Amazon: Bigger data are not always better data?
Most new media studies focus on the giants of the web, such as Google, Facebook, Twitter. They are specialized in search engines and social media and have accumulated tremendous data assets among their business. Boyd & Crawford (2012) mentions six provocations to criticize big data. In this article, I will use Amazon, a world-leading e-commerce company, as an example of the greatest product database, to explore Boyd & Crawford’s statement “Bigger data are not always better data.”
Data is a primary tool for academic, product, and idea study, a measurement of success, and a long-term asset for business when used to make decisions and strategies. “Big” data is still data, but in a more dynamic schema than traditional data, based on “3 Vs” of data: Volume, Variety, Velocity. Also, it’s important to be clear that “bigger” data is not just “more” data.
According to MyCustomer.com, Werner Vogels, the CTO and VP of Amazon, delivered a keynote speech at CeBIT regarding Amazon’s cloud computing, said that “as a company collecting vast amounts of data, bigger data is better – the more information you have, the better the recommendations.” From a business point of view, corporate data as an ecosystem feeds its product, service, opinion, and policy-making decision. In this, big data is an asset, possibility, and opportunity. By deepening into bigger consumer-driven and transaction-based data, “Amazon will likely use data from all of its platforms to create an ambient e-commerce experience for customers,” said Kenneth Sanford of Dataiku. Compared to those tech companies that provide data integration, cloud applications, and platform services, including IBM, Oracle, and Dell EMC, Amazon could get more actionable insights from the real value of big data. This process is seductive like what biological scientists have achieved. Venturini et al. (2015) also support that “the excitement of having ‘bigger’ data that are as large and rich as the natural scientists” is understandable.
The processing of big data begins with raw data. Ownership, flexibility, and control are keys of raw data. CNBC reported in November 2018 that Amazon switched off its largest Oracle database, moved 88% to its own data warehouse, Redshift, and closed the last database for consumer business in October 2019. Now, Amazon’s own cloud computing platforms Amazon Web Services (AWS) support those businesses. It can be said that it is part of Amazon’s strategies to “present themselves as providers of valuable analytics and partners to established and emerging data industries” (2015). According to Gillespie (2014), both the possession of and responsibility of “millions of data points lend a great deal of legitimacy, and algorithms spot patterns that researchers couldn’t see.” The great value of big data is to develop a direct relationship with users, where bigger data can be truly recognized as better data.
In Critical Questions for Big Data, Boyd & Crawford (2012) states that “Big data and whole data are also not the same. Without taking into account the sample of a data set, the size of the data set is meaningless.” Understanding sample is essential in new media research. The accounts on Twitter are not equal to users, so is Amazon. That is, both accounts and users cannot be representative of the population and “all people,” neither accurately reflect the characteristics of the larger group nor think about those who haven’t created an account. In this way, “bigger” data are not better data because they are not representative, regardless of the sample size or data set size.
This kind of representative issue also applies to the conditions of initially skewed or biased data. It is hard for researchers to examine or evaluate the quality of the given data that they are using for data analysis and data representation. In addition, when the sources are uncertain (Amazon utilized Oracle’s database before October 2019, but not their own.), different data streams or samplings may just show us a small part of the reality and truth. Are the existing data or sampling representative and transparent? Buzz, a typical example of bigger data, would not be regarded as better data for research as it may reinforce prejudice and alter the original text. Usually, mistakes are missed out unless researchers publicly account for “the limits of the data set, the limits of which questions they can ask of a data set, and what interpretations are appropriate” (Boyd & Crawford 2012).
Bigger data are not always better data when considering the limits of a single data set and its cumulative, expansive, and amplified effects. Big data analysis may integrate raw data from multiple sources. However, Jesper Anderson, co-founder of open financial data store FreeRisk, explains that “combining data from multiple sources creates unique challenges” (Boyd & Crawford 2012). For example, Amazon API Gateway provides developers with its AWS service to create, deploy, manage, and protect APIs (application programming interface) at any scale. But, when developers combine multiple sources or API services with unknown performance characteristics, how do they know whether AWS (Amazon Web Services) extract, add, delete, update and sync data from users’ online accounts or profiles automatically? They may not even perceive that there is any missing data.
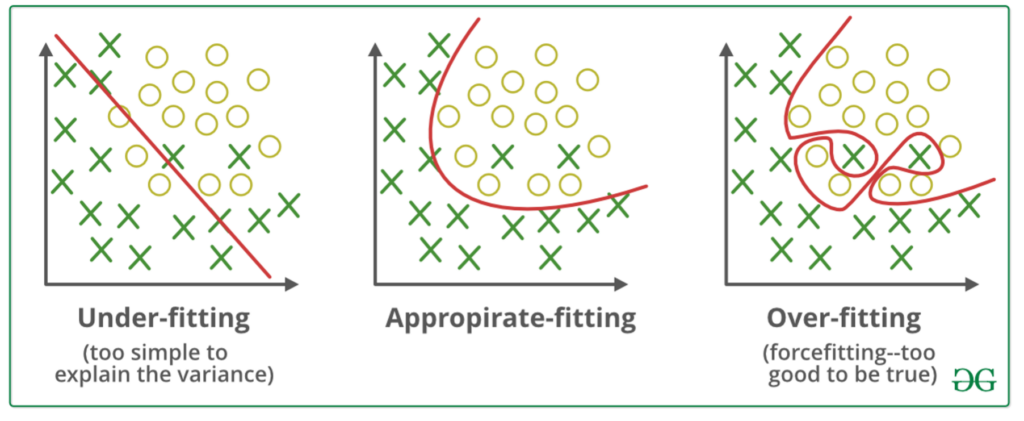
Bigger data are not always better data when lacking optimization models during development. When Amazon strives to collect and monetize our clicks and searches, understanding model fit – choose an accurate classifier algorithm – is needed (see Figure. 1), according to Amazon Machine Learning Developer Guide. But the prediction effect of the trained classifier is not completely positively correlate to the size of the training dataset. In doing so, for a high-variance trained classifier model, “the bigger” (also better) and more data input, the overfitting model will be optimized. But for a trained classifier model with high bias, the size of the training data set is not a critical problem. This kind of under-fitting model itself is not suitable for dealing with “bigger” but poor data. The first reason could be that the model is too simple to process complex classification. And the second reason is that too few efficient features are extracted from the big data sample for further analysis. In this case, bigger data won’t improve prediction accuracy. “The size of data should fit the research question being asked; in some cases, small is best,” said Boyd & Crawford (2012).
Throughout this article, Amazon as a popular online shopping platform with big data strongly helps advance the discussion on bigger data are not always better data. In the end, some conditions and improvements are pointed out to make “bigger” data better data.
Works Cited
Boyd, D., & Crawford, K. (2012). Critical Questions for Big Data: Provocations for a Cultural, Technological, and Scholarly Phenomenon. Information, Communication, & Society, 15(5), 662-679. http://dx.doi.org/10.1080/1369118X.2012.678878
Gillespie, T. (2014). ‘The relevance of algorithms’, in Media Technologies: Essays on Communication, Materiality, and Society, eds P. J. Boczkowski, K. A. Foot & T. Gillespie, MIT Press, Cambridge, MA, 167–194. https://mitpress.universitypressscholarship.com/view/10.7551/mitpress/9780262525374.001.0001/upso-9780262525374-chapter-9
Mitchell, Tom M. “Machine Learning.” Amazon, McGraw Hill, 2017, https://docs.aws.amazon.com/machine-learning/latest/dg/model-fit-underfitting-vs-overfitting.html.
Nautiyal, Dewang. “ML: Underfitting and Overfitting.” GeeksforGeeks, 20 Aug. 2021, https://www.geeksforgeeks.org/underfitting-and-overfitting-in-machine-learning/.
Steers, Natalie. “‘Big Data Is about Storage, Not Just Analytics’ – Amazon CTO.” MyCustomer, 9 Mar. 2012, https://www.mycustomer.com/marketing/data/big-data-is-about-storage-not-just-analytics-amazon-cto.
Venturini, T., Bounegru, L., Gray, J., & Rogers, R. (2018). A reality check(list) for digital methods. New Media & Society, 20(11), 4195–4217. https://doi.org/10.1177/1461444818769236