Twitter Chatter and Stock Market Trends
Social media as trend indicators
It is not uncommon that when “social media networks” are mentioned, the most common associations among people are with platforms that promote interaction with, and sharing and exchange of user-generated content. With its exponential growth, social media is now regarded as the collective weight and barometer of thoughts and ideas about every aspect of the world – a collective pulse of observation, wisdom and emotional reactions1. The aggregated opinions and feelings of online users are slowly gaining the status of ‘data’ and are altering the ways in which we now arrange and communicate information.
It is no wonder then that this phenomenon has led to a new sort of content analysis, one wherein mining the contents and attributes of social media content allows an exploration of social structure characteristics, analyse action patterns qualitatively and quantitatively, and, as an extension, also make possible the likelihood of successfully indicating and predicting future trends when extracted and analysed “properly”2.
A recent application of social media content for purposes of prediction is seen in the financial sector, where text and sentiment analysis methods are applied to Twitter content to gauge the relationship of tweets to stock returns (even predicting them a day ahead), trading volume and stock price volatility3. There already exist applications and networks, such as HedgeChatter and StockTwits, which offer real-time and automatic data mining to track stock trends. This hyped interest in Twitter content as an indicator of financial market patterns begs one question – is it really so?
Under the scanner
To study the phenomenon first-hand, I narrowed in on seven US volume leader stocks (as per October 11 and 12) – $GOOG (Google Inc.), $MSFT (Microsoft Corporation), $AAPL (Apple Inc.), $BAC (Bank of America Corporation), $JPM (JP Morgan Chase & Co.), $INFY (Infosys Ltd.), $INTC (Inter Corporation) – to chart the stock trends (using Twitter content) and compare it with each stock’s chart on Yahoo! Finance, the go-to “factual” database. US stocks, widely influenced by individuals worldwide, were therefore an appropriate choice of study for this analysis, with a likelihood of a high volume of related Twitter content. If the charts created from the cumulative of Twitter data followed similar a pattern to the Yahoo! charts, or at least gave similar indications, it would prove that Twitter can indeed be regarded as a source for stock market trend indicators.
The process
Using a Twitter scraper, I aggregated all available tweets related to each stock using the search query: “<stock name> stock” (e.g.: GOOG stock). Thereafter, I manually coded the collected tweets for each stock into 3 categories:
P – positive – including tweets that either included ‘buy’/’buying’, directly conveyed an upswing of the stock, or reflected a positive/optimistic attitude towards the stock.
N – negative – including tweets that either included ‘sell’/’selling’, directly conveyed a downswing of the stock, or reflected a negative/pessimistic attitude towards the stock.
NR – not relevant – including tweets that were either unrelated, ambivalent updates, mentioned incidentally in relation to other stocks, or related to other topics.
Once the content for each stock had been coded individually, I created seven line graphs, one for each, charting the difference in the sum of the positive and negative categories for the period of October 4 to October 12, on average. These line graphs where then compared with the standard 5-day trend charts available on Yahoo! Finance for each stock. The Yahoo! Finance charts, however, only included data from October 8 to October 12. Therefore, the comparison in this study was made only with the common data points in both charts.
Here’s what happened:
In a nutshell, it worked.
Due to the differing time periods used in my graphs and the charts on Yahoo! Finance, the trend lines aren’t an exact match, but the visible implied patterns are most often the same. In the $GOOG stock comparison below, both charts depict the high on October 8, the dip on October 9, and the peak on October 11.
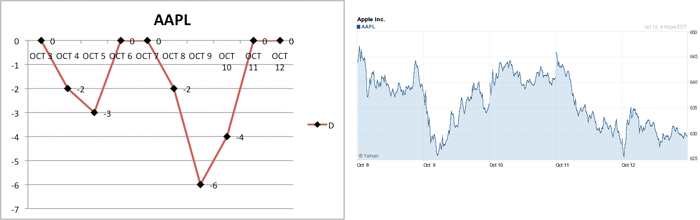
Chart tracing $AAPL stock trend basis Twitter content (created by Audrika Rakshit) + Actual 5-day $AAPL stock chart on Yahoo! Finance
While the $AAPL graphs appear different at a glance (mainly due to different scales of measure), the information it conveys is similar. Looking at both, it’s evident that the stock experienced a sharp dip on October 9 and found its way back up on October 11. Both charts also indicate the dip in Apple’s stock the very next day, on October 12.
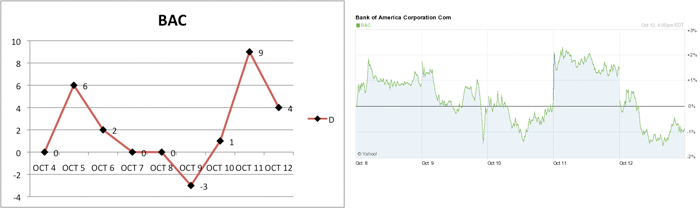
Chart tracing $BAC stock trend basis Twitter content (created by Audrika Rakshit) + Actual 5-day $BAC stock chart on Yahoo! Finance
For the Bank of America ($BAC) stocks, the trend lines differ on the dates on October 8 and October 9 but the Twitter content-based chart does echo the spike in stock action on October 11, as well as the fall the next day.
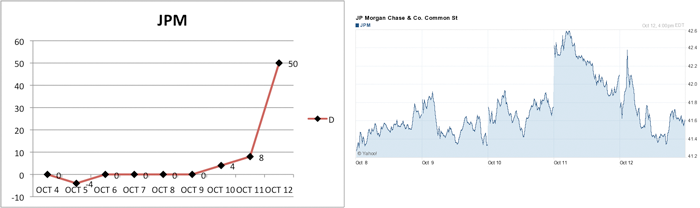
Chart tracing $JPM stock trend basis Twitter content (created by Audrika Rakshit) + Actual 5-day $JPM stock chart on Yahoo! Finance
In the $JPM stock comparison charts, the Twitter deduced chart mirrors the plateau in the early stages and also notes the spike in the stock during October 11 and October 12, in line with the Yahoo! Finance graph.
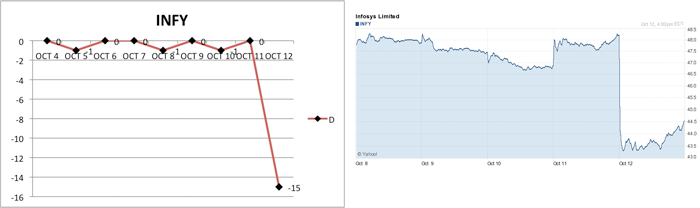
Chart tracing $INFY stock trend basis Twitter content (created by Audrika Rakshit) + Actual 5-day $INFY stock chart on Yahoo! Finance
The Infosys graphs are a clear match, with both charts showing a stagnant performance to begin with, followed by a sharp decline in the stock around October 12.
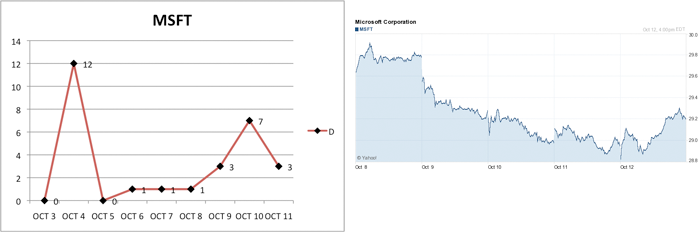
Chart tracing $MSFT stock trend basis Twitter content (created by Audrika Rakshit) + Actual 5-day $MSFT stock chart on Yahoo! FinanceChart tracing $INTC stock trend basis Twitter content (created by Audrika Rakshit) + Actual 5-day $INTC stock chart on Yahoo! Finance
On the other hand, the charts for $MSFT and $INTC are less well-matched. While the Twitter-sourced $MSFT chart does show the slight rise on October 10, it completely misses the spike in the Yahoo! Finance graph on October 8, showing the opposite, in fact – a stagnant dip. The $INTC charts show completely opposite trend lines, mirroring the fact that though there was a lot of Twitter buzz for INTC around October 11 and October 12, it did not necessarily mirror the actual dip in the stock market.
Indicator indeed
Given their propensity for and advantage of real-time data, social media platforms such as Twitter can, in fact, indicate the nature of actual occurrences. Though the stock market landscape is by nature volatile and unpredictable, early indicators extracted from online social media (Twitter feeds, blog, etc.) may enable the prediction of changes in various economic and commercial indicators4. As shown in this analysis, a careful selection of search queries and a clearly coded aggregation of Twitter content can indicate and reflect actual stock market trends, in many instances. To use these findings to further predict market performance is the next step to this analysis.
_________________________________________________
1McGuire, Seth. “Social Media and Markets: The New Frontier.” GNIP. 13 October 2012. <http://gnip.com/success-stories/gnip-smm-nf.pdf>
2Yu, Sheng and Kak, Subhash. “A Survey of Prediction Using Social Media”. 12 October 2012. <http://arxiv.org/pdf/1203.1647.pdf>
3Byrne, Ciara. “Twitter Predicts Performance of Individual Stocks” Venture Beat. Ed. Matt Marshall. 2011. 13 October 2012. <http://venturebeat.com/2011/04/06/twitter-predicts-performance-of-individual-stocks/#cHyJMkOPjb0UROQQ.99>
4Bollen, Johan, Mao, Huina, Zeng, Xiaojun. “Twitter mood predicts the stock market.” Journal of Computational Science. 2.1 (2011): 1-8. 12 October 2012.