“Do fans also like…”? — in what ways do big artists profit from Spotify’s recommendation system
WG1 – 7
In recent years streaming services have exponentially grown in their popularity. Streaming services like Netflix and Spotify have amassed large numbers of users, making them some of the most well-known platforms to date.
Especially for music, Spotify has emerged as a successful streaming service since its launch in 2008. According to Werner (2020), media technologies are intertwined in the act of listening to music, it is continuously co-creating the experience. This holds true when considering Spotify’s utilization of a recommendation system. Algorithmic recommendation systems have been known to guide consumption choices (Holtz et al. 2020). The choices these systems guide consumers to make have been criticized, some have speculated that recommendation systems lead to more homogenous consumption (Salganik et al. 2006). Spotify has also been appointed to that same criticism in both academia and by journalists. Victoria Turk (2021) from Wired describes this homogenous listening experience as a ‘feedback loop’. The same kind of artists and genres keep getting recommended by the system, creating a loop where the consumption becomes monotone.
In this article, we want to focus on the effects of homogenous consumption driven by Spotify’s recommendation system on the visibility of smaller artists in comparison to bigger artists on the platform. We focus in particular on the related artists function Spotify provides. Like many recommendation systems used by big companies, the Spotify recommendation system can be called a ‘black box’, meaning that it lacks transparency. Although, some good guesses have been made that give us some insight. According to Madathil (2017), Spotify uses collaborative filtering, meaning that the system analyses a user’s music taste in order to conceive the distance between users in this aspect. Then the system will recommend music that a close neighbor listens to. Another strategy the system uses is content based according to Johnson (2014). This strategy relies on personal user data such as age and sex. The recommendations that come out of these systems can be seen in several features of Spotify. It is for example how personalized playlist functions on Spotify are curated. It has been found that Spotify’s recommendation system has led to homogenous consumption (Holtz et al. 2020). We speculate that in order to discover smaller artists on Spotify, one already has to be listening to smaller artists to have them recommended. Meanwhile, the bigger artists that most people are already listening to keep getting recommended in a loop. Essentially, the rich are getting richer.
Algorithmic Culture and the Digital Divide
With the rise of algorithmic recommendation systems, the mediation of cultural consumption has become the norm. Alexander Galloway (2006) describes the marketing of cultural goods through software like recommendation systems as ‘algorithmic culture’. In his work ‘Gaming: Essays on Algorithmic Culture’ Galloway (2006) views software as an allegory for a method of control. Ted Striphas (2015) ascribes the “sorting, classifying and hierarchizing of people, places, objects and ideas” done by computational processes as algorithmic culture. The value of cultural goods is now given by hidden computational processes that are being done by big companies as a result of democratic processes. Democratic processes in this context mean that what is valued is what most people are listening to. Striphas’s view has been adopted within research on music streaming services. According to Morris (2015), recommendation systems give big companies the power to ascribe value to music. Instead of democratic processes, value is ascribed by big companies whose recommendation systems remain black boxes. Some studies show that a relatively large amount of people are unaware of how extensively algorithms dictate their cultural consumption (Eslami et al., 2015; Smith, 2018).
This lack of transparency and power relations related to algorithmic culture underlines the new digital divide among the population. The new digital divide pertains to inequalities among the population that come with the rise of the Internet and other digital technologies (Hargittai, 2003). The concept of the digital divide was first used to explain the imbalance of access to digital technologies and the skills necessary to understand them (Cullen, 2001). This divide creates power imbalances when considering the importance of technology within society. In terms of Spotify’s recommendation system, the lack of transparency causes a divide between its users and the company. It is unclear what music Spotify’s recommendation system ascribes cultural value to, and it is a process the Spotify users have no control over. As we find later in this article, Spotify’s recommendation system creates a divide between bigger and smaller artists in the way it might be ascribing more cultural value to music by bigger artists in its way of hierarchizing artists in their networks.
Because of Spotify’s popularity and its influence on the music industry, it is important to critically evaluate how the platform exerts power, and how it impacts the market. While the platform has democratizing potential, giving unknown and unsigned artists the opportunity to distribute their art, Spotify curates content audiences get to hear, and ultimately what audiences will like: “Digital media is providing cultural content for streaming – for example watching and listening – but discoverability of content is heavily reliant on recommendations and search engines”(Werner 2020).
“Many scholars, belonging to ‘critical algorithm studies’, have argued a need for more insight on algorithms due to their informational and content impact on what people encounter online” (Gran et al., 2021). While we are unable to deconstruct Spotify’s algorithm, using the platform’s API, we can still see and analyze the effects of the recommendation system. This allows us to not only analyze the platform from an academic perspective, looking at the political and economic power of streaming services and the “digital divide” they create but also how it affects user experience, as well as the creation and circulation of content.
Methodology
To analyze Spotify’s “fans also like” recommendation feature, we will utilize the Spotify Artist Network tool by the Digital Methods Initiative (Rieder 2021). The tool makes use of Spotify’s API to create networks of recommended artists. From a queried artist, the tool goes two steps to map out the relations. Although the DMI tool provides the option to visualize the network through sigma.js, we downloaded the network data and utilized Gephi for visualization purposes.
All networks use the Force Atlas 2 layout, a scaling set to 20, and gravity set to 0.5. The node size and color are indicative of the artist’s popularity. The popularity, according to the Spotify API, is a relative value between 1 and 100 that takes into account the popularity of the artist’s music. Therefore, the node sizes range from one to 100, in accordance with the popularity. Similarly, the colors range from light green to deep red. The former indicates relatively low popularity, the latter high popularity. Beyond that, Gephi’s Data Laboratory also gives insight into the artists’ follower counts, as well as the genres within which they are categorized. Similarities herein result in the formation of dense clusters.
We queried ten different artists, five popular artists, and five less popular artists, across different genres, with different amounts of followers, found through Spotify charts, Billboard charts, and playlists aiming to highlight small artists. Subsequently, we analyzed key similarities and differences in the resulting networks.
Analysis
When looking at the related artist’s networks of 5 popular musicians on Spotify, with millions of followers, namely Ariana Grande, Doja Cat, Billie Eilish, Drake, and Taylor Swift, a plethora of similarities can be identified (fig. 1). Generally, the networks consist of 800-900 unique nodes. These nodes form dense clusters around the queried artist. At first glance, as indicated by the color, these networks are made up of popular artists with large followings. This is confirmed by the graph data. Around three to five percent of the artists in these networks have a following smaller than 10.000 people. On the contrary, related to Ariana Grande, we find that about 25% of artists have a following greater than 1 million users.
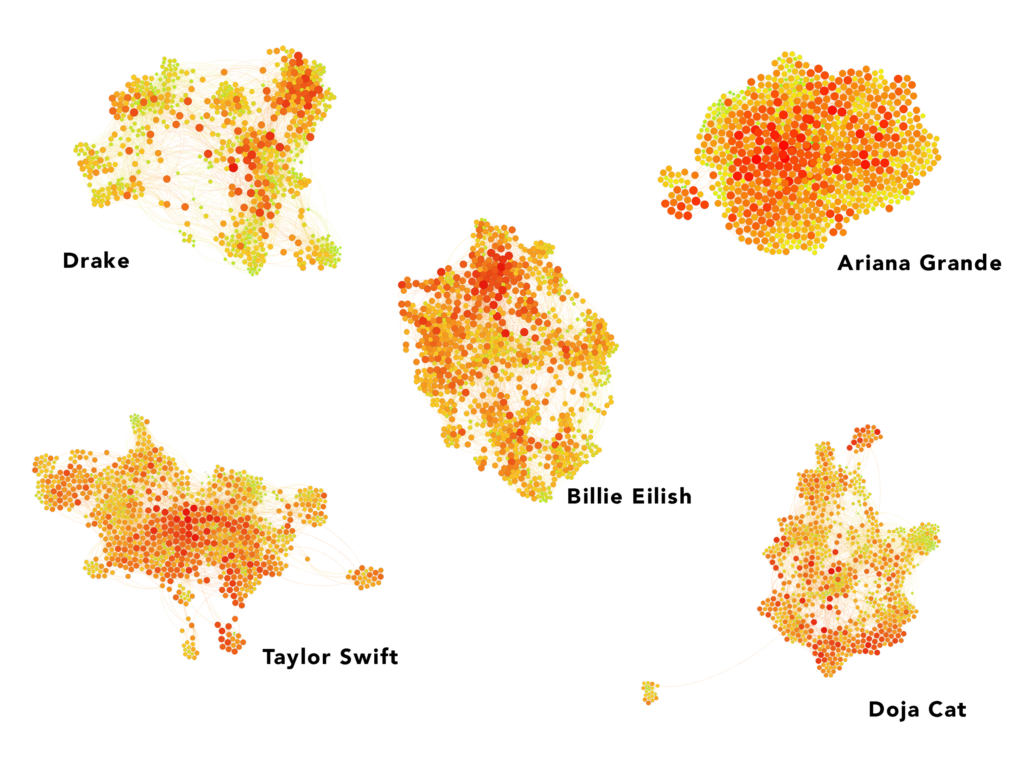
Looking at the genres, the bigger clusters are made up of similar (sub)genres. Especially with the artists that are considered mainstream pop artists, the related artists are faithful to that genre description. With more ambiguous artists, the bigger central clusters seem to be slightly fragmented into these categorizations. As an example, Billie Eilish was described as an “alternative” artist, before migrating towards the pop genre. The upper half of the cluster, therefore, consists of artists using purely “pop” or “dance-pop” as their genres, while the lower half also adds the descriptor “indie” or “indie pop” signifying their independent status.
The networks of these popular artists only show a few outliers. A common cluster that few of these artists share is a cluster of foreign artists, i.e. Spanish-speaking artists. Although these anomalies appear far removed from the center, the small clusters also mainly consist of popular artists. Their position is therefore only a result of the vast difference in genre, as well as the listening habits of users, which indicates the “similarity” of related artists (Spotify AB 2021).
The networks of these popular artists only show a few outliers. A common cluster that few of these artists share is a cluster of foreign artists, i.e. Spanish-speaking artists. Although these anomalies appear far removed from the center, the small clusters also mainly consist of popular artists. Their position is therefore only a result of the vast difference in genre, as well as the listening habits of users, which indicates the “similarity” of related artists (Spotify AB 2021).
When looking at smaller artists (fig. 2), relative to the size of the aforementioned ones, those with followers ranging from less than 1000 to around 200.000, the first thing that becomes apparent is the size of the resulting networks. We looked at the artists Shea Couleé, Badcrop, Slayyyter, Pudge, and Hannah Diamond. Compared to the popular artist, the resulting networks are considerably smaller. They average about 300 unique artists. Looking at artist Shea Couleè’s Spotify page, whose network only has a little over 100 nodes, a short engagement with the recommendation feature shows that it works circularly. As Couleé is a drag queen musician, almost exclusively other drag artists, as well as other queer artists, are recommended. It is difficult, as a user, to go beyond this “drag artists bubble”. Another thing that these networks have in common, is the high concentration of artists with few followers. For artist Pudge, for example, 74.4% of artists in the network have significantly less than 10.000 followers.
Rather than forming big, dense clusters of popular artists, here we can also see more fragmented groupings. Like with popular artists, these are indicative of the artists’ origins, the language they sing in, as well as various sub-genres and artistic movements within certain genres. For Slayyyter, who describes herself with a variety of labels of the pop genre (“hyper-pop”, “dance-pop”, etc.), many seemingly unrelated genres are recommended. These include “strut”, “future bass” “ambient”, and “indie”, among others. Compared to popular artists, recommendations, therefore, are not as faithful to the queried artist’s genre.
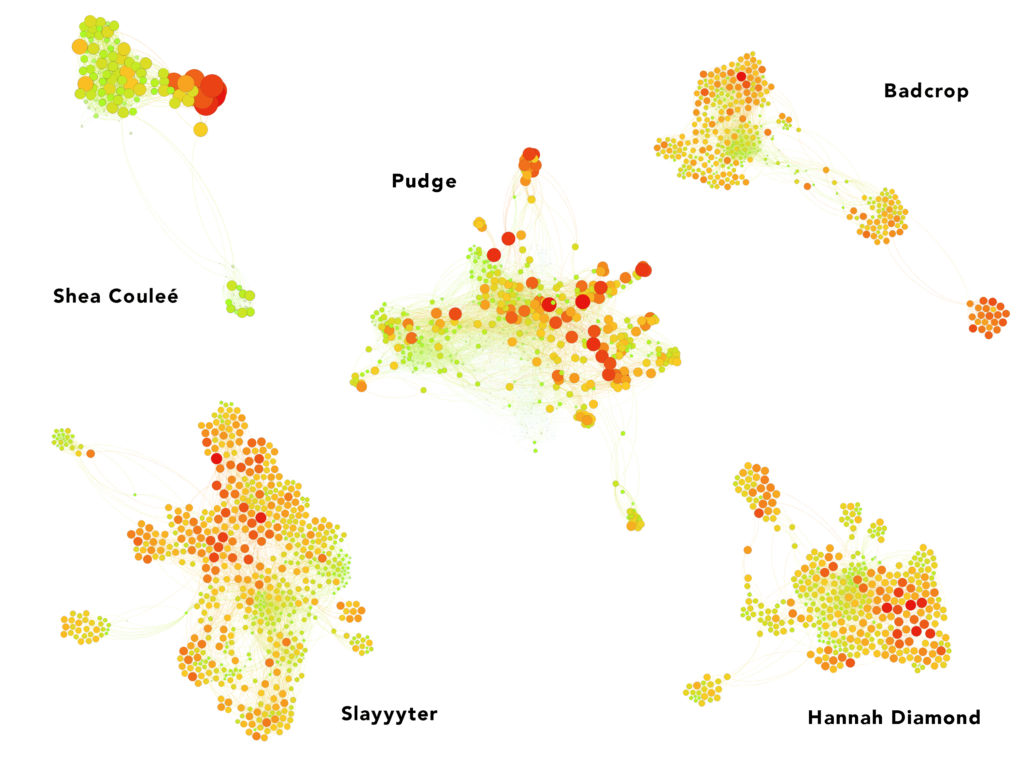
Discussion
The findings confirm that to find new emerging artists, users already have to be immersed in the small artist ecosystem. Another issue that can be identified, is the issue of categorization of artists. On their artist community page, Spotify urges artists to use detailed descriptors to categorize their music. They discourage general terms like “pop” or “rock”, since many artists share this descriptor, making it hard to relate two similar artists (Spotify 2019). The practice of using detailed genre descriptors is twofold. On one hand, popular artists using more general terms creates a closely connected network that is linked to the source. Even those artists who expand upon multiple genres, the recommended artists generally reflect their descriptors, with only little variety. Those artists who use very detailed sub-genres or artistic movements are mostly found at the periphery of those networks or in separate clusters. On the other hand, the use of detailed sub-genres among smaller artists makes it at all possible to be found by users through other artists using that descriptor. However, this also results in a fragmented network, as different artist clusters are sometimes only linked to the queried artist by one or a few shared recommended artists.
The “feedback loop” (Turk 2021) comes into play here as well. As identified, popular artists are more likely to recommend popular artists. Looking at the created networks, many similar artists can be found. Furthermore, some of the artists we queried can be found in the other artists’ networks, despite being categorized differently. Users who listen to these popular artists, therefore do not experience a lot of variety, creating a homogenous listening experience. Similarly, at the example of Shea Couleé, this feedback loop becomes even more apparent. As Spotify categorizes this type of music as “strut” (which seems to be a synonym for “drag queen music”), this creates a loop of almost exclusively other drag artists, despite the music including elements of “house”, “hip hop”, and “rap” music.
Another component of Spotify recommendation is user listening habits. A crucial element of that is playlisting, as a large portion of the music is consumed through playlists. However, proprietary and third-party playlists on Spotify, which are largely curated by editors, and include songs pitched by labels and artists, often include already popular artists. In 2020, the top 1% of Spotify generated around 90% of the streams (Blake 2020). When popular artists appear on similar playlists, they are more likely to be recommended to each other’s audiences. This is reflected by the overlaps in our generated networks, and demonstrates the digital divide (Cullen 2001; Hargittai 2003), as big label support enables artists to efficiently participate in this competitive ecosystem and provides the necessary resources and knowledge to optimize and influence the parameters that are determinant for recommendation.
Because of this, we conceptualized different ways in which the Spotify recommendation system could work. Rather than factors like listening habits, which may be indicative of user interest, but is heavily influenced by playlisting, for example, we tried to use the metadata available on Spotify to find an alternative way, one which may include both big and small artists.
For example, here we focused on personnel and collaborators. Every song on Spotify provides the option for users two view the Song Credits. Under this tab, writers, producers, performers, etc. of a song are credited. Writers or producers who appear frequently in an artist’s discography may have a distinct style that may be reflected in their projects with other artists, background vocalists appearing on songs may be pursuing their musical endeavors. Using these parameters, we may be able to create a recommendation that is more intrinsically linked, rather than through ephemeral bonds that are influenceable. At the example of singer Lana Del Rey, we show how this alternative could look like to Spotify users. We extracted the personnel data of Del Rey’s entire discography. Using a spreadsheet, we identified frequent collaborators and manually researched their other collaborations as well as their own projects. As a result, we conceptualized how the “fans also like” recommendations would change according to this parameter in the following way:
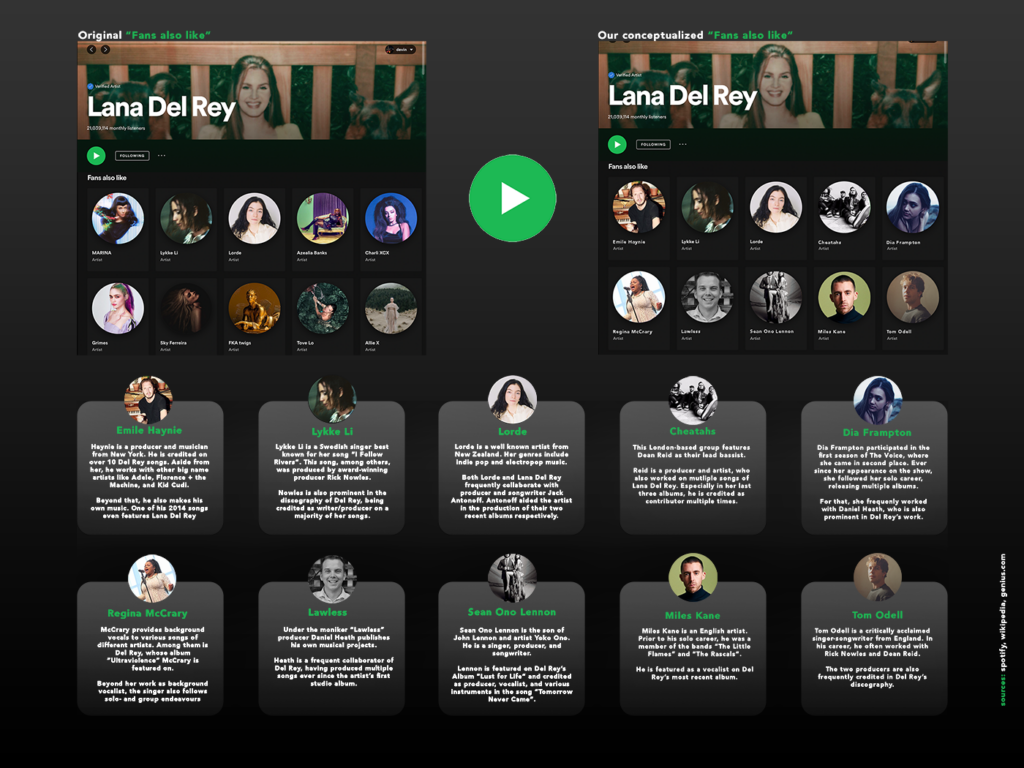
Conclusion
Currently, the recommendation of artists on Spotify is imbalanced. A majority of these are based on categorization/description and listening habits, both of which are easily influenceable. Playlist placement, for example, which considerably influences what music users listen to, is under the influence of big music companies. This makes it impossible for smaller artists to attain the same level of visibility as their more famous counterparts and inhibits users to break out of the “feedback loop” to discover new artists.
Spotify itself is aware of this issue. In May 2021, they launched an initiative to support independent artists and mentor them. Part of this was placing their new music on popular proprietary playlists curated by the platform (Hissong 2021). Certainly, this helped these few artists gain new audiences, however, rather than fixing the recommendation system and its biases, Spotify essentially manufactured their “breakthrough” through the digital divide we still see today.
In our own project, we tried to simulate how an alternative system of recommendation could function, utilizing the available data, as well leaning into Spotify’s collaboration with the music database Genius, which is prominently featured on the platform. Our results show that through our approach, we were able to include a multitude of artists at different fame-levels while staying faithful to the original artist. Furthermore, our findings show that there is an overlap between the Spotify recommended artists, and our curated list, indicating that this practice may very well reflect user listening habits and taste.
Ultimately, while the platform’s efforts with its newly launched initiative are commendable, a revision of the recommendation system is required. Not only in regard to small artists and their efforts to grow audiences, but also for users to be able to experience new music and discover new artists, creating a unique listening experience every time.
References
Bateira, José Lage. 2014. “Spotify-Ed: Music Recommendation and Discovery in Spotify.” Portugal: Universidade do Porto.
Blake, Emily. 2020. “Data Shows 90 Percent of Streams Go to the Top 1 Percent of Artists.” Rolling Stone (blog). September 9, 2020. https://www.rollingstone.com/pro/news/top-1-percent-streaming-1055005/.
Chodos, Asher Tobin. 2019. “What Does Music Mean to Spotify? An Essay on Musical Significance in the Era of Digital Curation.” INSAM I (2): 36–64.
Cullen, Rowena. 2001. “Addressing the Digital Divide.” Online Information Review 25 (5): 311–20. https://doi.org/10.1108/14684520110410517.
Eslami, Motahhare, Aimee Rickman, Kristen Vaccaro, Amirhossein Aleyasen, Andy Vuong, Karrie Karahalios, Kevin Hamilton, and Christian Sandvig. 2015. “‘I Always Assumed That I Wasn’t Really That Close to [Her]’: Reasoning about Invisible Algorithms in News Feeds.” Conference on Human Factors in Computing Systems, CHI ’15, 2015-: 153–62. https://doi.org/10.1145/2702123.2702556.
Galloway, Alexander R. 2006. “Essays on Algorithmic Culture.”
Gran, Anne-Britt, Peter Booth, and Taina Bucher. 2021. “To Be or Not to Be Algorithm Aware: A Question of a New Digital Divide?” Information, Communication & Society 24 (12): 1779–96. https://doi.org/10.1080/1369118X.2020.1736124.
Hargittai, Eszter. 2003. “The DIgital Divide and What To Do About It.” Edited by D.C. Jones. New Economy Handbook, 822–41.
Hissong, Samantha. 2021. “Spotify Is Making a Pledge to Mentor and Market Indie Artists.” Rolling Stone (blog). May 26, 2021. https://www.rollingstone.com/pro/news/spotify-indie-artists-fresh-finds-playlisting-1174065/.
Holtz, David, Benjamin Carterette, Praveen Chandar, Zahra Nazari, Henriette Cramer, and Sinan Aral. 2020. “The Engagement-Diversity Connection: Evidence from a Field Experiment on Spotify.”
Johnson, Christopher C. 2014. “Logistic Matrix Factorization for Implicit Feedback Data.”
Madathil, Mithun. 2017. “Music Recommendation System Spotify – Collaborative Filtering.” Germany: Aachen University.
Morris, Jeremy Wade. 2015. “Curation by Code: Infomediaries and the Data Mining of Taste.” European Journal of Cultural Studies 18 (4–5): 446–63. https://doi.org/10.1177/1367549415577387.
Rieder, Bernhard. 2021. Spotify Artist Network. http://labs.polsys.net/playground/spotify/.
Salganik, Matthew J, Peter Sheridan Dodds, and Duncan J Watts. 2006. “Experimental Study of Inequality and Unpredictability in an Artificial Cultural Market.” Science (American Association for the Advancement of Science)311 (5762): 854–56. https://doi.org/10.1126/science.1121066.
Spotify. 2019. “How ‘Fans Also Like’ Works – Spotify for Artists.” June 21, 2019. https://artists.spotify.com/blog/how-fans-also-like-works, /blog/how-fans-also-like-works.
Spotify AB. 2021. “Web API Reference | Spotify for Developers.” 2021. https://developer.spotify.com/documentation/web-api/reference/.
Turk, Victoria. 2021. “How to Break out of Your Spotify Feedback Loop and Find New Music.” Wired UK, January 13, 2021. https://www.wired.co.uk/article/spotify-feedback-loop-new-music.
Werner, Ann. 2020. “Organizing Music, Organizing Gender: Algorithmic Culture and Spotify Recommendations.” Popular Communication 18 (1): 78–90. https://doi.org/10.1080/15405702.2020.1715980.