Do recommender systems afford us to both browsing and searching?
Keywords: Digital humanities, Serendipity, Algorithms, Recommender systems
Searching vs. Browsing
Using search engines, such as Google Scholar, for information gathering and academic writing has become ubiquitous(Van Dijck 2010). It is almost instinctive for students and academics to start writing by using search engines because they offer convenient access to relevant texts that seemly solve the conundrum of “that much information probably exceeds our ability create reliable guides to it”(Ramsay 2010).
Whereas, searching for information via search engines alone has been described as insufficient by Stephen Ramsay(2010). Facing the fact that it is beyond one’s capability to read all the books and articles of a field of interest, the digital humanism scholar presented two fashion of gathering information: searching and browsing(Ramsay, 2010). Attempts to solve such conundrum never stopped, this explains why people like reading lists or even take classes in school — they want to obtain a clear picture of what is known. Ramsay(2010) argued that although search engines provide efficient access to relevant information, such mode of acquiring an enormous amount of information merely endangers researchers with the anxiety of realizing there is so much left to read and not knowing the path. When provide an enormous amount of literature, search engines present information in a chaotic order: the canonicity of research engines has been questioned regarding issues such as its nontransparent profiling system(Van Dijck 2010).
Ramsay(2010) introduced an alternative way of looking up for information: browsing. While purposive searching is handful in finding answer for a developed question, browsing is serendipitous and could be more illuminating. Browsing enables one to encounter relevant and useful information unexpectedly, and it helps to make sense of the vast network connections. Ramsay posits that library enable one to not only purposive search but also browsing around the stacks, but it is impossible to browsing around through search engines. Thus, in order to “understand each path through the vast archive”(Ramsay 2010), searching online alone is inadequate.
Serendipity in recommender systems
By comparing browsing and searching, Ramsay(2010) presented the problem of search engines: it is infeasible to obtain a clear overview of the topic of interest by showing a large amount of relevant literature. He then argued that serendipitous browsing could compensate for this shortcoming, but it is unattainable through online search engines(Ramsay 2010). Search engines adopting the recommender system seem to overcome this problem researchers face with the overwhelming amount of information.
In recent years, the focus of recommendation algorithm has gone beyond accuracy and shifted towards predicting users’ preferences(Kaminskas and Bridge 2016). Serendipity-oriented algorithms could surprise users with unexpected and interesting topic. Serendipity is a perplexing concept that is hard to define, and it is commonly agreed to be composed of three key elements: relevance, novelty and unexpectedness(Kotkov, Wang, and Veijalainen 2016). Contents recommended should be related to users’ interests, unknown by users and surprising to users. Thus, serendipitous algorithms should be able to recommend users with different, relatively unknown contents.
Can we have serendipity in recommender systems?
Corneli et al.(2014) situated serendipity in the online searching context and proposed that the shift in focus is a crucial indicator for serendipity. Corneli et al.(2014) suggested that the shift in focus from the original object of focus to the recommended content might create a bridge between the former and the latter, it echos with Ramsey(2010) who states that serendipitous browsing allow users to make sense of the vast network connections. Su and Khoshgoftaar(2009) further divided the recommender system into three different categories. In the following, this commentary will give examples for each category and discuss whether there is serendipity in these empirical examples.
Content based filtering
Content based filtering adopts item features to recommend other similar items(Corneli et al. 2014). The recommendation is based on the online behaviors of users. Bhatt(2013) shared his experience with Amazon on the Guardian, saying that Amazon advertised about Terry Pratchett’s upcoming book. Bhatt(2013) is convinced that such recommendation is based on tracking user’s purchasing habits, as he purchased Terry Pratchett’s book in paper and Kindle e-book before. The inherent logic of the content base filtering is that the tastes of users are not changing, and it is more reliable to predict their preferences by analyzing things users used to like. Although such recommendation might be accurate, it fails to create a serendipitous experience for users as they are neither unknown nor surprising to users.
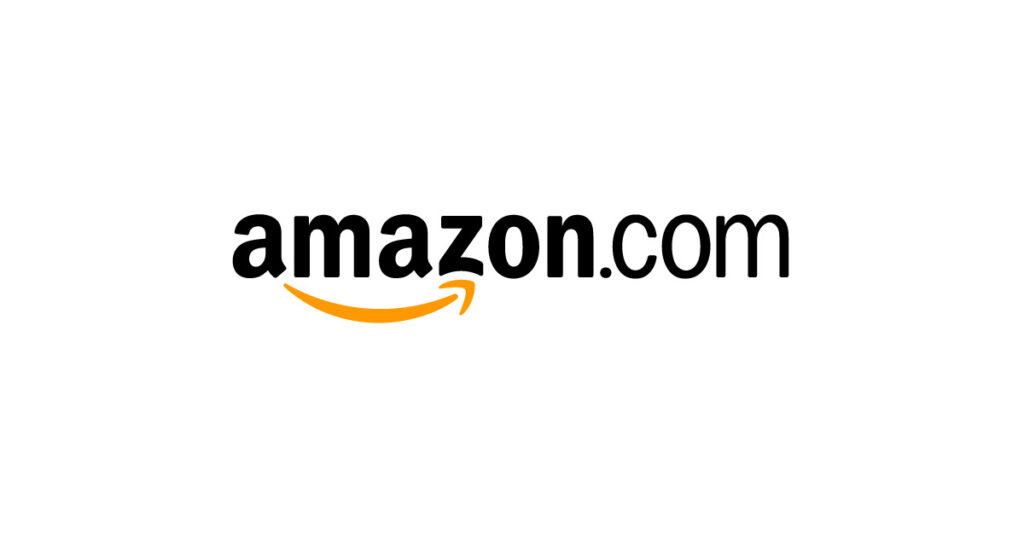
Collaborative filtering
Collaborative filtering collect preferences and information of many users and recommendations are made based on the collaborating information among users of similar tastes(Corneli et al. 2014). Spotify, the stream music platform, gathers information through recording users’ stream counts and counting songs that they clicked the heart bottom(Guneysu 2020). Data of users with similar music tastes will be used to generate recommendations on the “Discover New Music” session. Through combining data of different users, collaborative filtering is more likely to make users feel serendipitous while browsing because contents recommended are more likely to be relevant, novel, and unexpected.
Hybrid filtering
Hybrid filtering combines the algorithm of collaborative filtering and content based filtering(Corneli et al. 2014). Yaman(2021) used models to explain how Netflix takes into account both watching habits of individual users(content based filtering) and features of users with similar tastes(Collaborative filtering). Hybrid filtering is more likely to lead to serendipitous browsing than content based filtering, but its recommendations are less likely to be as unexpected and novel as that of collaborative filtering.
Conclusion
This commentary discussed whether recommender systems has both browsing and searching capacities that were regarded as non-existent by Stephen Ramsay(2010). By applying Ramsay’s concept of browsing, searching, and serendipity on three different types of recommender systems, this commentary demonstrates that while collaborative filtering and hybrid filtering afford users to browse and have serendipity, content based filtering do not. Although it is paradoxical to say a designated algorithm can generate serendipitous information, recommender systems are handy tools in helping finding serendipitous information.
References
Bhatt, Neerav. 2013. “The Science behind Online Content Recommendations.” The Guardian, May 31, 2013, sec. Media Network. https://www.theguardian.com/media-network/media-network-blog/2013/may/31/science-online-content-recommendations.
Corneli, Joseph, Anna Jordanous, Christian Guckelsberger, Alison Pease, and Simon Colton. 2020. “Modelling Serendipity in a Computational Context.” ArXiv:1411.0440 [Cs], April. http://arxiv.org/abs/1411.0440.
Dijck, J. van. 2010. “Search Engines and the Production of Academic Knowledge.” International Journal of Cultural Studies 13. https://doi.org/10.1177/1367877910376582.
Guneysu, Ulku. 2020. “How Is Spotify’s Thriving Recommendation System Becoming A New Advertising Platform?” The Startup (blog). December 15, 2020. https://medium.com/swlh/how-is-spotifys-thriving-recommendation-system-becoming-a-new-advertising-platform-a2b97ffe2012.
Kaminskas, Marius, and Derek Bridge. 2016. “Diversity, Serendipity, Novelty, and Coverage: A Survey and Empirical Analysis of Beyond-Accuracy Objectives in Recommender Systems.” ACM Transactions on Interactive Intelligent Systems 7 (1): 2:1–2:42. https://doi.org/10.1145/2926720.
Kotkov, Denis, Shuaiqiang Wang, and Jari Veijalainen. 2016. “A Survey of Serendipity in Recommender Systems.” Knowledge-Based Systems 111 (November): 180–92. https://doi.org/10.1016/j.knosys.2016.08.014.
Ramsay, Stephen. 2014. “The Hermeneutics of Screwing Around; or What You Do with a Million Books.” In Pastplay, edited by Kevin Kee, 111–20. Teaching and Learning History with Technology. University of Michigan Press. https://doi.org/10.2307/j.ctv65swr0.9.
Su, Xiaoyuan, and Taghi M. Khoshgoftaar. 2009. “A Survey of Collaborative Filtering Techniques.” Advances in Artificial Intelligence 2009 (October): e421425. https://doi.org/10.1155/2009/421425.
Yaman, Ayse. 2021. “Hybrid Recommender System-Netflix Prize Dataset.” Medium. March 22, 2021. https://medium.com/codex/hybrid-recommender-system-netflix-prize-dataset-e9f6b4a875aa.