AI comment moderation: Perspective API – a case study
With the increasing relevance of online social networking practices, forums of discussion have come to play a significant role in shaping the public opinion. Emerging from the panorama of Artificial intelligence employed to moderate online conversations is Perspective API, which rates the toxicity of the content pre-publication. This comes with advantages and disadvantages, improving empathy self-awareness on one hand, thus risking self-censorship on the other.
Introducing comment moderation
Nowadays, with the thriving of social networking platforms, the world wide web is easily associated to a stage, an arena, a digital forum where the population congregate to exert their free speech rights, adjourn and discuss newly agenda topics. In fact, in the economy of Information, discussion and opinion sharing among users is not only encouraged, but galvanized: citizens accessing open online discussion is at the core of the democratizing role of networked media within our digitalized society (Gibson, 2019). However, discussions are often subjected to disruption by malicious actors, contributing with online toxic comments, virtual harassment, hate speech and misinformation (Guberman, Schmitz & Hemphill, 2016; Obadimu, et co., 2019). According to recent reports, 73% of adult internet users witnessed online harassment, while more than 40 % have directly experienced it. Mostly problematic is, however, the 27% confessing to not be willing to comment and participate in discussion after witnessing harassment posted online, which could trigger a spiral of self silencing, a threat to the free expression of performative social interaction online (Adams, 2018; Obadimu et co., 2019). When left unaddressed without deterrents, verbal violence can freely result into actual, physical violence with irreversible consequences (Gibson, 2019).
Consequently, maintaining online forums a safe space is an imperative for functional confrontation (Gibson, 2019; Jain et. co, 2018).
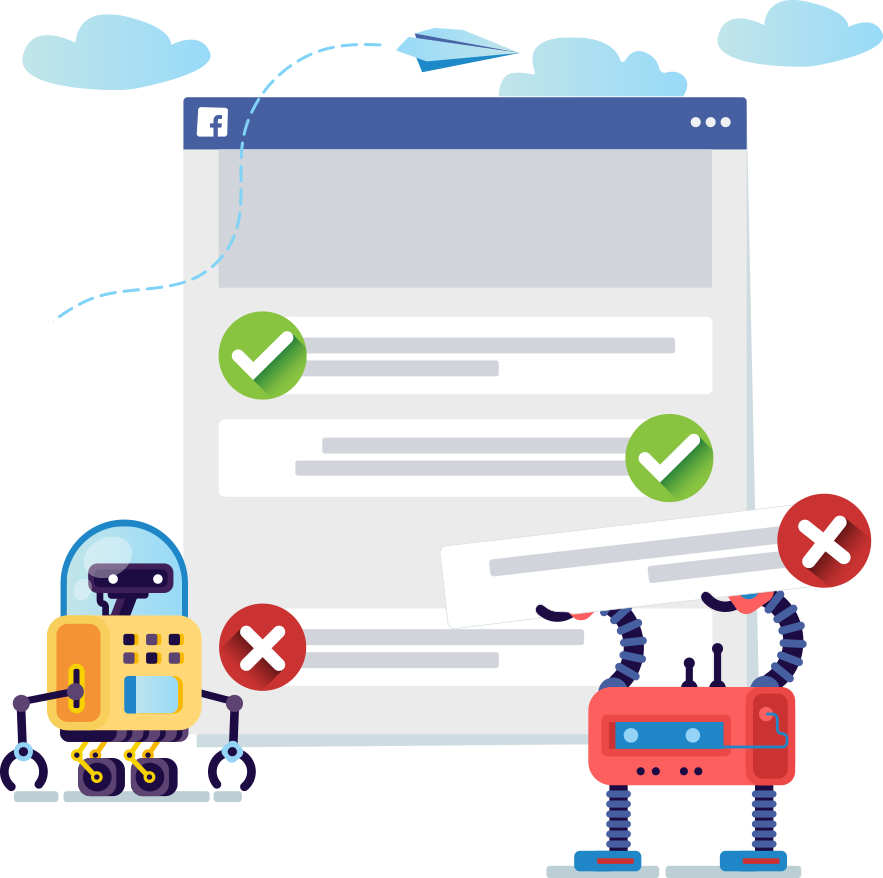
Who moderates the moderators?
One of the most popular approaches to refine information is crowdsourcing (Hosseini et co., 2017), concretization of a libertarian understanding of free speech where “[it’s] up to the individual user, not some committee or administrator, to decide what’s worth reading” (Pfaffenberger in Gibson, 2019, p.2). Yet, using feedback to infer content quality and suitability has proved ineffective for not all users who rate content rate it correctly, shifting the concern on reliability and judgment of participants (Ghosh, Kale, & McAfee, 2011).
In the hiatus between questionable content and questionable ratings is where machine learning chimed in, which addressed the persistent need for automatic detection of toxicity armed with computer vision, speech recognition and language processing (Hosseini et co., 2017).
Perspective API: content moderation
Already a functional feature implemented by the publishing behemoth, The New York Times, is Perspective API, a machine learning extension designed and launched by Google + Jigsaw.
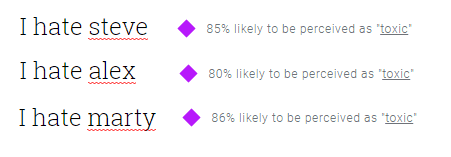
Perspective stands out from the panorama of latest automatic moderators for its capacity to provide real time feedback on the perceived toxicity of the content, rating the analysed data on a spectrum from 1 up to 100 and showing it to the user ante publication (Koen, 2020). Such mechanism can be considered the materialization of what is known as deindividuation theory, affirming that people forced to confront their own behaviour are less likely to transgress (Koen, 2020): in this sense, Perspective constitutes an unprecedented innovation for employing an interdependent synthesis of Artificial Intelligence and Neuroscientific Studies, positing the successful performance of the ML on the instrumentalization of self-awareness. Building on this, yet some vulnerabilities need to be addressed to improve the general performance of detecting semantic toxicity. Focusing on adversarial training could expand the range of modified versions of toxic jargon; Spell checking would be paramount to identify adversarial examples beforehand; and blocking suspicious users would prevent toxic triggers from entering the conversation by setting a threshold of adversarial attempts (Hosseini et. co, 2017).
Conversely, exemplary of context-oriented moderation is ConvoKit. Such algorithmic system enables analysis not only of the comment content, but also relates it to the wider context of the forum social dynamics that influences it (Chang et co., 2020).
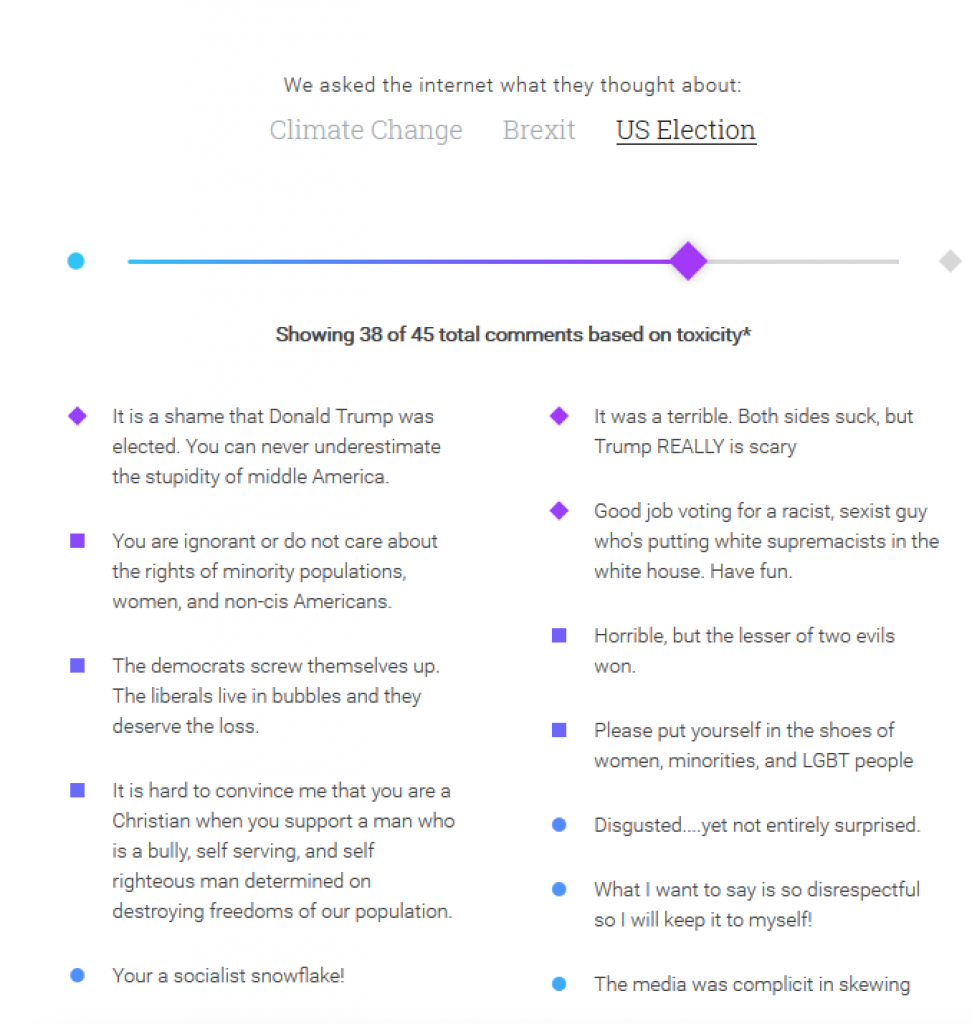
Automatic moderation and the online disinhibition effect
Eventually what can be automated will be automated. AI in comment moderation has constituted an overall improvement in terms of encouraging positive engagement. In fact, it counteracts what is known as the online disinhibition effect, describing the inherent bias of online forums in favour of malicious interference. In fact, the components of anonymity and empathy deficit give an extent of legitimacy to adopting aggressive to harmful communication (Cambridge Consultants, 2019). Again, the forced self-confrontation employed by Perspective constitutes the key element to foster uses a ‘nudging’ technique, thus prompting users to linger and reflect upon what is about to be published (Cambridge Consultants, 2019).
Automatic moderation and self-censorship
According to the Spiral of Silence theory, people measure their own personal opinion in relation to their environment, thus evaluating and anticipating reactions on given issues (Sherrick & Hoewe, 2016). Perspective API’s system is supposed to null the threats to censorship. However, through the exertion of adversarial examples, Perspective has been tested on susceptibility to false alarms, assigning high toxicity scores to harmless wording, due to its deficiency of semantic analysis. It fails to integrate the contextual outline of the discussion, thus ignoring how different social environments may expect different expected sanctions for transgression (Gibson, 2019). Consequently, it may fall into encouraging self-censorship and silencing of minority perspectives, thus hindering the qualitative diversity of online discussions (Koen, 2020).
In fact, measuring the quality of interaction exclusively on positive content prevent actual valuable interaction, impacting public attitude towards the topic of discussion favoured on the news site (Sherrick & Hoewe, 2016). The performance of the moderator has the power to mould the discussion more than any other contributor (Gibson, 2019), therefore some cautiousness should be taken into account while determining and enforcing baseline rules of discussion, so to preserve the quality of the conversation (Sherrick & Hoewe, 2016).
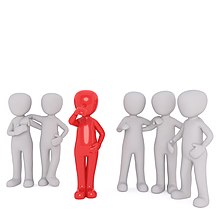
References
Adams, C. (2018, May 23). New York Times: Using AI to host better conversations. Retrieved September 27, 2020, from https://blog.google/topics/machine-learning/new-york-times-using-ai-host-better-conversations/
Cambridge Consultants. Ofcom.org.uk. 2020. [online] Available at <https://www.ofcom.org.uk/__data/assets/pdf_file/0028/157249/cambridge-consultants-ai-content-moderation.pdf> [Accessed 27 September 2020].
Chang, J. P., Chiam, C., Fu, L., Wang, A. Z., Zhang, J., & Danescu-Niculescu-Mizil, C. (2020). ConvoKit: A Toolkit for the Analysis of Conversations. arXiv preprint arXiv:2005.04246.
Ghosh, A., Kale, S., & McAfee, P. (2011, June). Who moderates the moderators? crowdsourcing abuse detection in user-generated content. In Proceedings of the 12th ACM conference on Electronic commerce (pp. 167-176).
Gibson, A. (2019). Free Speech and Safe Spaces: How Moderation Policies Shape Online Discussion Spaces. Social Media+ Society, 5(1), 2056305119832588.
Guberman, J., Schmitz, C., & Hemphill, L. (2016, February). Quantifying toxicity and verbal violence on Twitter. In Proceedings of the 19th ACM Conference on Computer Supported Cooperative Work and Social Computing Companion (pp. 277-280).
Hosseini, H., Kannan, S., Zhang, B., & Poovendran, R. (2017). Deceiving google’s perspective api built for detecting toxic comments. arXiv preprint arXiv:1702.08138.
Jain, E., Brown, S., Chen, J., Neaton, E., Baidas, M., Dong, Z., … & Artan, N. S. (2018, December). Adversarial Text Generation for Google’s Perspective API. In 2018 International Conference on Computational Science and Computational Intelligence (CSCI) (pp. 1136-1141). IEEE.
Sherrick, B., & Hoewe, J. (2018). The effect of explicit online comment moderation on three spiral of silence outcomes. New media & society, 20(2), 453-474.