Can you change the world with a homemade DeepFake?
Kenza Sabri (kenzasabri5@gmail.com)
Zuzanna Kędzia (zuzanna.k0987@gmail.com)
Daria Kochetkova (daria.kochetkova.08@gmail.com)
“At the most basic level, deepfakes are lies disguised to look like truth. If we take them as truth or evidence, we can easily make false conclusions with potentially disastrous consequences.”
Andrea Hickerson, Director of the School of Journalism and Mass Communications at the University of South Carolina
Introduction
In recent years, fake news has become an issue that is a threat to public discourse and human society (Borges et al., 2018; Qayyum et al., 2019). False information spreads quickly through social media, where it can impact millions of users. People tend to believe what they see (Parry, 2009), that is why a phenomenon of a deepfake became an issue.
Nowadays anyone can easily download a deepfake making app on their computer and create explicit content without the consent of those involved. In this paper, we will attempt to create our own deepfake and discuss the feasibility of creating one without any prior experience. We will also discuss how deepfakes contribute to the spread of disinformation online.
Context
Deepfake (the word itself is a blend of the terms “deep learning” and “fake”) is a “hyper-realistic video digitally manipulated to depict people saying and doing things that never actually happened” (Westerlund, 2019). The technologies that used for producing deepfakes are machine learning, “a branch of artificial intelligence that allows computer systems to learn directly from examples, data, and experience…[and] carry out complex processes by learning from data, rather than following pre-programmed rules” (The Royal Society, 2017) combined with artificial intelligence (AI), “computational models of human behavior and thought processes that are designed to operate rationally and intelligently” (i.e., simulate human behavior) (Maras, 2017), which together enables to swap the face of a person on a video into the face of another person. Deepfakes are usually produced by one of the four types of creators:
- communities of deepfake hobbyists (for online humor, such as memes);
- political players – foreign governments, and various activists (for disinformation campaigns to manipulate public opinion);
- other malevolent actors such as fraudsters (to stock manipulation, and other financial crimes);
- legitimate actors, such as television companies (for creating entertaining content) (Westerlund, 2019).
Deepfakes target social media platforms, where conspiracies, rumors, and misinformation spread easily and is consumed quickly by users, therefore affecting not only people, who are disinformed by deepfakes but also a person who is targeted in a video. These videos are hardly removable, because they can be transferred and spread through different platforms even if deleted from the original source. Deepfakes are mostly used for creating revenge pornographic videos, bullying videos, video evidence, political sabotage and propaganda clips, blackmail, and even fake news videos, which consists of methodical disinformation that misrepresents actual news and facts. Nowadays they can also be created for entertaining purposes like memes.
The first deepfake which went viral was published on Reddit in 2017 and was made by someone who used an algorithm to paste the face of actress Gal Gadot onto a pornographic video. Sometimes the face did not track correctly, however, at a glance the video seemed believable. The user named “deepfakes” then published a series of fake celebrity porn videos, which marked the first notable example of a single person who was able to create high-quality and convincing fake videos. Although attempts to put celebrity or other faces onto porn was not something completely new, the mode, speed, and seeming simplicity of the process were. In January 2018, the software called “FakeApp” appeared on Reddit, which allowed users to create their own deepfakes. Most people shared pornographic deepfakes, but some videos were less insulting, for instance, the most popular one was putting Nicholas Cage’s face into random movie scenes.
Later in 2018 deepfakes began to spread further. In April, BuzzFeed published a video of Barak Obama saying words that were not his own. This frighteningly realistic deepfake was made by a single person using FakeApp so as to point at the risks and threats that come with deepfakes.
In 2019, a deepfake of Marc Zuckerberg saying: “Imagine this for a second: One man, with total control of billions of people’s stolen data, all their secrets, their lives, their futures. I owe it all to Spectre. Spectre showed me that whoever controls the data, controls the future” was published, confusing people. Interestingly, instead of deleting the video, Facebook chose to de-prioritize it, so that it appeared less frequently in users’ feeds, and placed the video alongside third-party fact-checker information.
In February 2020, deepfake technology was used by Indian politician Manoj Tiwari. In the original video he criticizes his political opponent Arvind Kejriwal and encourages voters to vote for the party in the English language, but on a deepfake clip, he speaks over 20 different languages used in India in order to target voters across the country. The video went viral on WhatsApp and became the first one ever used for campaigning purposes.
Although deepfakes could be used for positive purposes such as creating voices of those who have lost theirs or updating episodes of movies without reshooting them (Marr, 2019), the number of vicious uses of deepfakes largely dominates that of the positive ones.
Deepfakes aiming to ruin a person’s image and spread disinformation, keep on appearing, highlighting the continued dangers of the deepfake technology. Given the ease in obtaining and spreading misinformation through social media platforms, it is increasingly hard to know what to trust, which results in harmful consequences for informed decision making. (Borges et al.,2018; Britt et al., 2019).
Methodology
In order to proceed with our experiment and create our own deepfake, we have searched for an accessible program that requires no experience in machine-learning technology or video-editing. As search engines are nowadays considered a primary instrument of knowledge production and an initial tool of information gathering (van Dijck), we used YouTube, as we seeked an approachable, yet considerably detailed instructions of the creation process. Numerous videos have pointed to the usage of Colaboratory, a product of Google Research, which is a service that enables writing and execution of arbitrary Python code through their browsers. We have aimed our focus on one particular video with 216 831 views as of October 18, 2020, titled “How To Make Basic DeepFakes with Easy Steps – Dame Da Ne Meme Tutorial”, uploaded by Drew Hunter (DrewHunter). Notably, the author opened his video by specifying “that he knows nothing about writing code”, as he aimed to highlight that a simple deepfake production is within anyone’s reach.
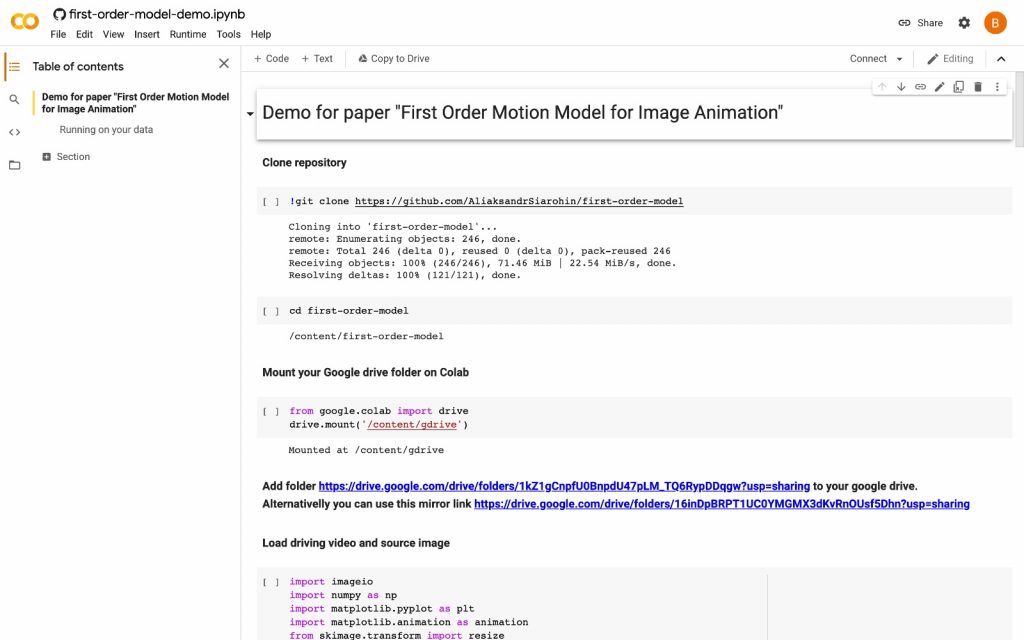
Following the presented procedure, we arrived at a GitHub-provided Colaboratory page called “first-model-order”. GitHub operates as a platform facilitating hosting services for software development and version control – a class of systems that manage changes within designated computer programs, complex web sites, documents, or other compilation of information – using Git (GitHub). Git can be described as a distributed version-control system – a particular form of version control, in which the entire collection of source codes is mirrored on the computer of each developer involved (Chacon, Straub). Git is used primarily for tracking changes made in the source code during the software development process (Scopatz). The “first-model-order” page consists of an interface depicting the steps necessary for the creation of deepfakes, which requires very little programming or copy-pasting of the pre-provided sequences, if relying on Drew Hunter’s video. Each step must start by clicking on the play button right below a given step’s title. Starting the procedure included inserting a missing passage “!pip install PyYAML==5.3.1” above the first lines of code, as directed by the video author, and pressing “play”.
The next step involves granting access to a Google account, which is done by pasting the generated access code into the Colab document. This action is necessary for establishing a connection between Colab and a designated Google drive folder, which acts as a source of content for the deepfake creation. A pre-curated folder called “first-order-motion-model” must be added to Google drive account and is downloadable from the Colab sheet directly. It includes a number of images and videos that can be treated as proposals of deepfake models, all in 256 x 256 pixel format, as well as two files containing the algorithms that ultimately create the deepfake. Additionally, any personal file can be pasted into the folder, as long as it meets the requirement of 256 x 256 format.
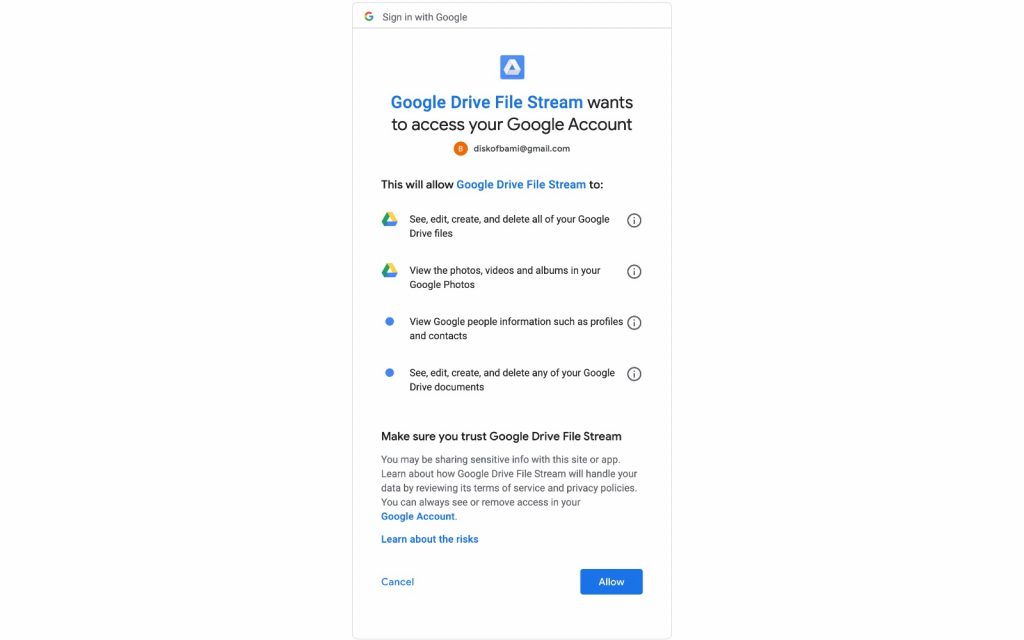
In order to produce a deepfake using “first-model-order” page, the user must choose two files, one image and one video, that will be combined for the purpose of deepfake creation. Following the procedure, the user adjusts the two pre-existing lines of code with the image and video assigned files, as the ends of the code lines must include the files’ names. The user is then able to move to the next step, titled “Create a model and load checkpoints”. In that part of the process, the pre-downloaded algorithm titled “vox-cpk.pth.tar” is activated. Importantly, without positioning the algorithm file within the Google drive folder the deepfake-creating mechanism cannot be mobilized. This particular step has caused a considerable amount of issues, as the Colab document was not generating the desired deepfake, despite the positive completion of all of the previous steps and the existence of the algorithm file within the Google drive folder, even after numerous attempts to refresh both Google drive folder and the Colab sheet. That problem has led to a need to restart the procedure, as it seemed that only after a fully “clean”, continuous step-by-step engagement with the page achieved results.
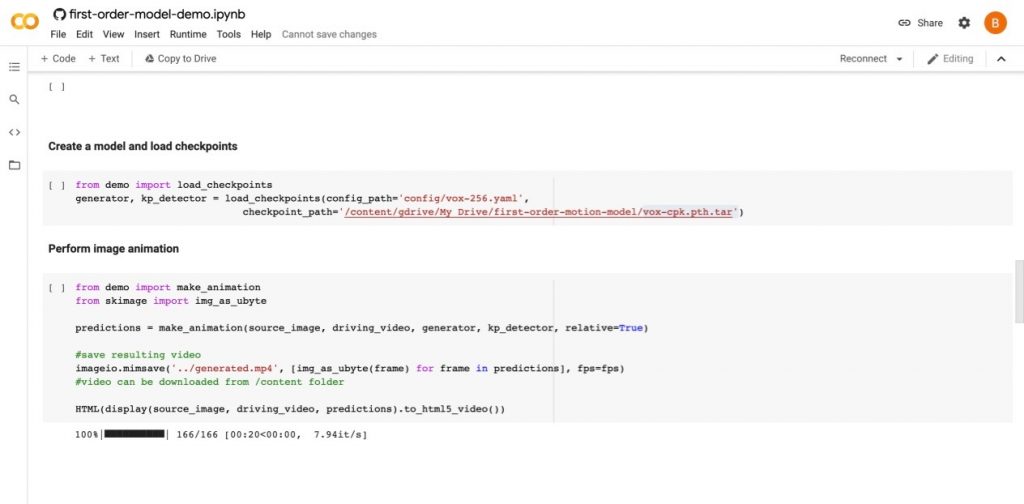
After successful completion of the preceding steps, the user is able to perform image animation and is then presented with a mp4 file, which can be downloaded. In overall, we were satisfied with the generated deepfake samples, the template and original ones likewise. Regrettably, the sound became an issue that followed, as the Colab document did not offer a voice manipulation service. Sound-generating programs were found online, however, they were either faulty, consumed a vast amount of time or could only bend the user’s own voices, without the possibility to change the voice’s pitch or tone. Our final result was a deepfake video of Donald Trump, using a voice generating software that imitates Trump’s voice called VoCodes. The alignment of sound and video proved to be another issue – video manipulation using video editing software caused additional complications. Our personal video, in which one of us uttered the words spoken by the voice generator, did not perform well as the deepfake driving model. Finally, we used the video editing software Final Cut Pro to align the video that we created with the generated audio. Subsequently, we have settled for a result lacking in perfection, however, we were still eager to observe the human reactions to this creation.
The received feedback varied in type and justification with the general consensus that it did not fool anyone. While most people believed the video to be fake because of the lack of alignment between the words uttered and the movement of the mouth, one person justified the falseness of the video by stating ‘’Trump would never say those words’’.
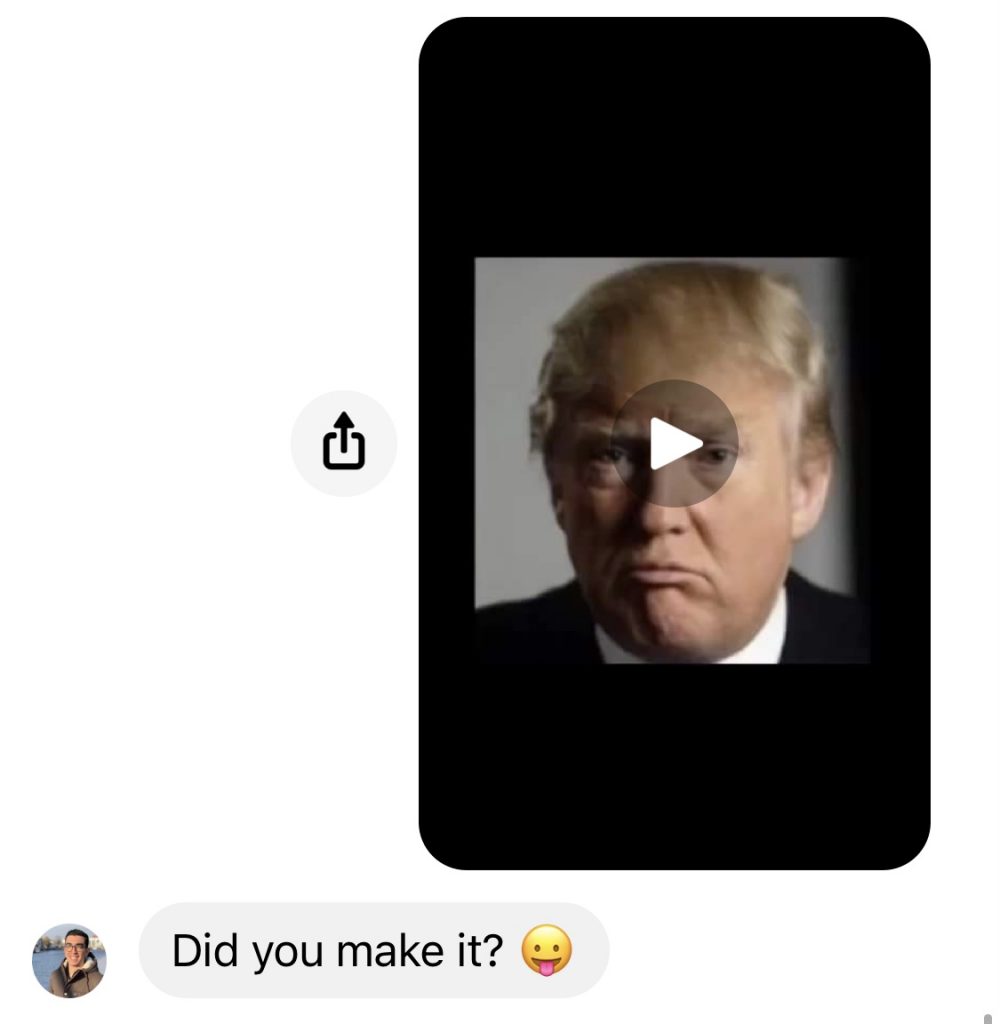
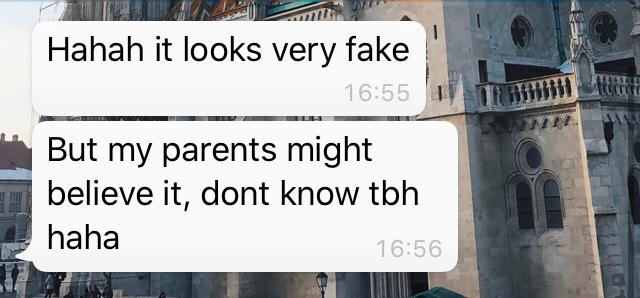
Fake News
In the past decade, we have witnessed a notable rise in the spread of false information online, especially on social media platforms. This practice of Fake News is defined as ”news articles that are intentionally and verifiably false, and could mislead readers” (Allcott and Gentzkow; 213). Fake news has been proven to be extremely dangerous, especially when used in the context of politics such as the 2016 US elections where it was alleged that fake news might have been pivotal in the presidential election of Donald Trump (Allcott and Gentzkow; 232). This issue is especially present for people who rely primarily on social media to gather their news. According to a survey conducted by Ipsos Public Affairs for BuzzFeed News in 2016, 75% of American adults are fooled by fake news headlines (Silverman and Singer-Vine). A Pew Research Center survey in 2018 revealed similar information and found that younger Americans are better at recognizing factual news statements from opinions than older Americans (Gottfried and Grieco). Indeed, adults and older generations who are used to their news being delivered factually through reliable sources such as television and newspapers have a harder time discerning fake news from real news on social media platforms.
While written fake news is an immense threat to our society, with efforts from the readers, the information can be verified by consulting reputable sources. However, in the last three years, we have seen the emergence of a new type of fake news content in the form of deepfakes. The dangers of deepfakes have already been demonstrated in the porn industry, violating both the celebrities’ and actresses’ privacy and work. But the greater issue at hand, most people argue, is the use of deepfakes in a political context threatening democracies and national securities around the world. With the release of these deepfakes, our brains are not accustomed to question videos. ”Most people may be poorly equipped to discern when they are being deceived by deepfakes. Rössler et al. (2018) found that people correctly identify fakes in only about 50% of cases, statistically as good as random guessing” (Vaccari and Chadwick; 3). Vaccari and Chadwick found that ”Anecdotal evidence suggests that the prospect of mass production and diffusion of deepfakes by malicious actors could present the most serious challenge yet to the authenticity of online political discourse”. They explain that even if these deepfakes do not convince the public of their authenticity, the more they are made and shared, they will put doubt in people’s mind which would result in a mistrust of all news sources and ultimately, the governments, and lower people’s individual sense of responsibility towards what they, in turn, share online.
In May of 2018, the Belgian political party Socialistische Partij Anders created and posted a deepfake on their Facebook page showing American president Donald Trump addressing the Belgian people on climate change and stating that Belgium ”is not taking any measures […] shame, total shame […] Belgium, don’t be a hypocrite”. The video is accompanied by Flemish subtitles, except for the last sentence ”we all know that climate change is fake, just like this video”. This deepfake was produced to draw people’s attention and get them to sign a petition about climate change actions. However, the stunt backfired when people believed it to be real, leaving comments such as ‘Humpy Trumpy has to look at his own country with his crazy child killers who just walk through the schools with the heaviest weapons” and ‘trump always has to attack someone”. Even though this video was not made to be believed, people were quick to react. A spokesperson for the party said ”It is clear from the lip movements that this is not a genuine speech by Trump, and we also state this at the end of the video” (Von Der Burchard). This deepens the arguments that with such technology available to everyone, these deepfakes do not need to be perfect or even well made to create a sense of chaos and confusion.
Legality
With the rise of such technology, many have wondered what the law is or should be doing to prevent deepfakes from circulating online. As of today, to prevent deepfakes from influencing political elections, Texas and California passed laws to criminalize publishing and distributing deepfake videos intended to harm a candidate or influence results within 30 days or 60 days of an election.
In China, The Cyberspace Administration of China has passed a new regulation banning the use of deepfakes, unless they are marked as such. The CAC stated “With the adoption of new technologies, such as deepfake, in online video and audio industries, there have been risks in using such content to disrupt social order and violate people’s interests, creating political risks and bringing a negative impact to national security and social stability,”
In Europe however, no new laws have passed regarding the creation and distribution of deepfakes. The EU relies mainly on the Code of Practice on Disinformation which includes ”commitments such as, amongst other things, ensuring that services have safeguards against disinformation and easily‐accessible tools for users to report disinformation” (Lovells; 1)
In a more general context where no laws target specifically deepfakes, the victims can sue the creators of the videos using already existing laws such as harassment, defamation, or copyright.
Conclusion
To conclude, the dangers that deepfakes represent to our democracies are very much real when in the wrong hands. Our experiment has proven that making a believable deepfake with free softwares and no prior experience is not easy, but the rate at which this technology is advancing is scary. As many people have proven, these videos don’t have to be perfect to create confusion and distrust among people.
References
Allcott, Hunt, and Matthew Gentzkow. “Social Media and Fake News in the 2016 Election.” Journal of Economic Perspectives, vol. 31, no. 2, 1 May 2017, pp. 211–236, 10.1257/jep.31.2.211.
Borges, L., Martins, B., & Calado, P. 2019. Combining Similarity Features and Deep Representation Learning for Stance Detection in the Context of Checking Fake News. Journal of Data and Information Quality, 11(3): Article No. 14. https://doi.org/10.1145/3287763
Britt, M. A., Rouet, J.-F., Blaum, D., & Millis, K. 2019. AReasoned Approach to Dealing With Fake News.Policy Insights from the Behavioral and BrainSciences, 6(1): 94–101.https://doi.org/10.1177/2372732218814855
Burchard, Hans von der. “Belgian Socialist Party Circulates ‘Deep Fake’ Donald Trump Video.” POLITICO, 21 May 2018, www.politico.eu/article/spa-donald-trump-belgium-paris-climate-agreement-belgian-socialist-party-circulates-deep-fake-trump-video/.
Castro, Daniel. “Deepfakes Are on the Rise — How Should Government Respond?” Www.Govtech.Com, Jan. 2020, www.govtech.com/policy/Deepfakes-Are-on-the-Rise-How-Should-Government-Respond.html.
Chacon, Scott; Straub, Ben. “Getting Started – About Version Control”. Pro Git, 2 ed. 2014.
“GitHub.” GitHub. Retrieved 19 October 2020
Gottfried, Jeffrey, and Elizabeth Grieco. “Younger Americans Are Better than Older Americans at Telling Factual News Statements from Opinions.” Pew Research Center, Pew Research Center, 23 Oct. 2018, www.pewresearch.org/fact-tank/2018/10/23/younger-americans-are-better-than-older-americans-at-telling-factual-news-statements-from-opinions/.
“How To Make Basic DeepFakes with Easy Steps – Dame Da Ne Meme Tutorial” YouTube, uploaded by Drew Hunter, 2 April 2020, https://www.youtube.com/watch?v=peOKeRBU_uQ&ab_channel=DrewHunter
Maras, M-H (2017) “Social media platforms: Targeting the ‘found space’ of terrorists.” Journal of Internet Law 21(2): 3–9.
Marr, B. (2019, July 22). The best (and scari- est) examples of AI-enabled deepfakes. Available at https://www.forbes.com/sites/bernardmarr/2019/07/22/the-best-and- scariest-examples-of-ai-enabled-deepfakes/
Parry, ZB (2009) Digital manipulation of photographic evidence: Defrauding the courts one thousand words at a time. Journal of Law, Technology & Policy 81: 176.
Qayyum, A., Qadir, J., Janjua, M. U. & Sher, F. 2019. Using Blockchain to Rein in the New Post-Truth World and Check the Spread of Fake News. IT Professional, 21(4): 16–24. https://doi.org/10.1109/MITP.2019.2910503
Scopatz, Anthony; Huff, Kathryn D. (2015). Effective Computation in Physics. O’Reilly Media, Inc. p. 351. ISBN9781491901595. Archived from the original on 7 May 2016. Retrieved 20 April 2016.
Silverman, Craig, and Jeremy Singer-Vine. “Most Americans Who See Fake News Believe It, New Survey Says.” BuzzFeed News, 6 Dec. 2016, www.buzzfeednews.com/article/craigsilverman/fake-news-survey#.dim75q97X. Accessed 7BC.
sp.a. “Als We Solidair Zijn, Dan Zal Het Ons Lukken.” Www.Facebook.Com, 9 Oct. 2020, www.facebook.com/watch/?v=719597495435207.
The Royal Society (2017) Machine Learning: The Power And Promise Of Computers That Learn By Example. London: The Royal Society. Available at: https://royalsociety-org.proxy.uba.uva.nl:2443/∼/media/policy/projects/machine-learning/publications/machine-learning-report.pdf (accessed 9 September 2018).
Thornton, Hogan Lovells-Penelope, et al. “Deepfakes: An EU and U.S. Perspective | Lexology.” Www.Lexology.Com, 24 July 2020, www.lexology.com/library/detail.aspx?g=8f038b17-a124-46b2-85dc-374f3ccf9392.
Vaccari, Cristian, and Andrew Chadwick. “Deepfakes and Disinformation: Exploring the Impact of Synthetic Political Video on Deception, Uncertainty, and Trust in News.” Social Media + Society, vol. 6, no. 1, Jan. 2020, p. 205630512090340, 10.1177/2056305120903408.
Van Dijck, José —— Search engines and the production of academic knowledge
Villas-Boas, Antonio. “China Is Trying to Prevent Deepfakes with New Law Requiring That Videos Using AI Are Prominently Marked.” Business Insider, 30 Nov. 2019, www.businessinsider.com/china-making-deepfakes-illegal-requiring-that-ai-videos-be-marked-2019-11?international=true&r=US&IR=T.
Villas-Boas, Antonio. “China Is Trying to Prevent Deepfakes with New Law Requiring That Videos Using AI Are Prominently Marked.” Business Insider, 30 Nov. 2019, www.businessinsider.com/china-making-deepfakes-illegal-requiring-that-ai-videos-be-marked-2019-11?international=true&r=US&IR=T.
Westerlund, M. 2019. The Emergence of DeepfakeTechnology: A Review. Technology InnovationManagement Review, 9(11): 39-52.http://doi.org/10.22215/timreview/1282