Big Data And AI: Fake Fake News or Fight Fake News
In nowadays, the booming of information technology allows individuals to become content creators and publishers, a great number of data quickly spreads globally on the Internet. Meanwhile, new dimensions for Fake News are born in the rising of social media and empowered artificial intelligence, which leads to growing distrust and even post-truth phenomenon. However, “technology is neither good nor bad; nor is it neutral” and data can never speak for itself (Kranzberg 1986, p. 545). By mainly classifying rumours with content patterns in techniques’ identification, this essay aims to discuss to what extent AI and Big Data could help to fight fake news, based on the method of boyd and Crawford’s Critical Data Studies.
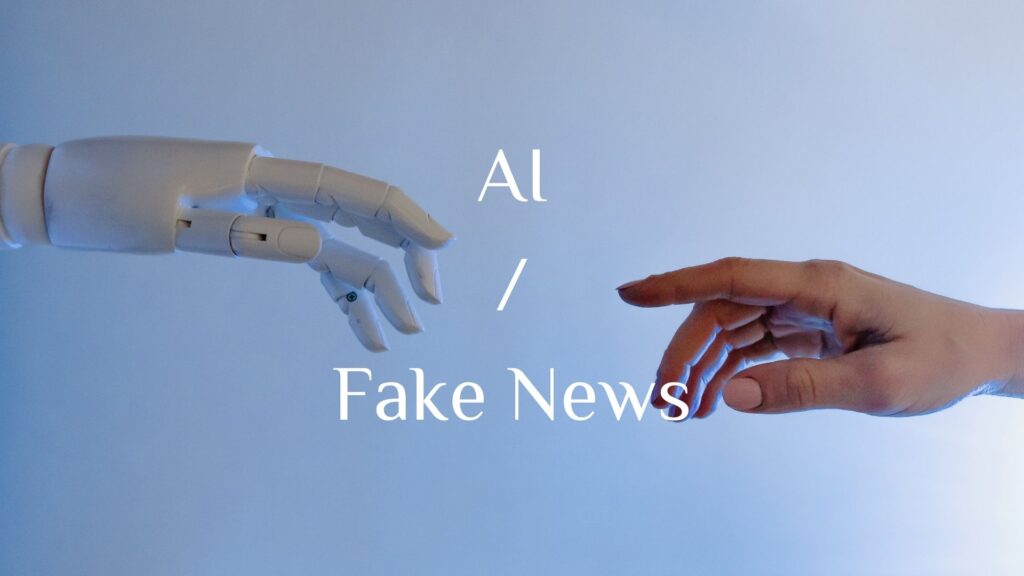
Fake news as misleading information highly targeted social media users for swaying in political voting or commercial interests secretly hides in the shared news. Particularly, from the 2016 U.S. presidential elections to the recent covid-19 pandemic period, the usage of the term “fake news” has increased by 365%, Collins Dictionary said (Flood, 2017). Therefore, in case of the damage that might cause by human fact checkers’ late reactions, Big Data and Artificial Intelligence (AI) are introduced for important applications to instantly identifying fake news.
From content patterns of techniques’ identifying misinformation, this essay aims to discuss to what extent AI and Big Data could help to fight fake news, based on the method of Boyd and Crawford’s Critical Data Studies.
The relationship between Big Data and AI
Big Data is usually indicating to the huge in volume (size); high in velocity (speed); exhaustive in scope; fine-grained in resolution; relational; flexible in extent and scale (Kitchin, 2013). Boyd and Crawford (2012) note that Big Data is more about its capability in searching, aggregating and “cross-reference large data sets” (p. 663). In contrast to Big Data, AI is the output of the processed data which trains its intelligence. This is supported by O’Leary (2013) that AI is also about developing volumes, velocities and a variety of unstructured data to be categorised or structured.
Critical Data Studies introduces the approach to openly interrogating the Big Data paradigm and questioning quantitative research as objective truth. According to Boyd and Crawford (2012), data cannot speak for itself, hereby, they argued that it produces both utopian and dystopian rhetoric. On the one hand, Big Data change the definition of knowledge and social life which particularly in the field of public health-related discipline, terrorism, and even climate change. On the other hand, Big Data might lose its meaning from the context, because it is a raw input that is necessary to be cleaned for valuable (ibid). Furthermore, the concentration of AI and Big Data usage might “limited access to Big Data creates new digital divides”.
Fight Fake News with AI
Normally, AI technology can be used to identify fake news from language patterns. The work of using deep neural networks to detect fake news by O’Brien, et al. (2018) could be introduced here as an example.
In the paper of The Language of Fake News: Opening the Black-Box of Deep Learning-Based Detectors, researchers used the deep-learning method to capture the subtle differences in the language of real news and fake news (O’Brien, et al. 2018). Convolutional neural networks as a powerful tool in this examination are to train datasets to determine the fake news and real news.
Particularly, in the training process, approximately 12,000 fake news sample articles were collected from the research data set called Kaggle. More than 11,000 pieces of news from the New York Times and Guardian were used for real news data sets as well. All the articles are published during the 2016 United States Presidential Election and contain the word “Trump”.
Although the results found that fake news likes to use exaggerated or superlative adjectives, while real news tends to use relatively conservative words, the cleaning of raw data might lead to the loss of meaning as well.
Fake Fake News with AI
AI technology could not only be used to identify fake news, but also fabricates fake news and spreads them. Take OpenAI’ s GPT-3 as an example. Released in 2020, OpenAI’s Generative Pre-Trained Transformer 3 (GPT-3), is a Transformer language model which is unsupervised trained with over billions of parameters. In particular, GPT-3 could generate seemingly believable text based on the provided introductory sentences, and when used to write news.
For instance, while OpenAI fed it with the words: “Russia has declared war on the United States after Donald Trump accidentally fired a missile in the air.”
AI could supplementarily text as:
Russia said it had “identified the missile’s trajectory and will take necessary measures to ensure the security of the Russian population and the country’s strategic nuclear forces.” The White House said it was “extremely concerned by the Russian violation” of a treaty banning intermediate-range ballistic missiles…
(Helberg, 2021)
Indeed, the OpenAI will not deliberately generate fake news, but it cannot stop criminals from unethical issues. Therefore, OpenAI chose not to publish key data and codes because of its power. However, the news of GPT-3 exclusively licensed to Microsoft in 2020 has caused concerns about AI concentrates power (Hao, 2020). People argued that the lab should benefit humanity instead of Microsoft which is one of the richest companies in the world (ibid).
Conclusion
Fake news cannot simply understand as a mathematical problem about algorithms and data, but a question about human choices in dealing with the truth. The limitation of this essay is lacking explanation about AI identification in the dissemination patterns and the ethical problems about data surveillance. Honestly, both individuals and technology are having the responsible for fake news. As new consumers in the digital age, we need to be more critical for acquiring and sharing information.
References:
Boyd, D., & Crawford, K. (2012). CRITICAL QUESTIONS FOR BIG DATA. Information, Communication & Society, 15(5), 662–679. https://doi.org/10.1080/1369118x.2012.678878
Flood, A. (2018, February 9). Fake news is “very real” word of the year for 2017. The Guardian. https://www.theguardian.com/books/2017/nov/02/fake-news-is-very-real-word-of-the-year-for-2017
Hao, K. (2020, September 23). OpenAI is giving Microsoft exclusive access to its GPT-3 language model. MIT Technology Review. https://www.technologyreview.com/2020/09/23/1008729/openai-is-giving-microsoft-exclusive-access-to-its-gpt-3-language-model/
Helberg, J. (2021). The Wires of War: Technology and the Global Struggle for Power. Avid Reader Press / Simon & Schuster.
Kitchin, R. (2013). Big data and human geography: Opportunities, challenges and risks. Dialogues in Human Geography, 3(3), 262–267.
Kranzberg, M. (1986). Technology and history: “Kranzberg’s laws”. Technology and Culture, 27, 544–560.
O’Brien, N., Latessa, S., Evangelopoulos, G., & Boix, X. (2018). The language of fake news: Opening the black-box of deep learning based detectors.
O’Leary, D. E. (2013). Artificial Intelligence and Big Data. IEEE Intelligent Systems, 28(2), 96–99. https://doi.org/10.1109/mis.2013.39