (Fig. 3), which quickly garnered interest on social media with the multitude of memes that users created (Harris et al. 2).
Lastly, the popularity of songs on Spotify could also be newsworthy for (online) media, which in turn generates more interest in the song and could lead to more streams on Spotify (Maasø 167). There is not just one external factor leading to success on Spotify. Rather, there are a multitude of methods and factors at interplay that increase the likelihood of streams on Spotify. Overall, we can conclude that popularity on Spotify reflects popularity across other platforms and events, both online and offline.
Conclusion
We set out to bridge popular and scientific literature regarding the factors categorization, playlistification, song properties and external factors, all of which presumably add to a song’s success on Spotify. This streaming platform has changed how users engage with music and the music industry’s look on producing music.
Categorization of music on Spotify works in twofold. For users, categorization matches music based on fluid genres to the real-time listening behavior of each user. For artists, data about categories on Spotify gives artists an impression of where they could fit in the musical landscape or inspire new genre-crossing songs. Categorization is thus seen as the cornerstone of Spotify’s playlists.
The playlistification of Spotify works through the categorization of music and the ‘taste profile’ of users. Being featured on playlists seems to be a necessity for success on the platform. It is not surprising to find that artists increasingly write their songs with playlists in mind.
We questioned how this search for success changes songs. First and foremost, songs are getting shorter. We cannot contribute this change solely to Spotify, however. Secondly, the intros of songs seem to get shorter. Lastly, songs have become more danceable and energetic. Due to the variance in the sample, we do not see these as predictors for song popularity.
We want to end this piece with a question to spark discussions: what do these findings mean for the future of music? There are both positive and negative outlooks. Certainly, the categorization of music on Spotify has given room for audiences to find their specific genre. If you are over the moon with ‘old school uk hip hop’ or ‘aggrotech’, Spotify will help you access these musical cavities in more depth. Moreover, Spotify is often credited with helping unknown artists make a name for themselves. This could hardly do any harm to music.
On the other hand, increasing algorithmic curation of music might lead to the increasing adaptation of music to fit this specific medium. Songs are getting shorter, albums are becoming less prominent due to the focus on playlists and the increasing analytical ingenuity of music producers might lead to a more uniform musical palette. If the focus of song release is success and getting featured on playlists, is that the future we want to listen to?
Creative intervention
Inspired by these developments, we have digitally produced a song destined to be successful on Spotify. As a creative intervention, its purpose is to question whether success – in this case in the shape of streams – should be at the center of creating music.
The song was produced with Alysia, an app that creates lyrics and melodies via artificial intelligence systems. In our case, we used our own lyrics (see appendix), based on Spotify’s Top 50 Global songs. Alongside the song, we created an album cover (Fig. 4), a tweet and Instagram stories post to garner interest around the song release (Fig. 5 and Fig. 6 respectively). Lastly, we created a few memes to spark our future audience to post about Old Town Road to Success (Fig 7., Fig. 8 and Fig. 9). The following is what was created when we let algorithms and established factors for success guide the process: Old Town Road to Success by Algorythm.
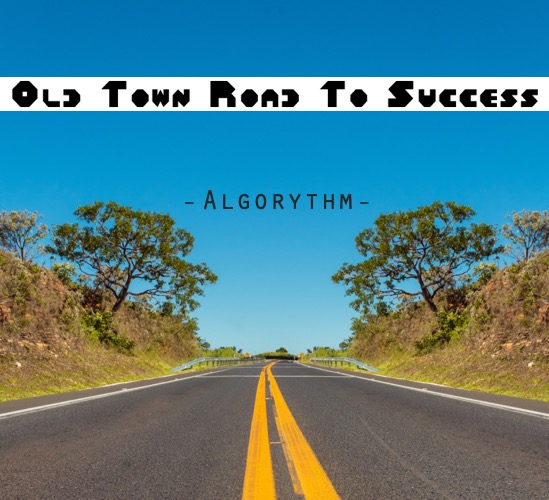
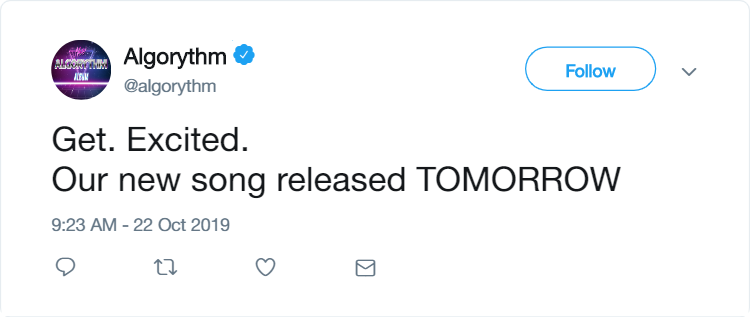
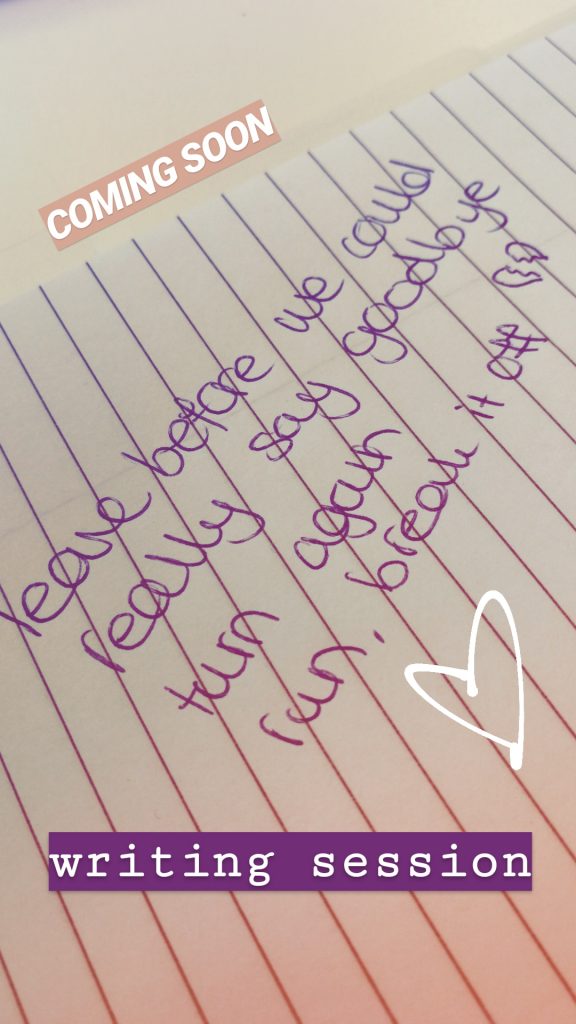
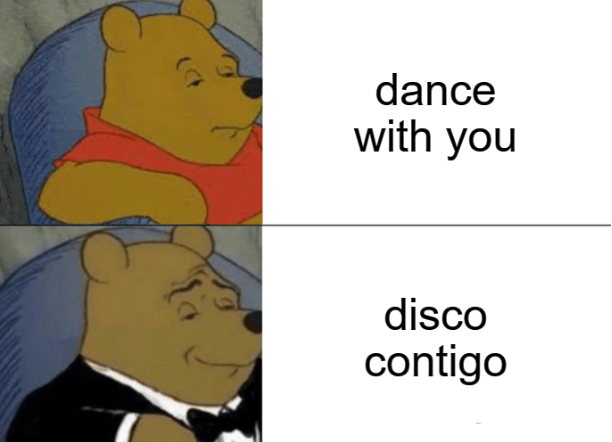
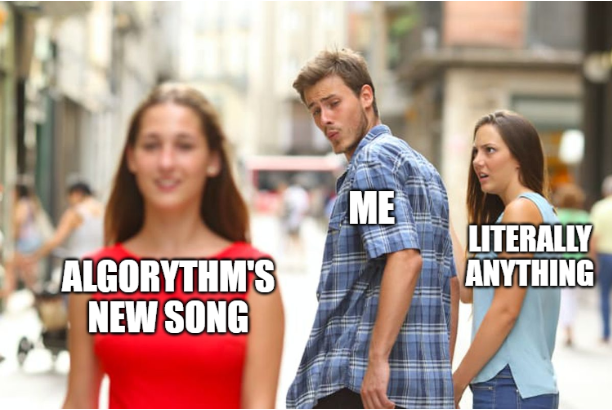
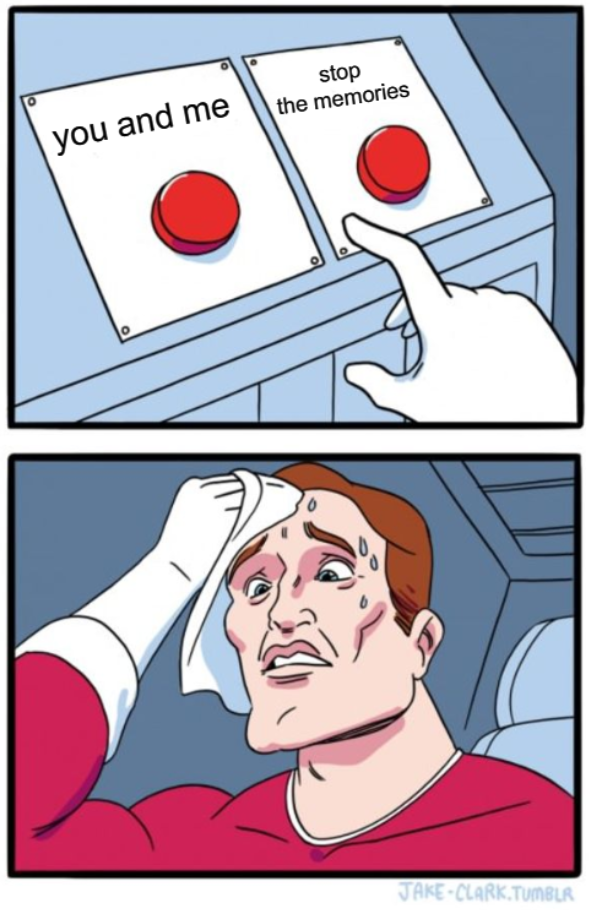
Bibliography
Aguiar, Luis, and Waldvogel, Joel. “Platforms, Promotion, and Product Discovery: Evidence from Spotify Playlists.” JRC Economy Working Paper, JRC Technical Reports, May 2018.
Cheney-Lippold, John. “A New Algorithmic Identity: Soft Biopolitics and the Modulation of Control.” Theory, Culture & Society, vol. 28, no. 6, 2011, pp. 164–181, doi: 10.1177/0263276411424420.
Eriksson, Maria et al. Spotify teardown: Inside the black box of streaming music. MIT Press, 2019.
Forde, Eamonn. “’They could destroy the album’: how Spotify’s playlists have changed music for ever.” The Guardian, 17 Aug. 2017, https://www.theguardian.com/music/2017/aug/17/they-could-destroy-the-album-how-spotify-playlists-have-changed-music-for-ever.
Gauvin, Hubert Léveillé. “Drawing Listener Attention in Popular Music: Testing Five Musical Features Arising from the Theory of Attention Economy.” Musicae Scientiae, vol. 22, no. 3, Sept. 2018, pp. 291-304, doi:10.1177/1029864917698010.
Gillespie, Tarleton. “The Relevance of Algorithms.” Media Technologies, The MIT Press, 2014, doi: 10.7551/mitpress/9780262525374.003.0009.
Greener, Guy, et al. “Electronic Word of Mouth and the Music Industry in the Age of Web 2.0.” SocArXiv, 11 Aug. 2019, doi: 10.31235/osf.io/7wqhd.
Harris, et al. “Analyzing the Spotify Top 200 Through a Point Process Lens.” arXiv, 9 Sep. 2019, p. 1-22.
Haynes, Jo, and Marshall, Lee. “Beats and tweets: Social media in the careers of independent musicians.” New Media & Society, vol. 20, no. 5, May 2018, pp. 1973–1993, doi:10.1177/1461444817711404.
Höthker et al. “Investigating the influence of representations and algorithms in music classification.” Computers and the Humanities, vol. 35, no. 1, Feb. 2001, pp. 65-79, doi: 10.1023/A:1002787826686.
Johnston, Maura. “How Spotify Discovers the Genres of Tomorrow.” Spotify for Artists, https://artists.spotify.com/blog/how-spotify-discovers-the-genres-of-tomorrow.
Maasø, Arnt. “Music Streaming, Festivals, and the Eventization of Music.” Popular Music and Society, vol. 41, no. 2, 2018, doi: 10.1080/03007766.2016.1231001.
Marshall, Lee. “‘Let’s keep music special. F— Spotify’: on-demand streaming and the controversy over artist royalties.”, Creative Industries Journal, vol. 8, no. 2, 2015, pp. 177-189, doi: 10.1080/17510694.2015.1096618.
McKay, Cory. Automatic music classification with jMIR. 2010. McGill University, thesis. http://citeseerx.ist.psu.edu/viewdoc/download?doi=10.1.1.165.2642&rep=rep1&type=pdf.
McKinney, Kelsey. “A Hit Song Is Usually 3 to 5 Minutes Long. Here’s Why.” Vox, 30 Jan. 2015, https://www.vox.com/2014/8/18/6003271/why-are-songs-3-minutes-long.
Prey, Robert. “Knowing Me, Knowing You: Datafication on Music Streaming Platforms.” Jahrbuch Für Musikwirtschafts- Und Musikkulturforschung Big Data Und Musik, 2019, pp. 9–21., doi:10.1007/978-3-658-21220-9_2.
Suh, Brendan J. International Music Preferences: An Analysis of the Determinants of Song Popularity on Spotify for the U.S., Norway, Taiwan, Ecuador, and Costa Rica. 2019. Claremont McKenna College, Bachelor thesis. CMC Senior Theses, scholarship.claremont.edu/cmc_theses/2271.
Vonderau, Patrick. “The Spotify Effect: Digital Distribution and Financial Growth.” Television & New Media, vol. 20, no. 1, Jan. 2019, pp. 3-19, doi: 10.1177/1527476417741200.
Appendix
[Intro]
Algorhythm, man
Baby, you know you want this
[First verse]
Your love is all I’ve got
What do I know, baby
It’s you and me
We go down like this
[Second verse]
Let me ride your heart
Take you with me
‘Cause you’re back now
Time can’t make ya see
[Third verse]
Can’t take me higher, bitch
Tell me you are gonna come through
[Pre-Chorus]
I’ve been through some shit, yeah
I’m good here too
[Chorus]
More loved, feelin’ loved
Leave before we could really say goodbye
Turn again, run, break it off
Then that’s it
You and me
Stop the memories
[Second verse]
Tryna put you out my mind
Time can’t heal the pain
Girl, don’t play games
Ooh let me love you
I don’t want to go down like this
I’ve got to call you beautiful more
[Pre-Chorus]
I’ve been through some shit, yeah
I’m good here too
[Chorus]
More loved, feelin’ loved
Leave before we could really say goodbye
Turn again, run, break it off
Then that’s it
You and me
Stop the memories
[Bridge]
Prr prr, lil’ bitch
Fuck this shit
Break it down like this
Don’t call me again
Tonight, I’m making friends myself
Leave me alone
Disco contigo
[Chorus]
More loved, feelin’ loved
Leave before we could really say goodbye
Turn again, run, break it off
Then that’s it
You and me
Stop the memories
[Outro]
Yeah, ooh ooh
La da da